mirror of https://github.com/hpcaitech/ColossalAI
[Refactor] remove useless inference code (#5022)
* remove useless code * fix quant model * fix test import bug * mv original inference legacy * fix chatglm2pull/5035/head
parent
81b8f5e76a
commit
c6295c3381
|
@ -9,8 +9,8 @@ from colossalai.pipeline.stage_manager import PipelineStageManager
|
|||
from colossalai.shardformer import ShardConfig, ShardFormer
|
||||
from colossalai.shardformer.policies.base_policy import Policy
|
||||
|
||||
from ..pipeline.microbatch_manager import MicroBatchManager
|
||||
from ..tensor_parallel.kvcache_manager import MemoryManager
|
||||
from ..kvcache_manager import MemoryManager
|
||||
from .microbatch_manager import MicroBatchManager
|
||||
|
||||
PP_AXIS, TP_AXIS = 0, 1
|
||||
|
||||
|
|
|
@ -0,0 +1,248 @@
|
|||
from enum import Enum
|
||||
from typing import Dict
|
||||
|
||||
import torch
|
||||
|
||||
from ..kvcache_manager import BatchInferState, MemoryManager
|
||||
|
||||
__all__ = "MicroBatchManager"
|
||||
|
||||
|
||||
class Status(Enum):
|
||||
PREFILL = 1
|
||||
GENERATE = 2
|
||||
DONE = 3
|
||||
COOLDOWN = 4
|
||||
|
||||
|
||||
class MicroBatchDescription:
|
||||
"""
|
||||
This is the class to record the infomation of each microbatch, and also do some update operation.
|
||||
This clase is the base class of `HeadMicroBatchDescription` and `BodyMicroBatchDescription`, for more
|
||||
details, please refer to the doc of these two classes blow.
|
||||
|
||||
Args:
|
||||
inputs_dict (Dict[str, torch.Tensor]): the inputs of current stage. The key should have `input_ids` and `attention_mask`.
|
||||
output_dict (Dict[str, torch.Tensor]): the outputs of previous stage. The key should have `hidden_states` and `past_key_values`.
|
||||
"""
|
||||
|
||||
def __init__(
|
||||
self,
|
||||
inputs_dict: Dict[str, torch.Tensor],
|
||||
max_input_len: int,
|
||||
max_output_len: int,
|
||||
cache_manager: MemoryManager,
|
||||
) -> None:
|
||||
self.mb_length = inputs_dict["input_ids"].shape[-1]
|
||||
self.target_length = self.mb_length + max_output_len
|
||||
self.infer_state = BatchInferState.init_from_batch(
|
||||
batch=inputs_dict, max_input_len=max_input_len, max_output_len=max_output_len, cache_manager=cache_manager
|
||||
)
|
||||
# print(f"[init] {inputs_dict}, {max_input_len}, {max_output_len}, {cache_manager}, {self.infer_state}")
|
||||
|
||||
def update(self, *args, **kwargs):
|
||||
pass
|
||||
|
||||
@property
|
||||
def state(self):
|
||||
"""
|
||||
Return the state of current micro batch, when current length is equal to target length,
|
||||
the state is DONE, otherwise GENERATE
|
||||
|
||||
"""
|
||||
# TODO: add the condition for early stopping
|
||||
if self.cur_length == self.target_length:
|
||||
return Status.DONE
|
||||
elif self.cur_length == self.target_length - 1:
|
||||
return Status.COOLDOWN
|
||||
else:
|
||||
return Status.GENERATE
|
||||
|
||||
@property
|
||||
def cur_length(self):
|
||||
"""
|
||||
Return the current sequnence length of micro batch
|
||||
|
||||
"""
|
||||
|
||||
|
||||
class HeadMicroBatchDescription(MicroBatchDescription):
|
||||
"""
|
||||
This class is used to record the infomation of the first stage of pipeline, the first stage should have attributes `input_ids` and `attention_mask`
|
||||
and `new_tokens`, and the `new_tokens` is the tokens generated by the first stage. Also due to the schdule of pipeline, the operation to update the
|
||||
information and the condition to determine the state is different from other stages.
|
||||
|
||||
Args:
|
||||
inputs_dict (Dict[str, torch.Tensor]): the inputs of current stage. The key should have `input_ids` and `attention_mask`.
|
||||
output_dict (Dict[str, torch.Tensor]): the outputs of previous stage. The key should have `hidden_states` and `past_key_values`.
|
||||
|
||||
"""
|
||||
|
||||
def __init__(
|
||||
self,
|
||||
inputs_dict: Dict[str, torch.Tensor],
|
||||
max_input_len: int,
|
||||
max_output_len: int,
|
||||
cache_manager: MemoryManager,
|
||||
) -> None:
|
||||
super().__init__(inputs_dict, max_input_len, max_output_len, cache_manager)
|
||||
assert inputs_dict is not None
|
||||
assert inputs_dict.get("input_ids") is not None and inputs_dict.get("attention_mask") is not None
|
||||
self.input_ids = inputs_dict["input_ids"]
|
||||
self.attn_mask = inputs_dict["attention_mask"]
|
||||
self.new_tokens = None
|
||||
|
||||
def update(self, new_token: torch.Tensor = None):
|
||||
if new_token is not None:
|
||||
self._update_newtokens(new_token)
|
||||
if self.state is not Status.DONE and new_token is not None:
|
||||
self._update_attnmask()
|
||||
|
||||
def _update_newtokens(self, new_token: torch.Tensor):
|
||||
if self.new_tokens is None:
|
||||
self.new_tokens = new_token
|
||||
else:
|
||||
self.new_tokens = torch.cat([self.new_tokens, new_token], dim=-1)
|
||||
|
||||
def _update_attnmask(self):
|
||||
self.attn_mask = torch.cat(
|
||||
(self.attn_mask, torch.ones((self.attn_mask.shape[0], 1), dtype=torch.int64, device="cuda")), dim=-1
|
||||
)
|
||||
|
||||
@property
|
||||
def cur_length(self):
|
||||
"""
|
||||
When there is no new_token, the length is mb_length, otherwise the sequence length is `mb_length` plus the length of new_token
|
||||
|
||||
"""
|
||||
if self.new_tokens is None:
|
||||
return self.mb_length
|
||||
else:
|
||||
return self.mb_length + len(self.new_tokens[0])
|
||||
|
||||
|
||||
class BodyMicroBatchDescription(MicroBatchDescription):
|
||||
"""
|
||||
This class is used to record the infomation of the stages except the first stage of pipeline, the stages should have attributes `hidden_states` and `past_key_values`,
|
||||
|
||||
Args:
|
||||
inputs_dict (Dict[str, torch.Tensor]): will always be `None`. Other stages only receive hiddenstates from previous stage.
|
||||
"""
|
||||
|
||||
def __init__(
|
||||
self,
|
||||
inputs_dict: Dict[str, torch.Tensor],
|
||||
max_input_len: int,
|
||||
max_output_len: int,
|
||||
cache_manager: MemoryManager,
|
||||
) -> None:
|
||||
super().__init__(inputs_dict, max_input_len, max_output_len, cache_manager)
|
||||
|
||||
@property
|
||||
def cur_length(self):
|
||||
"""
|
||||
When there is no kv_cache, the length is mb_length, otherwise the sequence length is `kv_cache[0][0].shape[-2]` plus 1
|
||||
|
||||
"""
|
||||
return self.infer_state.seq_len.max().item()
|
||||
|
||||
|
||||
class MicroBatchManager:
|
||||
"""
|
||||
MicroBatchManager is a class that manages the micro batch.
|
||||
|
||||
Args:
|
||||
stage (int): stage id of current stage.
|
||||
micro_batch_size (int): the micro batch size.
|
||||
micro_batch_buffer_size (int): the buffer size for micro batch. Normally, it should be the same as the number of pipeline stages.
|
||||
|
||||
"""
|
||||
|
||||
def __init__(
|
||||
self,
|
||||
stage: int,
|
||||
micro_batch_size: int,
|
||||
micro_batch_buffer_size: int,
|
||||
max_input_len: int,
|
||||
max_output_len: int,
|
||||
cache_manager_list: MemoryManager,
|
||||
):
|
||||
self.stage = stage
|
||||
self.micro_batch_size = micro_batch_size
|
||||
self.buffer_size = micro_batch_buffer_size
|
||||
self.max_input_len = max_input_len
|
||||
self.max_output_len = max_output_len
|
||||
self.cache_manager_list = cache_manager_list
|
||||
self.mb_descrption_buffer = {}
|
||||
self.new_tokens_buffer = {}
|
||||
self.idx = 0
|
||||
|
||||
def add_descrption(self, inputs_dict: Dict[str, torch.Tensor]):
|
||||
if self.stage == 0:
|
||||
self.mb_descrption_buffer[self.idx] = HeadMicroBatchDescription(
|
||||
inputs_dict, self.max_input_len, self.max_output_len, self.cache_manager_list[self.idx]
|
||||
)
|
||||
else:
|
||||
self.mb_descrption_buffer[self.idx] = BodyMicroBatchDescription(
|
||||
inputs_dict, self.max_input_len, self.max_output_len, self.cache_manager_list[self.idx]
|
||||
)
|
||||
|
||||
def step(self, new_token: torch.Tensor = None):
|
||||
"""
|
||||
Update the state if microbatch manager, 2 conditions.
|
||||
1. For first stage in PREFILL, receive inputs and outputs, `_add_descrption` will save its inputs.
|
||||
2. For other conditon, only receive the output of previous stage, and update the descrption.
|
||||
|
||||
Args:
|
||||
inputs_dict (Dict[str, torch.Tensor]): the inputs of current stage. The key should have `input_ids` and `attention_mask`.
|
||||
output_dict (Dict[str, torch.Tensor]): the outputs of previous stage. The key should have `hidden_states` and `past_key_values`.
|
||||
new_token (torch.Tensor): the new token generated by current stage.
|
||||
"""
|
||||
# Add descrption first if the descrption is None
|
||||
self.cur_descrption.update(new_token)
|
||||
return self.cur_state
|
||||
|
||||
def export_new_tokens(self):
|
||||
new_tokens_list = []
|
||||
for i in self.mb_descrption_buffer.values():
|
||||
new_tokens_list.extend(i.new_tokens.tolist())
|
||||
return new_tokens_list
|
||||
|
||||
def is_micro_batch_done(self):
|
||||
if len(self.mb_descrption_buffer) == 0:
|
||||
return False
|
||||
for mb in self.mb_descrption_buffer.values():
|
||||
if mb.state != Status.DONE:
|
||||
return False
|
||||
return True
|
||||
|
||||
def clear(self):
|
||||
self.mb_descrption_buffer.clear()
|
||||
for cache in self.cache_manager_list:
|
||||
cache.free_all()
|
||||
|
||||
def next(self):
|
||||
self.idx = (self.idx + 1) % self.buffer_size
|
||||
|
||||
def _remove_descrption(self):
|
||||
self.mb_descrption_buffer.pop(self.idx)
|
||||
|
||||
@property
|
||||
def cur_descrption(self) -> MicroBatchDescription:
|
||||
return self.mb_descrption_buffer.get(self.idx)
|
||||
|
||||
@property
|
||||
def cur_infer_state(self):
|
||||
if self.cur_descrption is None:
|
||||
return None
|
||||
return self.cur_descrption.infer_state
|
||||
|
||||
@property
|
||||
def cur_state(self):
|
||||
"""
|
||||
Return the state of current micro batch, when current descrption is None, the state is PREFILL
|
||||
|
||||
"""
|
||||
if self.cur_descrption is None:
|
||||
return Status.PREFILL
|
||||
return self.cur_descrption.state
|
|
@ -1,4 +1,5 @@
|
|||
from .bloom import BloomInferenceForwards
|
||||
from .chatglm2 import ChatGLM2InferenceForwards
|
||||
from .llama import LlamaInferenceForwards
|
||||
|
||||
__all__ = ["LlamaInferenceForwards", "BloomInferenceForwards"]
|
||||
__all__ = ["LlamaInferenceForwards", "BloomInferenceForwards", "ChatGLM2InferenceForwards"]
|
||||
|
|
|
@ -14,7 +14,7 @@ from transformers.models.bloom.modeling_bloom import (
|
|||
)
|
||||
from transformers.utils import logging
|
||||
|
||||
from colossalai.inference.tensor_parallel.batch_infer_state import BatchInferState
|
||||
from colossalai.inference.kvcache_manager.batch_infer_state import BatchInferState
|
||||
from colossalai.kernel.triton import bloom_context_attn_fwd, copy_kv_cache_to_dest, token_attention_fwd
|
||||
from colossalai.pipeline.stage_manager import PipelineStageManager
|
||||
|
||||
|
|
|
@ -3,7 +3,7 @@ from typing import List, Optional, Tuple
|
|||
import torch
|
||||
from transformers.utils import logging
|
||||
|
||||
from colossalai.inference.tensor_parallel.batch_infer_state import BatchInferState
|
||||
from colossalai.inference.kvcache_manager import BatchInferState
|
||||
from colossalai.kernel.triton.token_attention_kernel import Llama2TokenAttentionForwards
|
||||
from colossalai.pipeline.stage_manager import PipelineStageManager
|
||||
from colossalai.shardformer import ShardConfig
|
||||
|
|
|
@ -6,7 +6,7 @@ import torch
|
|||
from transformers.models.llama.modeling_llama import LlamaAttention, LlamaDecoderLayer, LlamaForCausalLM, LlamaModel
|
||||
from transformers.utils import logging
|
||||
|
||||
from colossalai.inference.tensor_parallel.batch_infer_state import BatchInferState
|
||||
from colossalai.inference.kvcache_manager.batch_infer_state import BatchInferState
|
||||
from colossalai.kernel.triton import llama_context_attn_fwd, token_attention_fwd
|
||||
from colossalai.kernel.triton.token_attention_kernel import Llama2TokenAttentionForwards
|
||||
from colossalai.pipeline.stage_manager import PipelineStageManager
|
||||
|
|
|
@ -1,5 +1,5 @@
|
|||
from .bloom import BloomModelInferPolicy
|
||||
from .chatglm import ChatGLM2InferPolicy
|
||||
from .chatglm2 import ChatGLM2InferPolicy
|
||||
from .llama import LlamaModelInferPolicy
|
||||
|
||||
__all__ = ["LlamaModelInferPolicy", "BloomModelInferPolicy", "ChatGLM2InferPolicy"]
|
||||
|
|
|
@ -0,0 +1,2 @@
|
|||
from .batch_infer_state import BatchInferState
|
||||
from .kvcache_manager import MemoryManager
|
|
@ -20,8 +20,7 @@ from transformers.modeling_utils import no_init_weights
|
|||
from transformers.utils.generic import ContextManagers
|
||||
from transformers.utils.hub import PushToHubMixin, cached_file
|
||||
|
||||
from colossalai.inference.tensor_parallel.batch_infer_state import BatchInferState
|
||||
from colossalai.inference.tensor_parallel.kvcache_manager import MemoryManager
|
||||
from colossalai.inference.kvcache_manager.batch_infer_state import BatchInferState, MemoryManager
|
||||
|
||||
try:
|
||||
import accelerate
|
||||
|
|
|
@ -21,7 +21,7 @@ from transformers.models.llama.modeling_llama import (
|
|||
)
|
||||
from transformers.utils import add_start_docstrings_to_model_forward
|
||||
|
||||
from colossalai.inference.tensor_parallel.batch_infer_state import BatchInferState
|
||||
from colossalai.inference.kvcache_manager.batch_infer_state import BatchInferState
|
||||
from colossalai.kernel.triton import (
|
||||
copy_kv_cache_to_dest,
|
||||
int8_rotary_embedding_fwd,
|
||||
|
|
|
@ -0,0 +1,143 @@
|
|||
# 🚀 Colossal-Inference
|
||||
|
||||
## Table of contents
|
||||
|
||||
## Introduction
|
||||
|
||||
`Colossal Inference` is a module that contains colossal-ai designed inference framework, featuring high performance, steady and easy usability. `Colossal Inference` incorporated the advantages of the latest open-source inference systems, including LightLLM, TGI, vLLM, FasterTransformer and flash attention. while combining the design of Colossal AI, especially Shardformer, to reduce the learning curve for users.
|
||||
|
||||
## Design
|
||||
|
||||
Colossal Inference is composed of two main components:
|
||||
|
||||
1. High performance kernels and ops: which are inspired from existing libraries and modified correspondingly.
|
||||
2. Efficient memory management mechanism:which includes the key-value cache manager, allowing for zero memory waste during inference.
|
||||
1. `cache manager`: serves as a memory manager to help manage the key-value cache, it integrates functions such as memory allocation, indexing and release.
|
||||
2. `batch_infer_info`: holds all essential elements of a batch inference, which is updated every batch.
|
||||
3. High-level inference engine combined with `Shardformer`: it allows our inference framework to easily invoke and utilize various parallel methods.
|
||||
1. `engine.TPInferEngine`: it is a high level interface that integrates with shardformer, especially for multi-card (tensor parallel) inference:
|
||||
2. `modeling.llama.LlamaInferenceForwards`: contains the `forward` methods for llama inference. (in this case : llama)
|
||||
3. `policies.llama.LlamaModelInferPolicy` : contains the policies for `llama` models, which is used to call `shardformer` and segmentate the model forward in tensor parallelism way.
|
||||
|
||||
## Pipeline of inference:
|
||||
|
||||
In this section we discuss how the colossal inference works and integrates with the `Shardformer` . The details can be found in our codes.
|
||||
|
||||
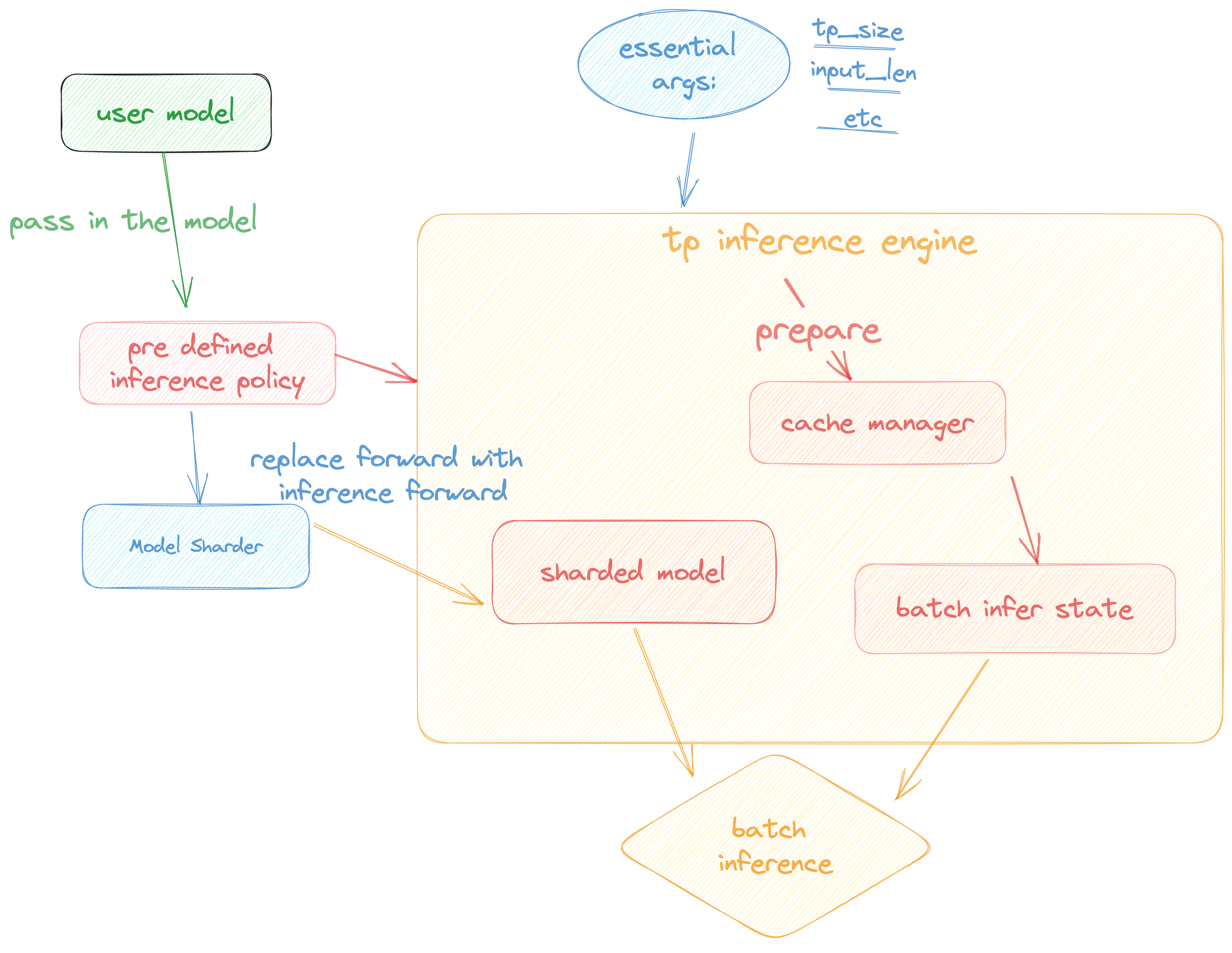
|
||||
|
||||
## Roadmap of our implementation
|
||||
|
||||
- [x] Design cache manager and batch infer state
|
||||
- [x] Design TpInference engine to integrates with `Shardformer`
|
||||
- [x] Register corresponding high-performance `kernel` and `ops`
|
||||
- [x] Design policies and forwards (e.g. `Llama` and `Bloom`)
|
||||
- [x] policy
|
||||
- [x] context forward
|
||||
- [x] token forward
|
||||
- [x] support flash-decoding
|
||||
- [ ] Replace the kernels with `faster-transformer` in token-forward stage
|
||||
- [ ] Support all models
|
||||
- [x] Llama
|
||||
- [x] Llama-2
|
||||
- [x] Bloom
|
||||
- [x] Chatglm2
|
||||
- [ ] Benchmarking for all models
|
||||
|
||||
## Get started
|
||||
|
||||
### Installation
|
||||
|
||||
```bash
|
||||
pip install -e .
|
||||
```
|
||||
|
||||
### Requirements
|
||||
|
||||
dependencies
|
||||
|
||||
```bash
|
||||
pytorch= 1.13.1 (gpu)
|
||||
cuda>= 11.6
|
||||
transformers= 4.30.2
|
||||
triton
|
||||
# for install flash-attention
|
||||
flash-attention
|
||||
|
||||
# install lightllm since we depend on lightllm triton kernels
|
||||
git clone https://github.com/ModelTC/lightllm
|
||||
cd lightllm
|
||||
git checkout 28c1267cfca536b7b4f28e921e03de735b003039
|
||||
pip3 install -e .
|
||||
|
||||
# also, install xformers from source:
|
||||
pip install ninja
|
||||
# Set TORCH_CUDA_ARCH_LIST if running and building on different GPU types
|
||||
pip install -v -U git+https://github.com/facebookresearch/xformers.git@main#egg=xformers
|
||||
|
||||
```
|
||||
|
||||
### Docker
|
||||
|
||||
You can use docker run to use docker container to set-up environment
|
||||
|
||||
```
|
||||
# env: python==3.8, cuda 11.6, pytorch == 1.13.1 triton==2.0.0.dev20221202, vllm kernels support, flash-attention-2 kernels support
|
||||
docker pull hpcaitech/colossalai-inference:v2
|
||||
docker run -it --gpus all --name ANY_NAME -v $PWD:/workspace -w /workspace hpcaitech/colossalai-inference:v2 /bin/bash
|
||||
|
||||
# enter into docker container
|
||||
cd /path/to/CollossalAI
|
||||
pip install -e .
|
||||
|
||||
# install lightllm
|
||||
git clone https://github.com/ModelTC/lightllm
|
||||
cd lightllm
|
||||
git checkout 28c1267cfca536b7b4f28e921e03de735b003039
|
||||
pip3 install -e .
|
||||
|
||||
# install xformers from source
|
||||
pip install ninja
|
||||
# Set TORCH_CUDA_ARCH_LIST if running and building on different GPU types
|
||||
pip install -v -U git+https://github.com/facebookresearch/xformers.git@main#egg=xformers
|
||||
```
|
||||
|
||||
### Dive into fast-inference!
|
||||
|
||||
example files are in
|
||||
|
||||
```bash
|
||||
cd colossalai.examples
|
||||
python xx
|
||||
```
|
||||
|
||||
## Performance
|
||||
|
||||
### environment:
|
||||
|
||||
We conducted multiple benchmark tests to evaluate the performance. We compared the inference `latency` and `throughputs` between `colossal-inference` and original `hugging-face torch fp16`.
|
||||
|
||||
For various models, experiments were conducted using multiple batch sizes under the consistent model configuration of `7 billion(7b)` parameters, `1024` input length, and 128 output length. The obtained results are as follows (due to time constraints, the evaluation has currently been performed solely on the `A100` single GPU performance; multi-GPU performance will be addressed in the future):
|
||||
|
||||
### Single GPU Performance:
|
||||
|
||||
Currently the stats below are calculated based on A100 (single GPU), and we calculate token latency based on average values of context-forward and decoding forward process, which means we combine both of processes to calculate token generation times. We are actively developing new features and methods to further optimize the performance of LLM models. Please stay tuned.
|
||||
|
||||
#### Llama
|
||||
|
||||
| batch_size | 8 | 16 | 32 |
|
||||
| :---------------------: | :----: | :----: | :----: |
|
||||
| hugging-face torch fp16 | 199.12 | 246.56 | 278.4 |
|
||||
| colossal-inference | 326.4 | 582.72 | 816.64 |
|
||||
|
||||

|
||||
|
||||
### Bloom
|
||||
|
||||
| batch_size | 8 | 16 | 32 |
|
||||
| :---------------------: | :----: | :----: | :----: |
|
||||
| hugging-face torch fp16 | 189.68 | 226.66 | 249.61 |
|
||||
| colossal-inference | 323.28 | 538.52 | 611.64 |
|
||||
|
||||

|
||||
|
||||
The results of more models are coming soon!
|
|
@ -0,0 +1,4 @@
|
|||
from .hybridengine import CaiInferEngine
|
||||
from .hybridengine.polices import LlamaModelInferPolicy
|
||||
|
||||
__all__ = ["CaiInferEngine", "LlamaModelInferPolicy"]
|
|
@ -0,0 +1,3 @@
|
|||
from .engine import CaiInferEngine
|
||||
|
||||
__all__ = ["CaiInferEngine"]
|
|
@ -0,0 +1,170 @@
|
|||
import torch
|
||||
import torch.distributed as dist
|
||||
import torch.nn as nn
|
||||
from transformers.tokenization_utils_base import BatchEncoding
|
||||
|
||||
from colossalai.cluster import ProcessGroupMesh
|
||||
from colossalai.pipeline.schedule.generate import GenerateSchedule
|
||||
from colossalai.pipeline.stage_manager import PipelineStageManager
|
||||
from colossalai.shardformer import ShardConfig, ShardFormer
|
||||
from colossalai.shardformer.policies.base_policy import Policy
|
||||
|
||||
from ..pipeline.microbatch_manager import MicroBatchManager
|
||||
from ..tensor_parallel.kvcache_manager import MemoryManager
|
||||
|
||||
PP_AXIS, TP_AXIS = 0, 1
|
||||
|
||||
_supported_models = [
|
||||
"LlamaForCausalLM",
|
||||
]
|
||||
|
||||
|
||||
class CaiInferEngine:
|
||||
"""
|
||||
CaiInferEngine is a class that handles the pipeline parallel inference.
|
||||
|
||||
Args:
|
||||
tp_size (int): the size of tensor parallelism.
|
||||
pp_size (int): the size of pipeline parallelism.
|
||||
model (`nn.Module`): the model not in pipeline style, and will be modified with `ShardFormer`.
|
||||
model_policy (`colossalai.shardformer.policies.base_policy.Policy`): the policy to shardformer model.
|
||||
micro_batch_size (int): the micro batch size.
|
||||
micro_batch_buffer_size (int): the buffer size for micro batch. Normally, it should be the same as the number of pipeline stages.
|
||||
max_batch_size (int): the maximum batch size.
|
||||
max_input_len (int): the maximum input length.
|
||||
max_output_len (int): the maximum output length.
|
||||
|
||||
Example:
|
||||
|
||||
```python
|
||||
from colossalai.inference import InferEngine
|
||||
from colossalai.inference.pipeline.policies import LlamaModelInferPolicy
|
||||
import colossalai
|
||||
from transformers import LlamaForCausalLM, LlamaTokenizer
|
||||
|
||||
colossalai.launch_from_torch(config={})
|
||||
|
||||
model = LlamaForCausalLM.from_pretrained("your_path_to_model")
|
||||
tokenizer = LlamaTokenizer.from_pretrained("/home/lczyh/share/models/llama-7b-hf")
|
||||
# assume the model is infered with 2 pipeline stages
|
||||
inferengine = CaiInferEngine(pp_size=2, model=model, model_policy=LlamaModelInferPolicy())
|
||||
|
||||
input = ["Introduce a landmark in China ","Introduce a landmark in China "]
|
||||
data = tokenizer(input, return_tensors='pt')
|
||||
output = inferengine.inference([data.to('cuda').data])
|
||||
|
||||
```
|
||||
|
||||
"""
|
||||
|
||||
def __init__(
|
||||
self,
|
||||
tp_size: int = 1,
|
||||
pp_size: int = 1,
|
||||
dtype: str = "fp16",
|
||||
model: nn.Module = None,
|
||||
model_policy: Policy = None,
|
||||
micro_batch_size: int = 1,
|
||||
micro_batch_buffer_size: int = None,
|
||||
max_batch_size: int = 4,
|
||||
max_input_len: int = 32,
|
||||
max_output_len: int = 32,
|
||||
verbose: bool = False,
|
||||
# TODO: implement early_stopping, and various gerneration options
|
||||
early_stopping: bool = False,
|
||||
do_sample: bool = False,
|
||||
num_beams: int = 1,
|
||||
) -> None:
|
||||
assert model.__class__.__name__ in _supported_models, f"Model {model.__class__.__name__} is not supported."
|
||||
assert (
|
||||
tp_size * pp_size == dist.get_world_size()
|
||||
), f"TP size({tp_size}) * PP size({pp_size}) should be equal to the global world size ({dist.get_world_size()})"
|
||||
assert model and model_policy, "Model with model_policy should be provided."
|
||||
assert dtype in ["fp16", "fp32", "bf16"], "dtype should be one of 'fp16', 'fp32', 'bf16'"
|
||||
|
||||
assert max_batch_size <= 64, "Max batch size exceeds the constraint"
|
||||
assert max_input_len + max_output_len <= 4096, "Max length exceeds the constraint"
|
||||
|
||||
# TODO: support only tensor parallel inference
|
||||
assert pp_size > 1, "Not support only tensor parallel inference."
|
||||
self.pp_size = pp_size
|
||||
self.tp_size = tp_size
|
||||
|
||||
if dtype == "fp16":
|
||||
self.dtype = torch.float16
|
||||
model.half()
|
||||
elif dtype == "bf16":
|
||||
self.dtype = torch.bfloat16
|
||||
model.to(torch.bfloat16)
|
||||
else:
|
||||
self.dtype = torch.float32
|
||||
|
||||
# Init pg mesh
|
||||
pg_mesh = ProcessGroupMesh(pp_size, tp_size)
|
||||
|
||||
stage_manager = None
|
||||
if pp_size > 1:
|
||||
stage_manager = PipelineStageManager(pg_mesh, PP_AXIS, True)
|
||||
self.cache_manager_list = [
|
||||
self._init_manager(model, max_batch_size, max_input_len, max_output_len)
|
||||
for _ in range(micro_batch_buffer_size or pp_size)
|
||||
]
|
||||
self.mb_manager = MicroBatchManager(
|
||||
stage_manager.stage,
|
||||
micro_batch_size,
|
||||
micro_batch_buffer_size or pp_size,
|
||||
max_input_len,
|
||||
max_output_len,
|
||||
self.cache_manager_list,
|
||||
)
|
||||
self.verbose = verbose
|
||||
self.schedule = GenerateSchedule(stage_manager, self.mb_manager, verbose)
|
||||
|
||||
self.model = self._shardformer(model, model_policy, stage_manager, pg_mesh.get_group_along_axis(TP_AXIS))
|
||||
|
||||
def inference(self, input_list):
|
||||
"""
|
||||
Args:
|
||||
input_list (list): a list of input data, each element is a `BatchEncoding` or `dict`.
|
||||
|
||||
Returns:
|
||||
out (list): a list of output data, each element is a list of token.
|
||||
timestamp (float): the time cost of the inference, only return when verbose is `True`.
|
||||
"""
|
||||
assert isinstance(
|
||||
input_list, (BatchEncoding, dict)
|
||||
), f"Only accept BatchEncoding or dict as input, but get {input_list.__class__.__name__}."
|
||||
if isinstance(input_list, BatchEncoding):
|
||||
input_list = input_list.data
|
||||
out, timestamp = self.schedule.generate_step(self.model, iter([input_list]))
|
||||
if self.verbose:
|
||||
return out, timestamp
|
||||
else:
|
||||
return out
|
||||
|
||||
def _shardformer(self, model, model_policy, stage_manager, tp_group):
|
||||
shardconfig = ShardConfig(
|
||||
tensor_parallel_process_group=tp_group,
|
||||
pipeline_stage_manager=stage_manager,
|
||||
enable_tensor_parallelism=False,
|
||||
enable_fused_normalization=False,
|
||||
enable_all_optimization=False,
|
||||
enable_flash_attention=False,
|
||||
enable_jit_fused=False,
|
||||
enable_sequence_parallelism=False,
|
||||
)
|
||||
shardformer = ShardFormer(shard_config=shardconfig)
|
||||
shard_model, _ = shardformer.optimize(model, model_policy)
|
||||
return shard_model.cuda()
|
||||
|
||||
def _init_manager(self, model, max_batch_size: int, max_input_len: int, max_output_len: int) -> None:
|
||||
max_total_token_num = max_batch_size * (max_input_len + max_output_len)
|
||||
head_dim = model.config.hidden_size // model.config.num_attention_heads
|
||||
head_num = model.config.num_attention_heads
|
||||
num_hidden_layers = (
|
||||
model.config.num_hidden_layers if hasattr(model.config, "num_hidden_layers") else model.config.num_layers
|
||||
)
|
||||
layer_num = num_hidden_layers // self.pp_size
|
||||
|
||||
cache_manager = MemoryManager(max_total_token_num, self.dtype, head_num, head_dim, layer_num)
|
||||
return cache_manager
|
|
@ -0,0 +1,3 @@
|
|||
from .llama import LlamaInferenceForwards
|
||||
|
||||
__all__ = ["LlamaInferenceForwards"]
|
|
@ -0,0 +1,489 @@
|
|||
# This code is adapted from huggingface transformers: https://github.com/huggingface/transformers/blob/v4.34.1/src/transformers/models/llama/modeling_llama.py
|
||||
import math
|
||||
from typing import List, Optional, Tuple
|
||||
|
||||
import torch
|
||||
from transformers.models.llama.modeling_llama import LlamaAttention, LlamaDecoderLayer, LlamaForCausalLM, LlamaModel
|
||||
from transformers.utils import logging
|
||||
|
||||
from colossalai.inference.tensor_parallel.batch_infer_state import BatchInferState
|
||||
from colossalai.kernel.triton import llama_context_attn_fwd, token_attention_fwd
|
||||
from colossalai.kernel.triton.token_attention_kernel import Llama2TokenAttentionForwards
|
||||
from colossalai.pipeline.stage_manager import PipelineStageManager
|
||||
|
||||
from ._utils import copy_kv_to_mem_cache
|
||||
|
||||
try:
|
||||
from lightllm.models.llama2.triton_kernel.context_flashattention_nopad import (
|
||||
context_attention_fwd as lightllm_llama2_context_attention_fwd,
|
||||
)
|
||||
from lightllm.models.llama.triton_kernel.context_flashattention_nopad import (
|
||||
context_attention_fwd as lightllm_context_attention_fwd,
|
||||
)
|
||||
from lightllm.models.llama.triton_kernel.rotary_emb import rotary_emb_fwd as llama_rotary_embedding_fwd
|
||||
|
||||
HAS_LIGHTLLM_KERNEL = True
|
||||
except:
|
||||
print("please install lightllm from source to run inference: https://github.com/ModelTC/lightllm")
|
||||
HAS_LIGHTLLM_KERNEL = False
|
||||
|
||||
try:
|
||||
from flash_attn import flash_attn_with_kvcache
|
||||
|
||||
HAS_FLASH_KERNEL = True
|
||||
except:
|
||||
HAS_FLASH_KERNEL = False
|
||||
print("please install flash attentiom from https://github.com/Dao-AILab/flash-attention")
|
||||
|
||||
|
||||
def rotate_half(x):
|
||||
"""Rotates half the hidden dims of the input."""
|
||||
x1 = x[..., : x.shape[-1] // 2]
|
||||
x2 = x[..., x.shape[-1] // 2 :]
|
||||
return torch.cat((-x2, x1), dim=-1)
|
||||
|
||||
|
||||
def apply_rotary_pos_emb(q, k, cos, sin, position_ids):
|
||||
# The first two dimensions of cos and sin are always 1, so we can `squeeze` them.
|
||||
cos = cos.squeeze(1).squeeze(0) # [seq_len, dim]
|
||||
sin = sin.squeeze(1).squeeze(0) # [seq_len, dim]
|
||||
cos = cos[position_ids].unsqueeze(1) # [bs, 1, seq_len, dim]
|
||||
sin = sin[position_ids].unsqueeze(1) # [bs, 1, seq_len, dim]
|
||||
|
||||
q_embed = (q * cos) + (rotate_half(q) * sin)
|
||||
k_embed = (k * cos) + (rotate_half(k) * sin)
|
||||
return q_embed, k_embed
|
||||
|
||||
|
||||
def llama_triton_context_attention(
|
||||
query_states, key_states, value_states, attn_output, infer_state, num_key_value_groups=1
|
||||
):
|
||||
if num_key_value_groups == 1:
|
||||
if HAS_LIGHTLLM_KERNEL is False:
|
||||
llama_context_attn_fwd(
|
||||
query_states,
|
||||
key_states,
|
||||
value_states,
|
||||
attn_output,
|
||||
infer_state.start_loc,
|
||||
infer_state.seq_len,
|
||||
# infer_state.cache_manager.past_key_values_length,
|
||||
infer_state.max_len_in_batch,
|
||||
)
|
||||
else:
|
||||
lightllm_context_attention_fwd(
|
||||
query_states,
|
||||
key_states,
|
||||
value_states,
|
||||
attn_output,
|
||||
infer_state.start_loc,
|
||||
infer_state.seq_len,
|
||||
# infer_state.cache_manager.past_key_values_length,
|
||||
infer_state.max_len_in_batch,
|
||||
)
|
||||
else:
|
||||
assert HAS_LIGHTLLM_KERNEL is True, "You have to install lightllm kernels to run llama2 model"
|
||||
lightllm_llama2_context_attention_fwd(
|
||||
query_states,
|
||||
key_states,
|
||||
value_states,
|
||||
attn_output,
|
||||
infer_state.start_loc,
|
||||
infer_state.seq_len,
|
||||
# infer_state.cache_manager.past_key_values_length,
|
||||
infer_state.max_len_in_batch,
|
||||
)
|
||||
|
||||
|
||||
def llama_triton_token_attention(query_states, attn_output, infer_state, num_key_value_groups=1):
|
||||
assert HAS_LIGHTLLM_KERNEL is True, "You have to install lightllm kernel to run token attention for llama models"
|
||||
if num_key_value_groups == 1:
|
||||
token_attention_fwd(
|
||||
query_states,
|
||||
infer_state.cache_manager.key_buffer[infer_state.decode_layer_id],
|
||||
infer_state.cache_manager.value_buffer[infer_state.decode_layer_id],
|
||||
attn_output,
|
||||
infer_state.block_loc,
|
||||
infer_state.start_loc,
|
||||
infer_state.seq_len,
|
||||
# infer_state.cache_manager.past_key_values_length,
|
||||
infer_state.max_len_in_batch,
|
||||
)
|
||||
else:
|
||||
Llama2TokenAttentionForwards.token_attn(
|
||||
query_states,
|
||||
infer_state.cache_manager.key_buffer[infer_state.decode_layer_id],
|
||||
infer_state.cache_manager.value_buffer[infer_state.decode_layer_id],
|
||||
attn_output,
|
||||
infer_state.block_loc,
|
||||
infer_state.start_loc,
|
||||
infer_state.seq_len,
|
||||
# infer_state.cache_manager.past_key_values_length,
|
||||
infer_state.max_len_in_batch,
|
||||
infer_state.other_kv_index,
|
||||
)
|
||||
|
||||
|
||||
class LlamaInferenceForwards:
|
||||
"""
|
||||
This class holds forwards for llama inference.
|
||||
We intend to replace the forward methods for LlamaModel, LlamaDecoderLayer, and LlamaAttention for LlamaForCausalLM.
|
||||
"""
|
||||
|
||||
@staticmethod
|
||||
def llama_causal_lm_forward(
|
||||
self: LlamaForCausalLM,
|
||||
input_ids: torch.LongTensor = None,
|
||||
attention_mask: Optional[torch.Tensor] = None,
|
||||
position_ids: Optional[torch.LongTensor] = None,
|
||||
past_key_values: Optional[List[torch.FloatTensor]] = None,
|
||||
inputs_embeds: Optional[torch.FloatTensor] = None,
|
||||
labels: Optional[torch.LongTensor] = None,
|
||||
use_cache: Optional[bool] = None,
|
||||
output_attentions: Optional[bool] = None,
|
||||
output_hidden_states: Optional[bool] = None,
|
||||
return_dict: Optional[bool] = None,
|
||||
infer_state: BatchInferState = None,
|
||||
stage_manager: Optional[PipelineStageManager] = None,
|
||||
hidden_states: Optional[torch.FloatTensor] = None,
|
||||
stage_index: Optional[List[int]] = None,
|
||||
):
|
||||
r"""
|
||||
Args:
|
||||
labels (`torch.LongTensor` of shape `(batch_size, sequence_length)`, *optional*):
|
||||
Labels for computing the masked language modeling loss. Indices should either be in `[0, ...,
|
||||
config.vocab_size]` or -100 (see `input_ids` docstring). Tokens with indices set to `-100` are ignored
|
||||
(masked), the loss is only computed for the tokens with labels in `[0, ..., config.vocab_size]`.
|
||||
|
||||
"""
|
||||
logger = logging.get_logger(__name__)
|
||||
|
||||
return_dict = return_dict if return_dict is not None else self.config.use_return_dict
|
||||
|
||||
if output_attentions:
|
||||
logger.warning_once("output_attentions=True is not supported for pipeline models at the moment.")
|
||||
output_attentions = False
|
||||
if output_hidden_states:
|
||||
logger.warning_once("output_hidden_states=True is not supported for pipeline models at the moment.")
|
||||
output_hidden_states = False
|
||||
|
||||
# If is first stage and after warmup, go throught lm_head first
|
||||
if stage_manager.is_first_stage() and hidden_states is not None:
|
||||
lm_logits = self.lm_head(hidden_states)
|
||||
return {"logits": lm_logits}
|
||||
|
||||
# decoder outputs consists of (dec_features, layer_state, dec_hidden, dec_attn)
|
||||
outputs = LlamaInferenceForwards.llama_model_forward(
|
||||
self.model,
|
||||
input_ids=input_ids,
|
||||
attention_mask=attention_mask,
|
||||
position_ids=position_ids,
|
||||
past_key_values=past_key_values,
|
||||
inputs_embeds=inputs_embeds,
|
||||
use_cache=use_cache,
|
||||
output_attentions=output_attentions,
|
||||
output_hidden_states=output_hidden_states,
|
||||
return_dict=return_dict,
|
||||
infer_state=infer_state,
|
||||
stage_manager=stage_manager,
|
||||
hidden_states=hidden_states,
|
||||
stage_index=stage_index,
|
||||
)
|
||||
|
||||
return outputs
|
||||
|
||||
@staticmethod
|
||||
def llama_model_forward(
|
||||
self: LlamaModel,
|
||||
input_ids: torch.LongTensor = None,
|
||||
attention_mask: Optional[torch.Tensor] = None,
|
||||
position_ids: Optional[torch.LongTensor] = None,
|
||||
past_key_values: Optional[List[torch.FloatTensor]] = None,
|
||||
inputs_embeds: Optional[torch.FloatTensor] = None,
|
||||
use_cache: Optional[bool] = None,
|
||||
output_attentions: Optional[bool] = None,
|
||||
output_hidden_states: Optional[bool] = None,
|
||||
return_dict: Optional[bool] = None,
|
||||
infer_state: BatchInferState = None,
|
||||
stage_manager: Optional[PipelineStageManager] = None,
|
||||
hidden_states: Optional[torch.FloatTensor] = None,
|
||||
stage_index: Optional[List[int]] = None,
|
||||
):
|
||||
return_dict = return_dict if return_dict is not None else self.config.use_return_dict
|
||||
|
||||
use_cache = use_cache if use_cache is not None else self.config.use_cache
|
||||
# retrieve input_ids and inputs_embeds
|
||||
if stage_manager is None or stage_manager.is_first_stage():
|
||||
if input_ids is not None and inputs_embeds is not None:
|
||||
raise ValueError("You cannot specify both decoder_input_ids and decoder_inputs_embeds at the same time")
|
||||
elif input_ids is not None:
|
||||
batch_size, seq_length = input_ids.shape
|
||||
elif inputs_embeds is not None:
|
||||
batch_size, seq_length, _ = inputs_embeds.shape
|
||||
else:
|
||||
raise ValueError("You have to specify either decoder_input_ids or decoder_inputs_embeds")
|
||||
device = input_ids.device if input_ids is not None else inputs_embeds.device
|
||||
if inputs_embeds is None:
|
||||
inputs_embeds = self.embed_tokens(input_ids)
|
||||
hidden_states = inputs_embeds
|
||||
else:
|
||||
assert stage_manager is not None
|
||||
assert hidden_states is not None, f"hidden_state should not be none in stage {stage_manager.stage}"
|
||||
input_shape = hidden_states.shape[:-1]
|
||||
batch_size, seq_length = input_shape
|
||||
device = hidden_states.device
|
||||
|
||||
if infer_state.is_context_stage:
|
||||
past_key_values_length = 0
|
||||
else:
|
||||
past_key_values_length = infer_state.max_len_in_batch - 1
|
||||
|
||||
# NOTE: differentiate with prefill stage
|
||||
# block_loc require different value-assigning method for two different stage
|
||||
if use_cache and seq_length != 1:
|
||||
# NOTE assume prefill stage
|
||||
# allocate memory block
|
||||
infer_state.is_context_stage = True # set prefill stage, notify attention layer
|
||||
infer_state.context_mem_index = infer_state.cache_manager.alloc(infer_state.total_token_num)
|
||||
infer_state.init_block_loc(
|
||||
infer_state.block_loc, infer_state.seq_len, seq_length, infer_state.context_mem_index
|
||||
)
|
||||
else:
|
||||
infer_state.is_context_stage = False
|
||||
alloc_mem = infer_state.cache_manager.alloc_contiguous(batch_size)
|
||||
if alloc_mem is not None:
|
||||
infer_state.decode_is_contiguous = True
|
||||
infer_state.decode_mem_index = alloc_mem[0]
|
||||
infer_state.decode_mem_start = alloc_mem[1]
|
||||
infer_state.decode_mem_end = alloc_mem[2]
|
||||
infer_state.block_loc[:, infer_state.max_len_in_batch - 1] = infer_state.decode_mem_index
|
||||
else:
|
||||
infer_state.decode_is_contiguous = False
|
||||
alloc_mem = infer_state.cache_manager.alloc(batch_size)
|
||||
infer_state.decode_mem_index = alloc_mem
|
||||
infer_state.block_loc[:, infer_state.max_len_in_batch - 1] = infer_state.decode_mem_index
|
||||
|
||||
if position_ids is None:
|
||||
position_ids = torch.arange(
|
||||
past_key_values_length, seq_length + past_key_values_length, dtype=torch.long, device=device
|
||||
)
|
||||
position_ids = position_ids.repeat(batch_size, 1)
|
||||
position_ids = position_ids.unsqueeze(0).view(-1, seq_length)
|
||||
else:
|
||||
position_ids = position_ids.view(-1, seq_length).long()
|
||||
|
||||
if infer_state.is_context_stage:
|
||||
infer_state.position_cos = torch.index_select(self._cos_cached, 0, position_ids.view(-1)).view(
|
||||
position_ids.view(-1).shape[0], -1
|
||||
)
|
||||
infer_state.position_sin = torch.index_select(self._sin_cached, 0, position_ids.view(-1)).view(
|
||||
position_ids.view(-1).shape[0], -1
|
||||
)
|
||||
|
||||
else:
|
||||
seq_len = infer_state.seq_len
|
||||
infer_state.position_cos = torch.index_select(self._cos_cached, 0, seq_len - 1).view(seq_len.shape[0], -1)
|
||||
infer_state.position_sin = torch.index_select(self._sin_cached, 0, seq_len - 1).view(seq_len.shape[0], -1)
|
||||
infer_state.other_kv_index = infer_state.block_loc[0, infer_state.max_len_in_batch - 1].item()
|
||||
|
||||
# embed positions
|
||||
if attention_mask is None:
|
||||
attention_mask = torch.ones(
|
||||
(batch_size, infer_state.max_len_in_batch), dtype=torch.bool, device=hidden_states.device
|
||||
)
|
||||
|
||||
attention_mask = self._prepare_decoder_attention_mask(
|
||||
attention_mask, (batch_size, seq_length), hidden_states, past_key_values_length
|
||||
)
|
||||
|
||||
# decoder layers
|
||||
infer_state.decode_layer_id = 0
|
||||
|
||||
start_idx, end_idx = stage_index[0], stage_index[1]
|
||||
if past_key_values is None:
|
||||
past_key_values = tuple([None] * (end_idx - start_idx + 1))
|
||||
|
||||
for idx, past_key_value in zip(range(start_idx, end_idx), past_key_values):
|
||||
decoder_layer = self.layers[idx]
|
||||
# NOTE: modify here for passing args to decoder layer
|
||||
layer_outputs = decoder_layer(
|
||||
hidden_states,
|
||||
attention_mask=attention_mask,
|
||||
position_ids=position_ids,
|
||||
past_key_value=past_key_value,
|
||||
output_attentions=output_attentions,
|
||||
use_cache=use_cache,
|
||||
infer_state=infer_state,
|
||||
)
|
||||
infer_state.decode_layer_id += 1
|
||||
hidden_states = layer_outputs[0]
|
||||
|
||||
if stage_manager.is_last_stage() or stage_manager.num_stages == 1:
|
||||
hidden_states = self.norm(hidden_states)
|
||||
|
||||
# update indices
|
||||
# infer_state.block_loc[:, infer_state.max_len_in_batch-1] = infer_state.total_token_num + torch.arange(0, batch_size, dtype=torch.int32, device="cuda")
|
||||
infer_state.start_loc += torch.arange(0, batch_size, dtype=torch.int32, device="cuda")
|
||||
infer_state.seq_len += 1
|
||||
infer_state.max_len_in_batch += 1
|
||||
|
||||
# if not return_dict:
|
||||
# return tuple(v for v in [hidden_states, next_cache, all_hidden_states, all_self_attns] if v is not None)
|
||||
|
||||
# return BaseModelOutputWithPast(
|
||||
# last_hidden_state=hidden_states,
|
||||
# past_key_values=next_cache,
|
||||
# hidden_states=all_hidden_states,
|
||||
# attentions=all_self_attns,
|
||||
# )
|
||||
return {"hidden_states": hidden_states}
|
||||
|
||||
@staticmethod
|
||||
def llama_decoder_layer_forward(
|
||||
self: LlamaDecoderLayer,
|
||||
hidden_states: torch.Tensor,
|
||||
attention_mask: Optional[torch.Tensor] = None,
|
||||
position_ids: Optional[torch.LongTensor] = None,
|
||||
past_key_value: Optional[Tuple[torch.Tensor]] = None,
|
||||
output_attentions: Optional[bool] = False,
|
||||
use_cache: Optional[bool] = False,
|
||||
infer_state: Optional[BatchInferState] = None,
|
||||
) -> Tuple[torch.FloatTensor, Optional[Tuple[torch.FloatTensor, torch.FloatTensor]]]:
|
||||
residual = hidden_states
|
||||
|
||||
hidden_states = self.input_layernorm(hidden_states)
|
||||
# Self Attention
|
||||
hidden_states, self_attn_weights, present_key_value = self.self_attn(
|
||||
hidden_states=hidden_states,
|
||||
attention_mask=attention_mask,
|
||||
position_ids=position_ids,
|
||||
past_key_value=past_key_value,
|
||||
output_attentions=output_attentions,
|
||||
use_cache=use_cache,
|
||||
infer_state=infer_state,
|
||||
)
|
||||
|
||||
hidden_states = residual + hidden_states
|
||||
|
||||
# Fully Connected
|
||||
residual = hidden_states
|
||||
hidden_states = self.post_attention_layernorm(hidden_states)
|
||||
hidden_states = self.mlp(hidden_states)
|
||||
hidden_states = residual + hidden_states
|
||||
|
||||
outputs = (hidden_states,)
|
||||
|
||||
if output_attentions:
|
||||
outputs += (self_attn_weights,)
|
||||
|
||||
if use_cache:
|
||||
outputs += (present_key_value,)
|
||||
|
||||
return outputs
|
||||
|
||||
@staticmethod
|
||||
def llama_flash_attn_kvcache_forward(
|
||||
self: LlamaAttention,
|
||||
hidden_states: torch.Tensor,
|
||||
attention_mask: Optional[torch.Tensor] = None,
|
||||
position_ids: Optional[torch.LongTensor] = None,
|
||||
past_key_value: Optional[Tuple[torch.Tensor]] = None,
|
||||
output_attentions: bool = False,
|
||||
use_cache: bool = False,
|
||||
infer_state: Optional[BatchInferState] = None,
|
||||
) -> Tuple[torch.Tensor, Optional[torch.Tensor], Optional[Tuple[torch.Tensor]]]:
|
||||
assert use_cache is True, "use_cache should be set to True using this llama attention"
|
||||
|
||||
bsz, q_len, _ = hidden_states.size()
|
||||
|
||||
# NOTE might think about better way to handle transposed k and v
|
||||
# key_states [bs, seq_len, num_heads, head_dim/embed_size_per_head]
|
||||
# key_states_transposed [bs, num_heads, seq_len, head_dim/embed_size_per_head]
|
||||
|
||||
query_states = self.q_proj(hidden_states).view(bsz, q_len, self.num_heads, self.head_dim)
|
||||
key_states = self.k_proj(hidden_states).view(bsz, q_len, self.num_key_value_heads, self.head_dim)
|
||||
value_states = self.v_proj(hidden_states).view(bsz, q_len, self.num_key_value_heads, self.head_dim)
|
||||
|
||||
# NOTE might want to revise
|
||||
# need some way to record the length of past key values cache
|
||||
# since we won't return past_key_value_cache right now
|
||||
|
||||
cos, sin = infer_state.position_cos, infer_state.position_sin
|
||||
|
||||
llama_rotary_embedding_fwd(query_states.view(-1, self.num_heads, self.head_dim), cos, sin)
|
||||
llama_rotary_embedding_fwd(key_states.view(-1, self.num_key_value_heads, self.head_dim), cos, sin)
|
||||
|
||||
query_states = query_states.reshape(-1, self.num_heads, self.head_dim)
|
||||
key_states = key_states.reshape(-1, self.num_key_value_heads, self.head_dim)
|
||||
value_states = value_states.reshape(-1, self.num_key_value_heads, self.head_dim)
|
||||
|
||||
if infer_state.is_context_stage:
|
||||
# first token generation
|
||||
# copy key and value calculated in current step to memory manager
|
||||
copy_kv_to_mem_cache(
|
||||
infer_state.decode_layer_id,
|
||||
key_states,
|
||||
value_states,
|
||||
infer_state.context_mem_index,
|
||||
infer_state.cache_manager,
|
||||
)
|
||||
attn_output = torch.empty_like(query_states)
|
||||
|
||||
llama_triton_context_attention(
|
||||
query_states,
|
||||
key_states,
|
||||
value_states,
|
||||
attn_output,
|
||||
infer_state,
|
||||
num_key_value_groups=self.num_key_value_groups,
|
||||
)
|
||||
else:
|
||||
if infer_state.decode_is_contiguous:
|
||||
# if decode is contiguous, then we copy to key cache and value cache in cache manager directly
|
||||
cache_k = infer_state.cache_manager.key_buffer[infer_state.decode_layer_id][
|
||||
infer_state.decode_mem_start : infer_state.decode_mem_end, :, :
|
||||
]
|
||||
cache_v = infer_state.cache_manager.value_buffer[infer_state.decode_layer_id][
|
||||
infer_state.decode_mem_start : infer_state.decode_mem_end, :, :
|
||||
]
|
||||
cache_k.copy_(key_states)
|
||||
cache_v.copy_(value_states)
|
||||
else:
|
||||
# if decode is not contiguous, use triton kernel to copy key and value cache
|
||||
# k, v shape: [batch_size, num_heads, head_dim/embed_size_per_head
|
||||
copy_kv_to_mem_cache(
|
||||
infer_state.decode_layer_id,
|
||||
key_states,
|
||||
value_states,
|
||||
infer_state.decode_mem_index,
|
||||
infer_state.cache_manager,
|
||||
)
|
||||
|
||||
if HAS_LIGHTLLM_KERNEL:
|
||||
attn_output = torch.empty_like(query_states)
|
||||
llama_triton_token_attention(
|
||||
query_states, attn_output, infer_state, num_key_value_groups=self.num_key_value_groups
|
||||
)
|
||||
else:
|
||||
self.num_heads // self.num_key_value_heads
|
||||
cache_k = infer_state.cache_manager.key_buffer[infer_state.decode_layer_id]
|
||||
cache_v = infer_state.cache_manager.value_buffer[infer_state.decode_layer_id]
|
||||
|
||||
query_states = query_states.view(bsz, -1, self.num_heads, self.head_dim)
|
||||
copy_cache_k = cache_k.view(bsz, -1, self.num_key_value_heads, self.head_dim)
|
||||
copy_cache_v = cache_v.view(bsz, -1, self.num_key_value_heads, self.head_dim)
|
||||
|
||||
attn_output = flash_attn_with_kvcache(
|
||||
q=query_states,
|
||||
k_cache=copy_cache_k,
|
||||
v_cache=copy_cache_v,
|
||||
softmax_scale=1 / math.sqrt(self.head_dim),
|
||||
causal=True,
|
||||
)
|
||||
|
||||
attn_output = attn_output.view(bsz, q_len, self.hidden_size)
|
||||
|
||||
attn_output = self.o_proj(attn_output)
|
||||
|
||||
# return past_key_value as None
|
||||
return attn_output, None, None
|
|
@ -0,0 +1,3 @@
|
|||
from .llama import LlamaModelInferPolicy
|
||||
|
||||
__all__ = ["LlamaModelInferPolicy"]
|
|
@ -0,0 +1,142 @@
|
|||
from functools import partial
|
||||
from typing import List
|
||||
|
||||
import torch
|
||||
from torch.nn import Module
|
||||
from transformers.models.llama.modeling_llama import (
|
||||
LlamaAttention,
|
||||
LlamaDecoderLayer,
|
||||
LlamaForCausalLM,
|
||||
LlamaModel,
|
||||
LlamaRMSNorm,
|
||||
)
|
||||
|
||||
from colossalai.shardformer.policies.base_policy import ModulePolicyDescription, SubModuleReplacementDescription
|
||||
|
||||
# import colossalai
|
||||
from colossalai.shardformer.policies.llama import LlamaForCausalLMPolicy
|
||||
|
||||
from ..modeling._utils import init_to_get_rotary
|
||||
from ..modeling.llama import LlamaInferenceForwards
|
||||
|
||||
try:
|
||||
from colossalai.kernel.triton import rmsnorm_forward
|
||||
|
||||
HAS_TRITON_RMSNORM = True
|
||||
except:
|
||||
print("you should install triton from https://github.com/openai/triton")
|
||||
HAS_TRITON_RMSNORM = False
|
||||
|
||||
|
||||
def get_triton_rmsnorm_forward():
|
||||
if HAS_TRITON_RMSNORM:
|
||||
|
||||
def _triton_rmsnorm_forward(self: LlamaRMSNorm, hidden_states: torch.Tensor):
|
||||
return rmsnorm_forward(hidden_states, self.weight.data, self.variance_epsilon)
|
||||
|
||||
return _triton_rmsnorm_forward
|
||||
else:
|
||||
return None
|
||||
|
||||
|
||||
class LlamaModelInferPolicy(LlamaForCausalLMPolicy):
|
||||
def __init__(self) -> None:
|
||||
super().__init__()
|
||||
|
||||
def module_policy(self):
|
||||
policy = super().module_policy()
|
||||
|
||||
if self.shard_config.inference_gptq:
|
||||
from colossalai.inference.quant.gptq.cai_gptq import ColCaiQuantLinear, RowCaiQuantLinear
|
||||
|
||||
decoder_attribute_replacement = {
|
||||
"self_attn.hidden_size": self.model.config.hidden_size // self.shard_config.tensor_parallel_size,
|
||||
"self_attn.num_heads": self.model.config.num_attention_heads // self.shard_config.tensor_parallel_size,
|
||||
}
|
||||
policy[LlamaDecoderLayer] = ModulePolicyDescription(
|
||||
attribute_replacement=decoder_attribute_replacement,
|
||||
sub_module_replacement=[
|
||||
SubModuleReplacementDescription(
|
||||
suffix="self_attn.q_proj",
|
||||
target_module=ColCaiQuantLinear,
|
||||
kwargs={"split_num": 1},
|
||||
),
|
||||
SubModuleReplacementDescription(
|
||||
suffix="self_attn.k_proj",
|
||||
target_module=ColCaiQuantLinear,
|
||||
kwargs={"split_num": 1},
|
||||
),
|
||||
SubModuleReplacementDescription(
|
||||
suffix="self_attn.v_proj",
|
||||
target_module=ColCaiQuantLinear,
|
||||
kwargs={"split_num": 1},
|
||||
),
|
||||
SubModuleReplacementDescription(
|
||||
suffix="self_attn.o_proj",
|
||||
target_module=RowCaiQuantLinear,
|
||||
kwargs={"split_num": 1},
|
||||
),
|
||||
SubModuleReplacementDescription(
|
||||
suffix="mlp.gate_proj",
|
||||
target_module=ColCaiQuantLinear,
|
||||
kwargs={"split_num": 1},
|
||||
),
|
||||
SubModuleReplacementDescription(
|
||||
suffix="mlp.up_proj",
|
||||
target_module=ColCaiQuantLinear,
|
||||
kwargs={"split_num": 1},
|
||||
),
|
||||
SubModuleReplacementDescription(
|
||||
suffix="mlp.down_proj",
|
||||
target_module=RowCaiQuantLinear,
|
||||
kwargs={"split_num": 1},
|
||||
),
|
||||
],
|
||||
)
|
||||
|
||||
self.shard_config._infer()
|
||||
|
||||
infer_forward = LlamaInferenceForwards.llama_model_forward
|
||||
method_replacement = {"forward": partial(infer_forward)}
|
||||
self.append_or_create_method_replacement(description=method_replacement, policy=policy, target_key=LlamaModel)
|
||||
|
||||
infer_forward = LlamaInferenceForwards.llama_decoder_layer_forward
|
||||
method_replacement = {"forward": partial(infer_forward)}
|
||||
self.append_or_create_method_replacement(
|
||||
description=method_replacement, policy=policy, target_key=LlamaDecoderLayer
|
||||
)
|
||||
|
||||
infer_forward = LlamaInferenceForwards.llama_flash_attn_kvcache_forward
|
||||
method_replacement = {"forward": partial(infer_forward)}
|
||||
self.append_or_create_method_replacement(
|
||||
description=method_replacement, policy=policy, target_key=LlamaAttention
|
||||
)
|
||||
|
||||
if self.pipeline_stage_manager:
|
||||
# set None as default
|
||||
self.set_pipeline_forward(
|
||||
model_cls=LlamaForCausalLM, new_forward=LlamaInferenceForwards.llama_causal_lm_forward, policy=policy
|
||||
)
|
||||
infer_forward = None
|
||||
if HAS_TRITON_RMSNORM:
|
||||
infer_forward = get_triton_rmsnorm_forward()
|
||||
|
||||
if infer_forward is not None:
|
||||
method_replacement = {"forward": partial(infer_forward)}
|
||||
self.append_or_create_method_replacement(
|
||||
description=method_replacement, policy=policy, target_key=LlamaRMSNorm
|
||||
)
|
||||
|
||||
return policy
|
||||
|
||||
def postprocess(self):
|
||||
init_to_get_rotary(self.model.model)
|
||||
return self.model
|
||||
|
||||
def get_held_layers(self) -> List[Module]:
|
||||
"""Get pipeline layers for current stage."""
|
||||
stage_manager = self.pipeline_stage_manager
|
||||
held_layers = super().get_held_layers()
|
||||
if stage_manager.is_first_stage():
|
||||
held_layers.append(self.model.lm_head)
|
||||
return held_layers
|
|
@ -0,0 +1,4 @@
|
|||
from .cai_gptq import HAS_AUTO_GPTQ
|
||||
|
||||
if HAS_AUTO_GPTQ:
|
||||
from .cai_gptq import CaiGPTQLinearOp, CaiQuantLinear
|
|
@ -0,0 +1,14 @@
|
|||
import warnings
|
||||
|
||||
HAS_AUTO_GPTQ = False
|
||||
try:
|
||||
import auto_gptq
|
||||
|
||||
HAS_AUTO_GPTQ = True
|
||||
except ImportError:
|
||||
warnings.warn("please install auto-gptq from https://github.com/PanQiWei/AutoGPTQ")
|
||||
HAS_AUTO_GPTQ = False
|
||||
|
||||
if HAS_AUTO_GPTQ:
|
||||
from .cai_quant_linear import CaiQuantLinear, ColCaiQuantLinear, RowCaiQuantLinear
|
||||
from .gptq_op import CaiGPTQLinearOp
|
|
@ -0,0 +1,354 @@
|
|||
# Adapted from AutoGPTQ auto_gptq: https://github.com/PanQiWei/AutoGPTQ
|
||||
|
||||
import math
|
||||
import warnings
|
||||
from typing import List, Union
|
||||
|
||||
import numpy as np
|
||||
import torch
|
||||
import torch.distributed as dist
|
||||
import torch.nn as nn
|
||||
from torch.distributed import ProcessGroup
|
||||
|
||||
from colossalai.lazy import LazyInitContext
|
||||
from colossalai.shardformer.layer import ParallelModule
|
||||
|
||||
from .gptq_op import CaiGPTQLinearOp
|
||||
|
||||
HAS_GPTQ_CUDA = False
|
||||
try:
|
||||
from colossalai.kernel.op_builder.gptq import GPTQBuilder
|
||||
|
||||
gptq_cuda = GPTQBuilder().load()
|
||||
HAS_GPTQ_CUDA = True
|
||||
except ImportError:
|
||||
warnings.warn("CUDA gptq is not installed")
|
||||
HAS_GPTQ_CUDA = False
|
||||
|
||||
|
||||
class CaiQuantLinear(nn.Module):
|
||||
def __init__(self, bits, groupsize, infeatures, outfeatures, bias, tp_size=1, tp_rank=0, row_split=False):
|
||||
super().__init__()
|
||||
if bits not in [2, 4, 8]:
|
||||
raise NotImplementedError("Only 2,4,8 bits are supported.")
|
||||
self.infeatures = infeatures
|
||||
self.outfeatures = outfeatures
|
||||
self.bits = bits
|
||||
self.maxq = 2**self.bits - 1
|
||||
self.groupsize = groupsize if groupsize != -1 else infeatures
|
||||
|
||||
self.register_buffer("qweight", torch.zeros((infeatures // 32 * self.bits, outfeatures), dtype=torch.int32))
|
||||
self.register_buffer(
|
||||
"qzeros",
|
||||
torch.zeros((math.ceil(infeatures / self.groupsize), outfeatures // 32 * self.bits), dtype=torch.int32),
|
||||
)
|
||||
self.register_buffer(
|
||||
"scales", torch.zeros((math.ceil(infeatures / self.groupsize), outfeatures), dtype=torch.float16)
|
||||
)
|
||||
if row_split:
|
||||
self.register_buffer(
|
||||
"g_idx",
|
||||
torch.tensor(
|
||||
[(i + (tp_rank * self.infeatures)) // self.groupsize for i in range(infeatures)], dtype=torch.int32
|
||||
),
|
||||
)
|
||||
else:
|
||||
self.register_buffer(
|
||||
"g_idx", torch.tensor([i // self.groupsize for i in range(infeatures)], dtype=torch.int32)
|
||||
)
|
||||
|
||||
if bias:
|
||||
self.register_buffer("bias", torch.zeros((outfeatures), dtype=torch.float16))
|
||||
else:
|
||||
self.bias = None
|
||||
|
||||
self.gptq_linear = CaiGPTQLinearOp(groupsize, bits)
|
||||
|
||||
self.q4 = None
|
||||
self.empty_tensor = torch.empty((1, 1), device="meta")
|
||||
self.tp_size = tp_size
|
||||
self.tp_rank = tp_rank
|
||||
self.row_split = row_split
|
||||
|
||||
def pack(self, linear, scales, zeros, g_idx=None):
|
||||
g_idx = (
|
||||
g_idx.clone()
|
||||
if g_idx is not None
|
||||
else torch.tensor([i // self.groupsize for i in range(self.infeatures)], dtype=torch.int32)
|
||||
)
|
||||
|
||||
scales = scales.t().contiguous()
|
||||
zeros = zeros.t().contiguous()
|
||||
scale_zeros = zeros * scales
|
||||
half_scales = scales.clone().half()
|
||||
# print("scale shape ", scales.shape, scale_zeros.shape, linear.weight.shape)
|
||||
self.scales = scales.clone().half()
|
||||
if linear.bias is not None:
|
||||
self.bias = linear.bias.clone().half()
|
||||
|
||||
pbits = 32
|
||||
ptype = torch.int32
|
||||
unsign_type = np.uint32
|
||||
sign_type = np.int32
|
||||
|
||||
intweight = []
|
||||
for idx in range(self.infeatures):
|
||||
intweight.append(
|
||||
torch.round((linear.weight.data[:, idx] + scale_zeros[g_idx[idx]]) / half_scales[g_idx[idx]]).to(ptype)[
|
||||
:, None
|
||||
]
|
||||
)
|
||||
intweight = torch.cat(intweight, dim=1)
|
||||
intweight = intweight.t().contiguous()
|
||||
intweight = intweight.numpy().astype(unsign_type)
|
||||
qweight = np.zeros((intweight.shape[0] // pbits * self.bits, intweight.shape[1]), dtype=unsign_type)
|
||||
|
||||
i = 0
|
||||
row = 0
|
||||
|
||||
while row < qweight.shape[0]:
|
||||
if self.bits in [2, 4, 8]:
|
||||
for j in range(i, i + (pbits // self.bits)):
|
||||
qweight[row] |= intweight[j] << (self.bits * (j - i))
|
||||
i += pbits // self.bits
|
||||
row += 1
|
||||
else:
|
||||
raise NotImplementedError("Only 2,4,8 bits are supported.")
|
||||
qweight = qweight.astype(sign_type)
|
||||
qweight1 = torch.from_numpy(qweight)
|
||||
qweight1 = qweight1.contiguous() # .to("cuda")
|
||||
self.qweight.data.copy_(qweight1)
|
||||
|
||||
qzeros = np.zeros((zeros.shape[0], zeros.shape[1] // pbits * self.bits), dtype=unsign_type)
|
||||
zeros -= 1
|
||||
zeros = zeros.numpy().astype(unsign_type)
|
||||
i = 0
|
||||
col = 0
|
||||
while col < qzeros.shape[1]:
|
||||
if self.bits in [2, 4, 8]:
|
||||
for j in range(i, i + (pbits // self.bits)):
|
||||
qzeros[:, col] |= zeros[:, j] << (self.bits * (j - i))
|
||||
i += pbits // self.bits
|
||||
col += 1
|
||||
else:
|
||||
raise NotImplementedError("Only 2,4,8 bits are supported.")
|
||||
qzeros = qzeros.astype(sign_type)
|
||||
qzeros = torch.from_numpy(qzeros)
|
||||
qzeros = qzeros
|
||||
self.qzeros.data.copy_(qzeros)
|
||||
|
||||
if torch.equal(self.g_idx.to(g_idx.device), g_idx):
|
||||
self.g_idx = None
|
||||
else:
|
||||
self.g_idx = g_idx
|
||||
|
||||
def init_q4(self):
|
||||
assert self.qweight.device.type == "cuda"
|
||||
self.q4_width = self.qweight.shape[1]
|
||||
if self.g_idx is not None:
|
||||
if self.row_split and torch.equal(
|
||||
self.g_idx,
|
||||
torch.tensor(
|
||||
[(i + (self.tp_rank * self.infeatures)) // self.groupsize for i in range(self.infeatures)],
|
||||
dtype=torch.int32,
|
||||
device=self.g_idx.device,
|
||||
),
|
||||
):
|
||||
self.g_idx = None
|
||||
elif torch.equal(
|
||||
self.g_idx,
|
||||
torch.tensor(
|
||||
[i // self.groupsize for i in range(self.infeatures)], dtype=torch.int32, device=self.g_idx.device
|
||||
),
|
||||
):
|
||||
self.g_idx = None
|
||||
|
||||
if self.g_idx is not None:
|
||||
g_idx = self.g_idx.to("cpu")
|
||||
else:
|
||||
g_idx = self.empty_tensor
|
||||
|
||||
self.q4 = gptq_cuda.make_q4(self.qweight, self.qzeros, self.scales, g_idx, torch.cuda.current_device())
|
||||
torch.cuda.synchronize()
|
||||
|
||||
def forward(self, x):
|
||||
outshape = x.shape[:-1] + (self.outfeatures,)
|
||||
|
||||
if HAS_GPTQ_CUDA and self.bits == 4:
|
||||
if self.q4 is None:
|
||||
self.init_q4()
|
||||
|
||||
x = x.view(-1, x.shape[-1])
|
||||
output = torch.empty((x.shape[0], self.outfeatures), dtype=torch.float16, device=x.device)
|
||||
gptq_cuda.q4_matmul(x.half(), self.q4, output)
|
||||
if self.bias is not None and (not self.row_split or self.tp_size == 1):
|
||||
output.add_(self.bias)
|
||||
else:
|
||||
if self.bias is not None and (not self.row_split or self.tp_size == 1):
|
||||
bias = self.bias
|
||||
else:
|
||||
bias = None
|
||||
output = self.gptq_linear(
|
||||
x,
|
||||
self.qweight,
|
||||
self.scales,
|
||||
self.qzeros,
|
||||
g_idx=self.g_idx,
|
||||
bias=bias,
|
||||
)
|
||||
return output.view(outshape)
|
||||
|
||||
|
||||
def split_column_copy(gptq_linear, cai_linear, tp_size=1, tp_rank=0, split_num=1):
|
||||
qweights = gptq_linear.qweight.split(gptq_linear.out_features // split_num, dim=-1)
|
||||
qzeros = gptq_linear.qzeros.split(gptq_linear.out_features // (32 // cai_linear.bits) // split_num, dim=-1)
|
||||
scales = gptq_linear.scales.split(gptq_linear.out_features // split_num, dim=-1)
|
||||
g_idx = gptq_linear.g_idx
|
||||
if gptq_linear.bias is not None:
|
||||
bias = gptq_linear.bias.split(gptq_linear.out_features // split_num, dim=-1)
|
||||
|
||||
cai_split_out_features = cai_linear.outfeatures // split_num
|
||||
zero_split_block = cai_linear.outfeatures // (32 // cai_linear.bits) // split_num
|
||||
|
||||
for i in range(split_num):
|
||||
cai_linear.qweight[:, i * cai_split_out_features : (i + 1) * cai_split_out_features] = qweights[i][
|
||||
:, tp_rank * cai_split_out_features : (tp_rank + 1) * cai_split_out_features
|
||||
]
|
||||
cai_linear.qzeros[:, i * zero_split_block : (i + 1) * zero_split_block] = qzeros[i][
|
||||
:, tp_rank * zero_split_block : (tp_rank + 1) * zero_split_block
|
||||
]
|
||||
cai_linear.scales[:, i * cai_split_out_features : (i + 1) * cai_split_out_features] = scales[i][
|
||||
:, tp_rank * cai_split_out_features : (tp_rank + 1) * cai_split_out_features
|
||||
]
|
||||
if cai_linear.bias is not None:
|
||||
cai_linear.bias[i * cai_split_out_features : (i + 1) * cai_split_out_features] = bias[i][
|
||||
tp_rank * cai_split_out_features : (tp_rank + 1) * cai_split_out_features
|
||||
]
|
||||
|
||||
cai_linear.g_idx.copy_(g_idx)
|
||||
|
||||
|
||||
def split_row_copy(gptq_linear, cai_linear, tp_rank=0, split_num=1):
|
||||
qweights = gptq_linear.qweight.split(gptq_linear.in_features // split_num, dim=0)
|
||||
qzeros = gptq_linear.qzeros.split(gptq_linear.in_features // split_num, dim=0)
|
||||
scales = gptq_linear.scales.split(gptq_linear.in_features // split_num, dim=0)
|
||||
g_idxs = gptq_linear.g_idx.split(gptq_linear.in_features // split_num, dim=0)
|
||||
|
||||
cai_split_in_features = cai_linear.infeatures // (32 // cai_linear.bits) // split_num
|
||||
zero_split_block = cai_linear.infeatures // cai_linear.groupsize // split_num
|
||||
idx_split_features = cai_linear.infeatures // split_num
|
||||
|
||||
for i in range(split_num):
|
||||
cai_linear.qweight[i * cai_split_in_features : (i + 1) * cai_split_in_features, :] = qweights[i][
|
||||
tp_rank * cai_split_in_features : (tp_rank + 1) * cai_split_in_features, :
|
||||
]
|
||||
cai_linear.qzeros[i * zero_split_block : (i + 1) * zero_split_block, :] = qzeros[i][
|
||||
tp_rank * zero_split_block : (tp_rank + 1) * zero_split_block, :
|
||||
]
|
||||
cai_linear.scales[i * zero_split_block : (i + 1) * zero_split_block, :] = scales[i][
|
||||
tp_rank * zero_split_block : (tp_rank + 1) * zero_split_block, :
|
||||
]
|
||||
cai_linear.g_idx[i * idx_split_features : (i + 1) * idx_split_features] = g_idxs[i][
|
||||
tp_rank * idx_split_features : (tp_rank + 1) * idx_split_features
|
||||
]
|
||||
if cai_linear.bias is not None:
|
||||
cai_linear.bias.copy_(gptq_linear.bias)
|
||||
|
||||
|
||||
class RowCaiQuantLinear(CaiQuantLinear, ParallelModule):
|
||||
def __init__(self, bits, groupsize, infeatures, outfeatures, bias, tp_size=1, tp_rank=0, row_split=False):
|
||||
super().__init__(
|
||||
bits, groupsize, infeatures, outfeatures, bias, tp_size=tp_size, tp_rank=tp_rank, row_split=row_split
|
||||
)
|
||||
self.process_group = None
|
||||
|
||||
@staticmethod
|
||||
def from_native_module(
|
||||
module: nn.Module, process_group: Union[ProcessGroup, List[ProcessGroup]], *args, **kwargs
|
||||
) -> ParallelModule:
|
||||
LazyInitContext.materialize(module)
|
||||
# get the attributes
|
||||
in_features = module.in_features
|
||||
|
||||
# ensure only one process group is passed
|
||||
if isinstance(process_group, (list, tuple)):
|
||||
assert len(process_group) == 1, f"Expected only one process group, got {len(process_group)}."
|
||||
process_group = process_group[0]
|
||||
|
||||
tp_size = dist.get_world_size(process_group)
|
||||
tp_rank = dist.get_rank(process_group)
|
||||
|
||||
if in_features < tp_size:
|
||||
return module
|
||||
|
||||
if in_features % tp_size != 0:
|
||||
raise ValueError(
|
||||
f"The size of in_features:{in_features} is not integer multiples of tensor parallel size: {tp_size}!"
|
||||
)
|
||||
linear_1d = RowCaiQuantLinear(
|
||||
module.bits,
|
||||
module.group_size,
|
||||
module.in_features // tp_size,
|
||||
module.out_features,
|
||||
module.bias is not None,
|
||||
tp_size=tp_size,
|
||||
tp_rank=tp_rank,
|
||||
row_split=True,
|
||||
)
|
||||
linear_1d.process_group = process_group
|
||||
|
||||
split_row_copy(module, linear_1d, tp_rank=tp_rank, **kwargs)
|
||||
return linear_1d
|
||||
|
||||
def forward(self, x):
|
||||
output = super().forward(x)
|
||||
if self.tp_size > 1:
|
||||
dist.all_reduce(output, op=dist.ReduceOp.SUM, group=self.process_group)
|
||||
if self.bias is not None:
|
||||
output.add_(self.bias)
|
||||
return output
|
||||
|
||||
|
||||
class ColCaiQuantLinear(CaiQuantLinear, ParallelModule):
|
||||
def __init__(self, bits, groupsize, infeatures, outfeatures, bias, tp_size=1, tp_rank=0, row_split=False):
|
||||
super().__init__(
|
||||
bits, groupsize, infeatures, outfeatures, bias, tp_size=tp_size, tp_rank=tp_rank, row_split=row_split
|
||||
)
|
||||
self.process_group = None
|
||||
|
||||
@staticmethod
|
||||
def from_native_module(
|
||||
module: nn.Module, process_group: Union[ProcessGroup, List[ProcessGroup]], *args, **kwargs
|
||||
) -> ParallelModule:
|
||||
LazyInitContext.materialize(module)
|
||||
# get the attributes
|
||||
in_features = module.in_features
|
||||
|
||||
# ensure only one process group is passed
|
||||
if isinstance(process_group, (list, tuple)):
|
||||
assert len(process_group) == 1, f"Expected only one process group, got {len(process_group)}."
|
||||
process_group = process_group[0]
|
||||
|
||||
tp_size = dist.get_world_size(process_group)
|
||||
tp_rank = dist.get_rank(process_group)
|
||||
|
||||
if in_features < tp_size:
|
||||
return module
|
||||
|
||||
if in_features % tp_size != 0:
|
||||
raise ValueError(
|
||||
f"The size of in_features:{in_features} is not integer multiples of tensor parallel size: {tp_size}!"
|
||||
)
|
||||
linear_1d = ColCaiQuantLinear(
|
||||
module.bits,
|
||||
module.group_size,
|
||||
module.in_features,
|
||||
module.out_features // tp_size,
|
||||
module.bias is not None,
|
||||
tp_size=tp_size,
|
||||
tp_rank=tp_rank,
|
||||
)
|
||||
linear_1d.process_group = process_group
|
||||
|
||||
split_column_copy(module, linear_1d, tp_rank=tp_rank, **kwargs)
|
||||
return linear_1d
|
|
@ -0,0 +1,58 @@
|
|||
import torch
|
||||
|
||||
from colossalai.kernel.triton import gptq_fused_linear_triton
|
||||
|
||||
|
||||
class CaiGPTQLinearOp(torch.nn.Module):
|
||||
def __init__(self, gptq_group_size, gptq_quant_bits):
|
||||
super(CaiGPTQLinearOp, self).__init__()
|
||||
self.group_size = gptq_group_size
|
||||
self.bits = gptq_quant_bits
|
||||
self.maxq = 2**self.bits - 1
|
||||
self.empty_tensor = torch.zeros(4, device=torch.cuda.current_device())
|
||||
|
||||
def forward(
|
||||
self,
|
||||
input: torch.Tensor,
|
||||
weight: torch.Tensor,
|
||||
weight_scales: torch.Tensor,
|
||||
weight_zeros: torch.Tensor,
|
||||
g_idx: torch.Tensor = None,
|
||||
act_type=0,
|
||||
bias: torch.Tensor = None,
|
||||
residual: torch.Tensor = None,
|
||||
qkv_fused=False,
|
||||
):
|
||||
add_bias = True
|
||||
if bias is None:
|
||||
bias = self.empty_tensor
|
||||
add_bias = False
|
||||
|
||||
add_residual = True
|
||||
if residual is None:
|
||||
residual = self.empty_tensor
|
||||
add_residual = False
|
||||
x = input.view(-1, input.shape[-1])
|
||||
|
||||
out = gptq_fused_linear_triton(
|
||||
x,
|
||||
weight,
|
||||
weight_scales,
|
||||
weight_zeros,
|
||||
bias,
|
||||
residual,
|
||||
self.bits,
|
||||
self.maxq,
|
||||
self.group_size,
|
||||
qkv_fused,
|
||||
add_bias,
|
||||
add_residual,
|
||||
act_type=act_type,
|
||||
g_idx=g_idx,
|
||||
)
|
||||
if qkv_fused:
|
||||
out = out.view(3, input.shape[0], input.shape[1], weight.shape[-1])
|
||||
else:
|
||||
out = out.view(input.shape[0], input.shape[1], weight.shape[-1])
|
||||
|
||||
return out
|
|
@ -0,0 +1,12 @@
|
|||
try:
|
||||
import torch_int
|
||||
|
||||
HAS_TORCH_INT = True
|
||||
except ImportError:
|
||||
HAS_TORCH_INT = False
|
||||
raise ImportError(
|
||||
"Not install torch_int. Please install torch_int from https://github.com/Guangxuan-Xiao/torch-int"
|
||||
)
|
||||
|
||||
if HAS_TORCH_INT:
|
||||
from .llama import LLamaSmoothquantAttention, LlamaSmoothquantMLP
|
|
@ -0,0 +1,487 @@
|
|||
# Adapted from AutoGPTQ: https://github.com/PanQiWei/AutoGPTQ
|
||||
# Adapted from smoothquant: https://github.com/mit-han-lab/smoothquant/blob/main/smoothquant/calibration.py
|
||||
# Adapted from smoothquant: https://github.com/mit-han-lab/smoothquant/blob/main/smoothquant/smooth.py
|
||||
|
||||
import os
|
||||
import warnings
|
||||
from abc import abstractmethod
|
||||
from functools import partial
|
||||
from os.path import isdir, isfile, join
|
||||
from typing import Dict, List, Optional, Union
|
||||
|
||||
import accelerate
|
||||
import numpy as np
|
||||
import torch
|
||||
import torch.nn as nn
|
||||
import transformers
|
||||
from safetensors.torch import save_file as safe_save
|
||||
from tqdm import tqdm
|
||||
from transformers import AutoConfig, AutoModelForCausalLM, PreTrainedModel
|
||||
from transformers.modeling_utils import no_init_weights
|
||||
from transformers.utils.generic import ContextManagers
|
||||
from transformers.utils.hub import PushToHubMixin, cached_file
|
||||
|
||||
from colossalai.inference.tensor_parallel.batch_infer_state import BatchInferState
|
||||
from colossalai.inference.tensor_parallel.kvcache_manager import MemoryManager
|
||||
|
||||
SUPPORTED_MODELS = ["llama"]
|
||||
|
||||
|
||||
class BaseSmoothForCausalLM(nn.Module, PushToHubMixin):
|
||||
layer_type: str = None
|
||||
|
||||
def __init__(self, model: PreTrainedModel, quantized: bool = False):
|
||||
super().__init__()
|
||||
|
||||
self.model = model
|
||||
self.model_type = self.model.config.model_type
|
||||
self._quantized = quantized
|
||||
self.config = self.model.config
|
||||
self.cache_manager = None
|
||||
self.max_total_token_num = 0
|
||||
|
||||
@property
|
||||
def quantized(self):
|
||||
return self._quantized
|
||||
|
||||
def init_cache_manager(self, max_total_token_num=2048):
|
||||
if self.config.model_type == "llama":
|
||||
head_num = self.config.num_key_value_heads
|
||||
layer_num = self.config.num_hidden_layers
|
||||
head_dim = self.config.hidden_size // head_num
|
||||
|
||||
self.cache_manager = MemoryManager(max_total_token_num, torch.int8, head_num, head_dim, layer_num)
|
||||
self.max_total_token_num = max_total_token_num
|
||||
|
||||
def init_batch_state(self, max_output_len=256, **kwargs):
|
||||
input_ids = kwargs["input_ids"]
|
||||
batch_size = len(input_ids)
|
||||
|
||||
seq_start_indexes = torch.zeros(batch_size, dtype=torch.int32, device="cuda")
|
||||
seq_lengths = torch.zeros(batch_size, dtype=torch.int32, device="cuda")
|
||||
start_index = 0
|
||||
max_len_in_batch = -1
|
||||
|
||||
for i in range(batch_size):
|
||||
seq_len = len(input_ids[i])
|
||||
seq_lengths[i] = seq_len
|
||||
seq_start_indexes[i] = start_index
|
||||
start_index += seq_len
|
||||
max_len_in_batch = seq_len if seq_len > max_len_in_batch else max_len_in_batch
|
||||
|
||||
if "max_total_token_num" in kwargs.keys():
|
||||
max_total_token_num = kwargs["max_total_token_num"]
|
||||
self.init_cache_manager(max_total_token_num)
|
||||
|
||||
if "max_new_tokens" in kwargs.keys():
|
||||
max_output_len = kwargs["max_new_tokens"]
|
||||
|
||||
if batch_size * (max_len_in_batch + max_output_len) > self.max_total_token_num:
|
||||
max_total_token_num = batch_size * (max_len_in_batch + max_output_len)
|
||||
warnings.warn(f"reset max tokens to {max_total_token_num}")
|
||||
self.init_cache_manager(max_total_token_num)
|
||||
|
||||
block_loc = torch.empty((batch_size, max_len_in_batch + max_output_len), dtype=torch.long, device="cuda")
|
||||
batch_infer_state = BatchInferState(batch_size, max_len_in_batch)
|
||||
batch_infer_state.seq_len = seq_lengths.to("cuda")
|
||||
batch_infer_state.start_loc = seq_start_indexes.to("cuda")
|
||||
batch_infer_state.block_loc = block_loc
|
||||
batch_infer_state.decode_layer_id = 0
|
||||
batch_infer_state.is_context_stage = True
|
||||
batch_infer_state.set_cache_manager(self.cache_manager)
|
||||
batch_infer_state.cache_manager.free_all()
|
||||
return batch_infer_state
|
||||
|
||||
@abstractmethod
|
||||
@torch.inference_mode()
|
||||
def quantize(
|
||||
self,
|
||||
examples: List[Dict[str, Union[List[int], torch.LongTensor]]],
|
||||
):
|
||||
if self.quantized:
|
||||
raise EnvironmentError("can't execute quantize because the model is quantized.")
|
||||
|
||||
def forward(self, *args, **kwargs):
|
||||
return self.model(*args, **kwargs)
|
||||
|
||||
def generate(self, **kwargs):
|
||||
"""shortcut for model.generate"""
|
||||
|
||||
batch_infer_state = self.init_batch_state(**kwargs)
|
||||
if self.config.model_type == "llama":
|
||||
setattr(self.model.model, "infer_state", batch_infer_state)
|
||||
|
||||
with torch.inference_mode():
|
||||
return self.model.generate(**kwargs)
|
||||
|
||||
def prepare_inputs_for_generation(self, *args, **kwargs):
|
||||
"""shortcut for model.prepare_inputs_for_generation"""
|
||||
return self.model.prepare_inputs_for_generation(*args, **kwargs)
|
||||
|
||||
def collect_act_scales(self, model, tokenizer, dataset, device, num_samples=512, seq_len=512):
|
||||
for text in tqdm(dataset):
|
||||
input_ids = tokenizer(text, return_tensors="pt", max_length=seq_len, truncation=True).input_ids.to(device)
|
||||
model(input_ids)
|
||||
|
||||
def collect_act_dict(self, model, tokenizer, dataset, act_dict, device, num_samples=512, seq_len=512):
|
||||
pbar = tqdm(dataset)
|
||||
for text in pbar:
|
||||
input_ids = tokenizer(text, return_tensors="pt", max_length=seq_len, truncation=True).input_ids.to(device)
|
||||
model(input_ids)
|
||||
mean_scale = np.mean([v["input"] for v in act_dict.values()])
|
||||
pbar.set_description(f"Mean input scale: {mean_scale:.2f}")
|
||||
|
||||
# Adatped from https://github.com/mit-han-lab/smoothquant/blob/main/smoothquant/calibration.py
|
||||
def get_act_scales(self, model, tokenizer, dataset, num_samples=512, seq_len=512):
|
||||
model.eval()
|
||||
device = next(model.parameters()).device
|
||||
act_scales = {}
|
||||
|
||||
def stat_tensor(name, tensor):
|
||||
hidden_dim = tensor.shape[-1]
|
||||
tensor = tensor.view(-1, hidden_dim).abs().detach()
|
||||
comming_max = torch.max(tensor, dim=0)[0].float().cpu()
|
||||
if name in act_scales:
|
||||
act_scales[name] = torch.max(act_scales[name], comming_max)
|
||||
else:
|
||||
act_scales[name] = comming_max
|
||||
|
||||
def stat_input_hook(m, x, y, name):
|
||||
if isinstance(x, tuple):
|
||||
x = x[0]
|
||||
stat_tensor(name, x)
|
||||
|
||||
hooks = []
|
||||
for name, m in model.named_modules():
|
||||
if isinstance(m, nn.Linear):
|
||||
hooks.append(m.register_forward_hook(partial(stat_input_hook, name=name)))
|
||||
|
||||
self.collect_act_scales(model, tokenizer, dataset, device, num_samples, seq_len)
|
||||
|
||||
for h in hooks:
|
||||
h.remove()
|
||||
|
||||
return act_scales
|
||||
|
||||
# Adapted from https://github.com/mit-han-lab/smoothquant/blob/main/smoothquant/smooth.py
|
||||
@torch.no_grad()
|
||||
def smooth_ln_fcs(self, ln, fcs, act_scales, alpha=0.5):
|
||||
if not isinstance(fcs, list):
|
||||
fcs = [fcs]
|
||||
for fc in fcs:
|
||||
assert isinstance(fc, nn.Linear)
|
||||
assert ln.weight.numel() == fc.in_features == act_scales.numel()
|
||||
|
||||
device, dtype = fcs[0].weight.device, fcs[0].weight.dtype
|
||||
act_scales = act_scales.to(device=device, dtype=dtype)
|
||||
weight_scales = torch.cat([fc.weight.abs().max(dim=0, keepdim=True)[0] for fc in fcs], dim=0)
|
||||
weight_scales = weight_scales.max(dim=0)[0].clamp(min=1e-5)
|
||||
|
||||
scales = (act_scales.pow(alpha) / weight_scales.pow(1 - alpha)).clamp(min=1e-5).to(device).to(dtype)
|
||||
|
||||
ln.weight.div_(scales)
|
||||
if hasattr(ln, "bias"):
|
||||
ln.bias.div_(scales)
|
||||
|
||||
for fc in fcs:
|
||||
fc.weight.mul_(scales.view(1, -1))
|
||||
|
||||
@classmethod
|
||||
def create_quantized_model(model):
|
||||
raise NotImplementedError("Not implement create_quantized_model method")
|
||||
|
||||
# Adapted from AutoGPTQ: https://github.com/PanQiWei/AutoGPTQ/blob/main/auto_gptq/modeling/_base.py
|
||||
def save_quantized(
|
||||
self,
|
||||
save_dir: str,
|
||||
model_basename: str,
|
||||
use_safetensors: bool = False,
|
||||
safetensors_metadata: Optional[Dict[str, str]] = None,
|
||||
):
|
||||
"""save quantized model and configs to local disk"""
|
||||
os.makedirs(save_dir, exist_ok=True)
|
||||
|
||||
if not self.quantized:
|
||||
raise EnvironmentError("can only save quantized model, please execute .quantize first.")
|
||||
|
||||
self.model.to("cpu")
|
||||
|
||||
model_base_name = model_basename # or f"smooth-"
|
||||
if use_safetensors:
|
||||
model_save_name = model_base_name + ".safetensors"
|
||||
state_dict = self.model.state_dict()
|
||||
state_dict = {k: v.clone().contiguous() for k, v in state_dict.items()}
|
||||
if safetensors_metadata is None:
|
||||
safetensors_metadata = {}
|
||||
elif not isinstance(safetensors_metadata, dict):
|
||||
raise TypeError("safetensors_metadata must be a dictionary.")
|
||||
else:
|
||||
print(f"Received safetensors_metadata: {safetensors_metadata}")
|
||||
new_safetensors_metadata = {}
|
||||
converted_keys = False
|
||||
for key, value in safetensors_metadata.items():
|
||||
if not isinstance(key, str) or not isinstance(value, str):
|
||||
converted_keys = True
|
||||
try:
|
||||
new_key = str(key)
|
||||
new_value = str(value)
|
||||
except Exception as e:
|
||||
raise TypeError(
|
||||
f"safetensors_metadata: both keys and values must be strings and an error occured when trying to convert them: {e}"
|
||||
)
|
||||
if new_key in new_safetensors_metadata:
|
||||
print(
|
||||
f"After converting safetensors_metadata keys to strings, the key '{new_key}' is duplicated. Ensure that all your metadata keys are strings to avoid overwriting."
|
||||
)
|
||||
new_safetensors_metadata[new_key] = new_value
|
||||
safetensors_metadata = new_safetensors_metadata
|
||||
if converted_keys:
|
||||
print(
|
||||
f"One or more safetensors_metadata keys or values had to be converted to str(). Final safetensors_metadata: {safetensors_metadata}"
|
||||
)
|
||||
|
||||
# Format is required to enable Accelerate to load the metadata
|
||||
# otherwise it raises an OSError
|
||||
safetensors_metadata["format"] = "pt"
|
||||
|
||||
safe_save(state_dict, join(save_dir, model_save_name), safetensors_metadata)
|
||||
else:
|
||||
model_save_name = model_base_name + ".bin"
|
||||
torch.save(self.model.state_dict(), join(save_dir, model_save_name))
|
||||
|
||||
self.model.config.save_pretrained(save_dir)
|
||||
|
||||
# Adapted from AutoGPTQ: https://github.com/PanQiWei/AutoGPTQ/blob/main/auto_gptq/modeling/_base.py
|
||||
def save_pretrained(
|
||||
self,
|
||||
save_dir: str,
|
||||
use_safetensors: bool = False,
|
||||
safetensors_metadata: Optional[Dict[str, str]] = None,
|
||||
**kwargs,
|
||||
):
|
||||
"""alias of save_quantized"""
|
||||
warnings.warn("you are using save_pretrained, which will re-direct to save_quantized.")
|
||||
self.save_quantized(save_dir, use_safetensors, safetensors_metadata)
|
||||
|
||||
# Adapted from AutoGPTQ: https://github.com/PanQiWei/AutoGPTQ/blob/main/auto_gptq/modeling/_base.py
|
||||
@classmethod
|
||||
def from_pretrained(
|
||||
cls,
|
||||
pretrained_model_name_or_path: str,
|
||||
max_memory: Optional[dict] = None,
|
||||
trust_remote_code: bool = False,
|
||||
torch_dtype: torch.dtype = torch.float16,
|
||||
**model_init_kwargs,
|
||||
):
|
||||
if not torch.cuda.is_available():
|
||||
raise EnvironmentError("Load pretrained model to do quantization requires CUDA available.")
|
||||
|
||||
def skip(*args, **kwargs):
|
||||
pass
|
||||
|
||||
torch.nn.init.kaiming_uniform_ = skip
|
||||
torch.nn.init.uniform_ = skip
|
||||
torch.nn.init.normal_ = skip
|
||||
|
||||
# Parameters related to loading from Hugging Face Hub
|
||||
cache_dir = model_init_kwargs.pop("cache_dir", None)
|
||||
force_download = model_init_kwargs.pop("force_download", False)
|
||||
resume_download = model_init_kwargs.pop("resume_download", False)
|
||||
proxies = model_init_kwargs.pop("proxies", None)
|
||||
local_files_only = model_init_kwargs.pop("local_files_only", False)
|
||||
use_auth_token = model_init_kwargs.pop("use_auth_token", None)
|
||||
revision = model_init_kwargs.pop("revision", None)
|
||||
subfolder = model_init_kwargs.pop("subfolder", "")
|
||||
model_init_kwargs.pop("_commit_hash", None)
|
||||
|
||||
cached_file_kwargs = {
|
||||
"cache_dir": cache_dir,
|
||||
"force_download": force_download,
|
||||
"proxies": proxies,
|
||||
"resume_download": resume_download,
|
||||
"local_files_only": local_files_only,
|
||||
"use_auth_token": use_auth_token,
|
||||
"revision": revision,
|
||||
"subfolder": subfolder,
|
||||
}
|
||||
|
||||
config = AutoConfig.from_pretrained(pretrained_model_name_or_path, trust_remote_code=True, **cached_file_kwargs)
|
||||
if config.model_type not in SUPPORTED_MODELS:
|
||||
raise TypeError(f"{config.model_type} isn't supported yet.")
|
||||
|
||||
# enforce some values despite user specified
|
||||
model_init_kwargs["torch_dtype"] = torch_dtype
|
||||
model_init_kwargs["trust_remote_code"] = trust_remote_code
|
||||
if max_memory:
|
||||
if "disk" in max_memory:
|
||||
raise NotImplementedError("disk offload not support yet.")
|
||||
with accelerate.init_empty_weights():
|
||||
model = AutoModelForCausalLM.from_config(config, trust_remote_code=True)
|
||||
model.tie_weights()
|
||||
|
||||
max_memory = accelerate.utils.get_balanced_memory(
|
||||
model,
|
||||
max_memory=max_memory,
|
||||
no_split_module_classes=[cls.layer_type],
|
||||
dtype=model_init_kwargs["torch_dtype"],
|
||||
low_zero=False,
|
||||
)
|
||||
model_init_kwargs["device_map"] = accelerate.infer_auto_device_map(
|
||||
model,
|
||||
max_memory=max_memory,
|
||||
no_split_module_classes=[cls.layer_type],
|
||||
dtype=model_init_kwargs["torch_dtype"],
|
||||
)
|
||||
model_init_kwargs["low_cpu_mem_usage"] = True
|
||||
|
||||
del model
|
||||
else:
|
||||
model_init_kwargs["device_map"] = None
|
||||
model_init_kwargs["low_cpu_mem_usage"] = False
|
||||
|
||||
torch.cuda.empty_cache()
|
||||
|
||||
merged_kwargs = {**model_init_kwargs, **cached_file_kwargs}
|
||||
model = AutoModelForCausalLM.from_pretrained(pretrained_model_name_or_path, **merged_kwargs)
|
||||
|
||||
model_config = model.config.to_dict()
|
||||
seq_len_keys = ["max_position_embeddings", "seq_length", "n_positions"]
|
||||
if any([k in model_config for k in seq_len_keys]):
|
||||
for key in seq_len_keys:
|
||||
if key in model_config:
|
||||
model.seqlen = model_config[key]
|
||||
break
|
||||
else:
|
||||
warnings.warn("can't get model's sequence length from model config, will set to 4096.")
|
||||
model.seqlen = 4096
|
||||
model.eval()
|
||||
|
||||
return cls(model, False)
|
||||
|
||||
# Adapted from AutoGPTQ: https://github.com/PanQiWei/AutoGPTQ/blob/main/auto_gptq/modeling/_base.py
|
||||
@classmethod
|
||||
def from_quantized(
|
||||
cls,
|
||||
model_name_or_path: Optional[str],
|
||||
model_basename: Optional[str] = None,
|
||||
device_map: Optional[Union[str, Dict[str, Union[int, str]]]] = None,
|
||||
max_memory: Optional[dict] = None,
|
||||
device: Optional[Union[str, int]] = None,
|
||||
low_cpu_mem_usage: bool = False,
|
||||
torch_dtype: Optional[torch.dtype] = None,
|
||||
use_safetensors: bool = False,
|
||||
trust_remote_code: bool = False,
|
||||
**kwargs,
|
||||
):
|
||||
"""load quantized model from local disk"""
|
||||
|
||||
# Parameters related to loading from Hugging Face Hub
|
||||
cache_dir = kwargs.pop("cache_dir", None)
|
||||
force_download = kwargs.pop("force_download", False)
|
||||
resume_download = kwargs.pop("resume_download", False)
|
||||
proxies = kwargs.pop("proxies", None)
|
||||
local_files_only = kwargs.pop("local_files_only", False)
|
||||
use_auth_token = kwargs.pop("use_auth_token", None)
|
||||
revision = kwargs.pop("revision", None)
|
||||
subfolder = kwargs.pop("subfolder", "")
|
||||
commit_hash = kwargs.pop("_commit_hash", None)
|
||||
|
||||
cached_file_kwargs = {
|
||||
"cache_dir": cache_dir,
|
||||
"force_download": force_download,
|
||||
"proxies": proxies,
|
||||
"resume_download": resume_download,
|
||||
"local_files_only": local_files_only,
|
||||
"use_auth_token": use_auth_token,
|
||||
"revision": revision,
|
||||
"subfolder": subfolder,
|
||||
"_raise_exceptions_for_missing_entries": False,
|
||||
"_commit_hash": commit_hash,
|
||||
}
|
||||
|
||||
# == step1: prepare configs and file names == #
|
||||
config = AutoConfig.from_pretrained(
|
||||
model_name_or_path, trust_remote_code=trust_remote_code, **cached_file_kwargs
|
||||
)
|
||||
|
||||
if config.model_type not in SUPPORTED_MODELS:
|
||||
raise TypeError(f"{config.model_type} isn't supported yet.")
|
||||
|
||||
extensions = []
|
||||
if use_safetensors:
|
||||
extensions.append(".safetensors")
|
||||
else:
|
||||
extensions += [".bin", ".pt"]
|
||||
|
||||
model_name_or_path = str(model_name_or_path)
|
||||
is_local = isdir(model_name_or_path)
|
||||
|
||||
resolved_archive_file = None
|
||||
if is_local:
|
||||
model_save_name = join(model_name_or_path, model_basename)
|
||||
for ext in extensions:
|
||||
if isfile(model_save_name + ext):
|
||||
resolved_archive_file = model_save_name + ext
|
||||
break
|
||||
else: # remote
|
||||
for ext in extensions:
|
||||
resolved_archive_file = cached_file(model_name_or_path, model_basename + ext, **cached_file_kwargs)
|
||||
if resolved_archive_file is not None:
|
||||
break
|
||||
|
||||
if resolved_archive_file is None: # Could not find a model file to use
|
||||
raise FileNotFoundError(f"Could not find model in {model_name_or_path}")
|
||||
|
||||
model_save_name = resolved_archive_file
|
||||
|
||||
# == step2: convert model to quantized-model (replace Linear) == #
|
||||
def skip(*args, **kwargs):
|
||||
pass
|
||||
|
||||
torch.nn.init.kaiming_uniform_ = skip
|
||||
torch.nn.init.uniform_ = skip
|
||||
torch.nn.init.normal_ = skip
|
||||
|
||||
transformers.modeling_utils._init_weights = False
|
||||
|
||||
init_contexts = [no_init_weights()]
|
||||
if low_cpu_mem_usage:
|
||||
init_contexts.append(accelerate.init_empty_weights(include_buffers=True))
|
||||
|
||||
with ContextManagers(init_contexts):
|
||||
model = AutoModelForCausalLM.from_config(
|
||||
config, trust_remote_code=trust_remote_code, torch_dtype=torch_dtype
|
||||
)
|
||||
cls.create_quantized_model(model)
|
||||
model.tie_weights()
|
||||
|
||||
# == step3: load checkpoint to quantized-model == #
|
||||
accelerate.utils.modeling.load_checkpoint_in_model(
|
||||
model, checkpoint=model_save_name, offload_state_dict=True, offload_buffers=True
|
||||
)
|
||||
|
||||
# == step4: set seqlen == #
|
||||
model_config = model.config.to_dict()
|
||||
seq_len_keys = ["max_position_embeddings", "seq_length", "n_positions"]
|
||||
if any([k in model_config for k in seq_len_keys]):
|
||||
for key in seq_len_keys:
|
||||
if key in model_config:
|
||||
model.seqlen = model_config[key]
|
||||
break
|
||||
else:
|
||||
warnings.warn("can't get model's sequence length from model config, will set to 4096.")
|
||||
model.seqlen = 4096
|
||||
|
||||
return cls(
|
||||
model,
|
||||
True,
|
||||
)
|
||||
|
||||
def __getattr__(self, item):
|
||||
try:
|
||||
return super().__getattr__(item)
|
||||
except:
|
||||
return getattr(self.model, item)
|
||||
|
||||
|
||||
__all__ = ["BaseSmoothForCausalLM"]
|
|
@ -0,0 +1,179 @@
|
|||
# modified from torch-int: https://github.com/Guangxuan-Xiao/torch-int/blob/main/torch_int/nn/linear.py
|
||||
|
||||
import torch
|
||||
from torch_int._CUDA import linear_a8_w8_b8_o8, linear_a8_w8_bfp32_ofp32
|
||||
from torch_int.functional.quantization import quantize_per_tensor_absmax
|
||||
|
||||
try:
|
||||
from colossalai.kernel.op_builder.smoothquant import SmoothquantBuilder
|
||||
|
||||
smoothquant_cuda = SmoothquantBuilder().load()
|
||||
HAS_SMOOTHQUANT_CUDA = True
|
||||
except ImportError:
|
||||
HAS_SMOOTHQUANT_CUDA = False
|
||||
raise ImportError("CUDA smoothquant linear is not installed")
|
||||
|
||||
|
||||
class W8A8BFP32O32LinearSiLU(torch.nn.Module):
|
||||
def __init__(self, in_features, out_features, alpha=1.0, beta=1.0):
|
||||
super().__init__()
|
||||
self.in_features = in_features
|
||||
self.out_features = out_features
|
||||
|
||||
self.register_buffer(
|
||||
"weight",
|
||||
torch.randint(
|
||||
-127,
|
||||
127,
|
||||
(self.out_features, self.in_features),
|
||||
dtype=torch.int8,
|
||||
requires_grad=False,
|
||||
),
|
||||
)
|
||||
self.register_buffer(
|
||||
"bias",
|
||||
torch.zeros((1, self.out_features), dtype=torch.float, requires_grad=False),
|
||||
)
|
||||
self.register_buffer("a", torch.tensor(alpha))
|
||||
|
||||
def to(self, *args, **kwargs):
|
||||
super().to(*args, **kwargs)
|
||||
self.weight = self.weight.to(*args, **kwargs)
|
||||
self.bias = self.bias.to(*args, **kwargs)
|
||||
return self
|
||||
|
||||
@torch.no_grad()
|
||||
def forward(self, x):
|
||||
x_shape = x.shape
|
||||
x = x.view(-1, x_shape[-1])
|
||||
y = smoothquant_cuda.linear_silu_a8_w8_bfp32_ofp32(x, self.weight, self.bias, self.a.item(), 1.0)
|
||||
y = y.view(*x_shape[:-1], -1)
|
||||
return y
|
||||
|
||||
@staticmethod
|
||||
def from_float(module: torch.nn.Linear, input_scale):
|
||||
int8_module = W8A8BFP32O32LinearSiLU(module.in_features, module.out_features)
|
||||
int8_weight, weight_scale = quantize_per_tensor_absmax(module.weight)
|
||||
alpha = input_scale * weight_scale
|
||||
int8_module.weight = int8_weight
|
||||
if module.bias is not None:
|
||||
int8_module.bias.data.copy_(module.bias.to(torch.float))
|
||||
int8_module.a = alpha
|
||||
return int8_module
|
||||
|
||||
|
||||
# modified from torch-int: https://github.com/Guangxuan-Xiao/torch-int/blob/main/torch_int/nn/linear.py
|
||||
class W8A8B8O8Linear(torch.nn.Module):
|
||||
# For qkv_proj
|
||||
def __init__(self, in_features, out_features, alpha=1.0, beta=1.0):
|
||||
super().__init__()
|
||||
self.in_features = in_features
|
||||
self.out_features = out_features
|
||||
|
||||
self.register_buffer(
|
||||
"weight",
|
||||
torch.randint(
|
||||
-127,
|
||||
127,
|
||||
(self.out_features, self.in_features),
|
||||
dtype=torch.int8,
|
||||
requires_grad=False,
|
||||
),
|
||||
)
|
||||
self.register_buffer(
|
||||
"bias",
|
||||
torch.zeros((1, self.out_features), dtype=torch.int8, requires_grad=False),
|
||||
)
|
||||
self.register_buffer("a", torch.tensor(alpha))
|
||||
self.register_buffer("b", torch.tensor(beta))
|
||||
|
||||
def to(self, *args, **kwargs):
|
||||
super().to(*args, **kwargs)
|
||||
self.weight = self.weight.to(*args, **kwargs)
|
||||
self.bias = self.bias.to(*args, **kwargs)
|
||||
return self
|
||||
|
||||
@torch.no_grad()
|
||||
def forward(self, x):
|
||||
x_shape = x.shape
|
||||
x = x.view(-1, x_shape[-1])
|
||||
y = linear_a8_w8_b8_o8(x, self.weight, self.bias, self.a.item(), self.b.item())
|
||||
y = y.view(*x_shape[:-1], -1)
|
||||
return y
|
||||
|
||||
@staticmethod
|
||||
def from_float(module: torch.nn.Linear, input_scale, output_scale):
|
||||
int8_module = W8A8B8O8Linear(module.in_features, module.out_features)
|
||||
int8_weight, weight_scale = quantize_per_tensor_absmax(module.weight)
|
||||
alpha = input_scale * weight_scale / output_scale
|
||||
int8_module.weight = int8_weight
|
||||
int8_module.a = alpha
|
||||
|
||||
if module.bias is not None:
|
||||
int8_bias, bias_scale = quantize_per_tensor_absmax(module.bias)
|
||||
int8_module.bias = int8_bias
|
||||
beta = bias_scale / output_scale
|
||||
int8_module.b = beta
|
||||
|
||||
return int8_module
|
||||
|
||||
|
||||
# modified from torch-int: https://github.com/Guangxuan-Xiao/torch-int/blob/main/torch_int/nn/linear.py
|
||||
class W8A8BFP32OFP32Linear(torch.nn.Module):
|
||||
# For fc2 and out_proj
|
||||
def __init__(self, in_features, out_features, alpha=1.0, beta=1.0):
|
||||
super().__init__()
|
||||
self.in_features = in_features
|
||||
self.out_features = out_features
|
||||
|
||||
self.register_buffer(
|
||||
"weight",
|
||||
torch.randint(
|
||||
-127,
|
||||
127,
|
||||
(self.out_features, self.in_features),
|
||||
dtype=torch.int8,
|
||||
requires_grad=False,
|
||||
),
|
||||
)
|
||||
self.register_buffer(
|
||||
"bias",
|
||||
torch.zeros(self.out_features, dtype=torch.float32, requires_grad=False),
|
||||
)
|
||||
self.register_buffer("a", torch.tensor(alpha))
|
||||
|
||||
def _apply(self, fn):
|
||||
# prevent the bias from being converted to half
|
||||
super()._apply(fn)
|
||||
self.bias = self.bias.to(torch.float32)
|
||||
return self
|
||||
|
||||
def to(self, *args, **kwargs):
|
||||
super().to(*args, **kwargs)
|
||||
self.weight = self.weight.to(*args, **kwargs)
|
||||
self.bias = self.bias.to(*args, **kwargs)
|
||||
self.bias = self.bias.to(torch.float32)
|
||||
return self
|
||||
|
||||
@torch.no_grad()
|
||||
def forward(self, x):
|
||||
x_shape = x.shape
|
||||
x = x.view(-1, x_shape[-1])
|
||||
y = linear_a8_w8_bfp32_ofp32(x, self.weight, self.bias, self.a.item(), 1)
|
||||
y = y.view(*x_shape[:-1], -1)
|
||||
return y
|
||||
|
||||
@staticmethod
|
||||
def from_float(module: torch.nn.Linear, input_scale):
|
||||
int8_module = W8A8BFP32OFP32Linear(module.in_features, module.out_features)
|
||||
int8_weight, weight_scale = quantize_per_tensor_absmax(module.weight)
|
||||
alpha = input_scale * weight_scale
|
||||
int8_module.weight = int8_weight
|
||||
int8_module.a = alpha
|
||||
int8_module.input_scale = input_scale
|
||||
int8_module.weight_scale = weight_scale
|
||||
|
||||
if module.bias is not None:
|
||||
int8_module.bias = module.bias.to(torch.float32)
|
||||
|
||||
return int8_module
|
|
@ -0,0 +1,838 @@
|
|||
import math
|
||||
import os
|
||||
import types
|
||||
from collections import defaultdict
|
||||
from functools import partial
|
||||
from typing import List, Optional, Tuple, Union
|
||||
|
||||
import torch
|
||||
import torch.nn as nn
|
||||
import torch.nn.functional as F
|
||||
from torch_int.nn.bmm import BMM_S8T_S8N_F32T, BMM_S8T_S8N_S8T
|
||||
from transformers import PreTrainedModel
|
||||
from transformers.modeling_outputs import BaseModelOutputWithPast
|
||||
from transformers.models.llama.configuration_llama import LlamaConfig
|
||||
from transformers.models.llama.modeling_llama import (
|
||||
LLAMA_INPUTS_DOCSTRING,
|
||||
LlamaAttention,
|
||||
LlamaDecoderLayer,
|
||||
LlamaMLP,
|
||||
LlamaRotaryEmbedding,
|
||||
repeat_kv,
|
||||
rotate_half,
|
||||
)
|
||||
from transformers.utils import add_start_docstrings_to_model_forward
|
||||
|
||||
from colossalai.inference.tensor_parallel.batch_infer_state import BatchInferState
|
||||
from colossalai.kernel.triton import (
|
||||
copy_kv_cache_to_dest,
|
||||
int8_rotary_embedding_fwd,
|
||||
smooth_llama_context_attn_fwd,
|
||||
smooth_token_attention_fwd,
|
||||
)
|
||||
|
||||
from .base_model import BaseSmoothForCausalLM
|
||||
from .linear import W8A8B8O8Linear, W8A8BFP32O32LinearSiLU, W8A8BFP32OFP32Linear
|
||||
|
||||
|
||||
class LLamaSmoothquantAttention(nn.Module):
|
||||
def __init__(
|
||||
self,
|
||||
hidden_size: int,
|
||||
num_heads: int,
|
||||
):
|
||||
super().__init__()
|
||||
self.hidden_size = hidden_size
|
||||
self.num_heads = num_heads
|
||||
self.head_dim = hidden_size // num_heads
|
||||
|
||||
if (self.head_dim * num_heads) != self.hidden_size:
|
||||
raise ValueError(
|
||||
f"hidden_size must be divisible by num_heads (got `hidden_size`: {self.hidden_size}"
|
||||
f" and `num_heads`: {num_heads})."
|
||||
)
|
||||
|
||||
self.qk_bmm = BMM_S8T_S8N_F32T(1.0)
|
||||
self.pv_bmm = BMM_S8T_S8N_S8T(1.0)
|
||||
|
||||
self.k_proj = W8A8B8O8Linear(hidden_size, hidden_size)
|
||||
self.v_proj = W8A8B8O8Linear(hidden_size, hidden_size)
|
||||
self.q_proj = W8A8B8O8Linear(hidden_size, hidden_size)
|
||||
self.o_proj = W8A8BFP32OFP32Linear(hidden_size, hidden_size)
|
||||
|
||||
self.register_buffer("q_output_scale", torch.tensor([1.0]))
|
||||
self.register_buffer("k_output_scale", torch.tensor([1.0]))
|
||||
self.register_buffer("v_output_scale", torch.tensor([1.0]))
|
||||
self.register_buffer("q_rotary_output_scale", torch.tensor([1.0]))
|
||||
self.register_buffer("k_rotary_output_scale", torch.tensor([1.0]))
|
||||
self.register_buffer("out_input_scale", torch.tensor([1.0]))
|
||||
self.register_buffer("attn_input_scale", torch.tensor([1.0]))
|
||||
|
||||
self._init_rope()
|
||||
self.num_key_value_heads = num_heads
|
||||
|
||||
def _init_rope(self):
|
||||
self.rotary_emb = LlamaRotaryEmbedding(
|
||||
self.head_dim,
|
||||
max_position_embeddings=2048,
|
||||
base=10000.0,
|
||||
)
|
||||
|
||||
@staticmethod
|
||||
def pack(
|
||||
module: LlamaAttention,
|
||||
attn_input_scale: float,
|
||||
q_output_scale: float,
|
||||
k_output_scale: float,
|
||||
v_output_scale: float,
|
||||
q_rotary_output_scale: float,
|
||||
k_rotary_output_scale: float,
|
||||
out_input_scale: float,
|
||||
):
|
||||
int8_module = LLamaSmoothquantAttention(module.hidden_size, module.num_heads)
|
||||
|
||||
int8_module.attn_input_scale = torch.tensor([attn_input_scale])
|
||||
|
||||
int8_module.q_output_scale = torch.tensor([q_output_scale])
|
||||
int8_module.k_output_scale = torch.tensor([k_output_scale])
|
||||
int8_module.v_output_scale = torch.tensor([v_output_scale])
|
||||
|
||||
int8_module.q_rotary_output_scale = torch.tensor([q_rotary_output_scale])
|
||||
int8_module.k_rotary_output_scale = torch.tensor([k_rotary_output_scale])
|
||||
|
||||
int8_module.q_proj = W8A8B8O8Linear.from_float(module.q_proj, attn_input_scale, q_output_scale)
|
||||
int8_module.k_proj = W8A8B8O8Linear.from_float(module.k_proj, attn_input_scale, k_output_scale)
|
||||
int8_module.v_proj = W8A8B8O8Linear.from_float(module.v_proj, attn_input_scale, v_output_scale)
|
||||
int8_module.o_proj = W8A8BFP32OFP32Linear.from_float(module.o_proj, out_input_scale)
|
||||
|
||||
int8_module.out_input_scale = torch.tensor([out_input_scale])
|
||||
|
||||
return int8_module
|
||||
|
||||
def _shape(self, tensor: torch.Tensor, seq_len: int, bsz: int):
|
||||
return tensor.view(bsz, seq_len, self.num_heads, self.head_dim).transpose(1, 2).contiguous()
|
||||
|
||||
@torch.no_grad()
|
||||
def forward(
|
||||
self,
|
||||
hidden_states: torch.Tensor,
|
||||
rotary_emb: Tuple[torch.Tensor] = None,
|
||||
attention_mask: Optional[torch.Tensor] = None,
|
||||
position_ids: Optional[torch.LongTensor] = None,
|
||||
past_key_value: Optional[Tuple[torch.Tensor]] = None,
|
||||
output_attentions: bool = False,
|
||||
use_cache: bool = False,
|
||||
padding_mask: Optional[torch.LongTensor] = None,
|
||||
infer_state: Optional[BatchInferState] = None,
|
||||
) -> Tuple[torch.Tensor, Optional[torch.Tensor], Optional[Tuple[torch.Tensor]]]:
|
||||
bsz, q_len, _ = hidden_states.size()
|
||||
|
||||
query_states = self.q_proj(hidden_states)
|
||||
key_states = self.k_proj(hidden_states)
|
||||
value_states = self.v_proj(hidden_states)
|
||||
|
||||
cos = rotary_emb[0]
|
||||
sin = rotary_emb[1]
|
||||
|
||||
int8_rotary_embedding_fwd(
|
||||
query_states.view(-1, self.num_heads, self.head_dim),
|
||||
cos,
|
||||
sin,
|
||||
self.q_output_scale.item(),
|
||||
self.q_rotary_output_scale.item(),
|
||||
)
|
||||
int8_rotary_embedding_fwd(
|
||||
key_states.view(-1, self.num_heads, self.head_dim),
|
||||
cos,
|
||||
sin,
|
||||
self.k_output_scale.item(),
|
||||
self.k_rotary_output_scale.item(),
|
||||
)
|
||||
|
||||
def _copy_kv_to_mem_cache(layer_id, key_buffer, value_buffer, context_mem_index, mem_manager):
|
||||
copy_kv_cache_to_dest(key_buffer, context_mem_index, mem_manager.key_buffer[layer_id])
|
||||
copy_kv_cache_to_dest(value_buffer, context_mem_index, mem_manager.value_buffer[layer_id])
|
||||
return
|
||||
|
||||
query_states = query_states.view(-1, self.num_heads, self.head_dim)
|
||||
key_states = key_states.view(-1, self.num_heads, self.head_dim)
|
||||
value_states = value_states.view(-1, self.num_heads, self.head_dim)
|
||||
|
||||
if infer_state.is_context_stage:
|
||||
# first token generation
|
||||
|
||||
# copy key and value calculated in current step to memory manager
|
||||
_copy_kv_to_mem_cache(
|
||||
infer_state.decode_layer_id,
|
||||
key_states,
|
||||
value_states,
|
||||
infer_state.context_mem_index,
|
||||
infer_state.cache_manager,
|
||||
)
|
||||
|
||||
attn_output = torch.empty_like(query_states)
|
||||
|
||||
smooth_llama_context_attn_fwd(
|
||||
query_states,
|
||||
key_states,
|
||||
value_states,
|
||||
attn_output,
|
||||
self.q_rotary_output_scale.item(),
|
||||
self.k_rotary_output_scale.item(),
|
||||
self.v_output_scale.item(),
|
||||
self.out_input_scale.item(),
|
||||
infer_state.start_loc,
|
||||
infer_state.seq_len,
|
||||
q_len,
|
||||
)
|
||||
|
||||
else:
|
||||
if infer_state.decode_is_contiguous:
|
||||
# if decode is contiguous, then we copy to key cache and value cache in cache manager directly
|
||||
cache_k = infer_state.cache_manager.key_buffer[infer_state.decode_layer_id][
|
||||
infer_state.decode_mem_start : infer_state.decode_mem_end, :, :
|
||||
]
|
||||
cache_v = infer_state.cache_manager.value_buffer[infer_state.decode_layer_id][
|
||||
infer_state.decode_mem_start : infer_state.decode_mem_end, :, :
|
||||
]
|
||||
cache_k.copy_(key_states)
|
||||
cache_v.copy_(value_states)
|
||||
else:
|
||||
# if decode is not contiguous, use triton kernel to copy key and value cache
|
||||
# k, v shape: [batch_size, num_heads, head_dim/embed_size_per_head
|
||||
_copy_kv_to_mem_cache(
|
||||
infer_state.decode_layer_id,
|
||||
key_states,
|
||||
value_states,
|
||||
infer_state.decode_mem_index,
|
||||
infer_state.cache_manager,
|
||||
)
|
||||
|
||||
# (batch_size, seqlen, nheads, headdim)
|
||||
attn_output = torch.empty_like(query_states)
|
||||
|
||||
smooth_token_attention_fwd(
|
||||
query_states,
|
||||
infer_state.cache_manager.key_buffer[infer_state.decode_layer_id],
|
||||
infer_state.cache_manager.value_buffer[infer_state.decode_layer_id],
|
||||
attn_output,
|
||||
self.q_rotary_output_scale.item(),
|
||||
self.k_rotary_output_scale.item(),
|
||||
self.v_output_scale.item(),
|
||||
self.out_input_scale.item(),
|
||||
infer_state.block_loc,
|
||||
infer_state.start_loc,
|
||||
infer_state.seq_len,
|
||||
infer_state.max_len_in_batch,
|
||||
)
|
||||
|
||||
attn_output = attn_output.view(bsz, q_len, self.num_heads * self.head_dim)
|
||||
attn_output = self.o_proj(attn_output)
|
||||
|
||||
return attn_output, None, None
|
||||
|
||||
|
||||
class LlamaLayerNormQ(torch.nn.Module):
|
||||
def __init__(self, dim, eps=1e-5):
|
||||
super().__init__()
|
||||
self.input_scale = 1.0
|
||||
self.variance_epsilon = eps
|
||||
self.register_buffer("weight", torch.ones(dim, dtype=torch.float32))
|
||||
|
||||
def forward(self, x):
|
||||
ln_output_fp = torch.nn.functional.layer_norm(x, x.shape[-1:], self.weight, None, self.variance_epsilon)
|
||||
ln_output_int8 = ln_output_fp.round().clamp(-128, 127).to(torch.int8)
|
||||
return ln_output_int8
|
||||
|
||||
@staticmethod
|
||||
def from_float(module: torch.nn.LayerNorm, output_scale: float):
|
||||
assert module.weight.shape[0] == module.weight.numel()
|
||||
q_module = LlamaLayerNormQ(module.weight.shape[0], module.variance_epsilon)
|
||||
q_module.weight = module.weight / output_scale
|
||||
return q_module
|
||||
|
||||
|
||||
class LlamaSmoothquantMLP(nn.Module):
|
||||
def __init__(self, intermediate_size, hidden_size):
|
||||
super().__init__()
|
||||
self.gate_proj = W8A8BFP32O32LinearSiLU(hidden_size, intermediate_size)
|
||||
self.up_proj = W8A8BFP32OFP32Linear(hidden_size, intermediate_size)
|
||||
self.down_proj = W8A8BFP32OFP32Linear(intermediate_size, hidden_size)
|
||||
self.register_buffer("down_proj_input_scale", torch.tensor([1.0]))
|
||||
|
||||
@staticmethod
|
||||
def pack(
|
||||
mlp_module: LlamaMLP,
|
||||
gate_proj_input_scale: float,
|
||||
up_proj_input_scale: float,
|
||||
down_proj_input_scale: float,
|
||||
):
|
||||
int8_module = LlamaSmoothquantMLP(
|
||||
mlp_module.intermediate_size,
|
||||
mlp_module.hidden_size,
|
||||
)
|
||||
|
||||
int8_module.gate_proj = W8A8BFP32O32LinearSiLU.from_float(mlp_module.gate_proj, gate_proj_input_scale)
|
||||
int8_module.up_proj = W8A8BFP32OFP32Linear.from_float(mlp_module.up_proj, up_proj_input_scale)
|
||||
int8_module.down_proj = W8A8BFP32OFP32Linear.from_float(mlp_module.down_proj, down_proj_input_scale)
|
||||
int8_module.down_proj_input_scale = torch.tensor([down_proj_input_scale])
|
||||
return int8_module
|
||||
|
||||
def forward(
|
||||
self,
|
||||
hidden_states: torch.Tensor,
|
||||
):
|
||||
x_shape = hidden_states.shape
|
||||
gate_out = self.gate_proj(hidden_states)
|
||||
up_out = self.up_proj(hidden_states)
|
||||
inter_out = gate_out * up_out
|
||||
inter_out = inter_out.div_(self.down_proj_input_scale.item()).round().clamp(-128, 127).to(torch.int8)
|
||||
down_out = self.down_proj(inter_out)
|
||||
down_out = down_out.view(*x_shape[:-1], -1)
|
||||
return down_out
|
||||
|
||||
|
||||
class LlamaSmoothquantDecoderLayer(nn.Module):
|
||||
def __init__(self, config: LlamaConfig):
|
||||
super().__init__()
|
||||
self.hidden_size = config.hidden_size
|
||||
self.self_attn = LLamaSmoothquantAttention(config.hidden_size, config.num_attention_heads)
|
||||
|
||||
self.mlp = LlamaSmoothquantMLP(config.intermediate_size, config.hidden_size)
|
||||
self.input_layernorm = LlamaLayerNormQ(config.hidden_size, eps=config.rms_norm_eps)
|
||||
|
||||
self.post_attention_layernorm = LlamaLayerNormQ(config.hidden_size, eps=config.rms_norm_eps)
|
||||
|
||||
@staticmethod
|
||||
def pack(
|
||||
module: LlamaDecoderLayer,
|
||||
attn_input_scale: float,
|
||||
q_output_scale: float,
|
||||
k_output_scale: float,
|
||||
v_output_scale: float,
|
||||
q_rotary_output_scale: float,
|
||||
k_rotary_output_scale: float,
|
||||
out_input_scale: float,
|
||||
gate_input_scale: float,
|
||||
up_input_scale: float,
|
||||
down_input_scale: float,
|
||||
):
|
||||
config = module.self_attn.config
|
||||
int8_decoder_layer = LlamaSmoothquantDecoderLayer(config)
|
||||
|
||||
int8_decoder_layer.input_layernorm = LlamaLayerNormQ.from_float(module.input_layernorm, attn_input_scale)
|
||||
int8_decoder_layer.self_attn = LLamaSmoothquantAttention.pack(
|
||||
module.self_attn,
|
||||
attn_input_scale,
|
||||
q_output_scale,
|
||||
k_output_scale,
|
||||
v_output_scale,
|
||||
q_rotary_output_scale,
|
||||
k_rotary_output_scale,
|
||||
out_input_scale,
|
||||
)
|
||||
|
||||
int8_decoder_layer.post_attention_layernorm = LlamaLayerNormQ.from_float(
|
||||
module.post_attention_layernorm, gate_input_scale
|
||||
)
|
||||
|
||||
int8_decoder_layer.mlp = LlamaSmoothquantMLP.pack(
|
||||
module.mlp,
|
||||
gate_input_scale,
|
||||
up_input_scale,
|
||||
down_input_scale,
|
||||
)
|
||||
|
||||
return int8_decoder_layer
|
||||
|
||||
def forward(
|
||||
self,
|
||||
hidden_states: torch.Tensor,
|
||||
rotary_emb: Tuple[torch.Tensor] = None,
|
||||
attention_mask: Optional[torch.Tensor] = None,
|
||||
position_ids: Optional[torch.LongTensor] = None,
|
||||
past_key_value: Optional[Tuple[torch.Tensor]] = None,
|
||||
output_attentions: Optional[bool] = False,
|
||||
use_cache: Optional[bool] = False,
|
||||
padding_mask: Optional[torch.LongTensor] = None,
|
||||
infer_state: Optional[BatchInferState] = None,
|
||||
) -> Tuple[torch.FloatTensor, Optional[Tuple[torch.FloatTensor, torch.FloatTensor]]]:
|
||||
"""
|
||||
Args:
|
||||
hidden_states (`torch.FloatTensor`): input to the layer of shape `(batch, seq_len, embed_dim)`
|
||||
attention_mask (`torch.FloatTensor`, *optional*): attention mask of size
|
||||
`(batch, 1, tgt_len, src_len)` where padding elements are indicated by very large negative values.
|
||||
output_attentions (`bool`, *optional*):
|
||||
Whether or not to return the attentions tensors of all attention layers. See `attentions` under
|
||||
returned tensors for more detail.
|
||||
use_cache (`bool`, *optional*):
|
||||
If set to `True`, `past_key_values` key value states are returned and can be used to speed up decoding
|
||||
(see `past_key_values`).
|
||||
past_key_value (`Tuple(torch.FloatTensor)`, *optional*): cached past key and value projection states
|
||||
"""
|
||||
|
||||
residual = hidden_states
|
||||
|
||||
hidden_states = self.input_layernorm(hidden_states)
|
||||
|
||||
# Self Attention
|
||||
hidden_states, self_attn_weights, present_key_value = self.self_attn(
|
||||
hidden_states=hidden_states,
|
||||
rotary_emb=rotary_emb,
|
||||
attention_mask=attention_mask,
|
||||
position_ids=position_ids,
|
||||
past_key_value=past_key_value,
|
||||
output_attentions=output_attentions,
|
||||
use_cache=use_cache,
|
||||
padding_mask=padding_mask,
|
||||
infer_state=infer_state,
|
||||
)
|
||||
hidden_states = residual + hidden_states
|
||||
|
||||
# Fully Connected
|
||||
residual = hidden_states
|
||||
hidden_states = self.post_attention_layernorm(hidden_states)
|
||||
hidden_states = self.mlp(hidden_states)
|
||||
hidden_states = residual + hidden_states
|
||||
|
||||
return hidden_states, None, None
|
||||
|
||||
|
||||
class LlamaApplyRotary(nn.Module):
|
||||
def __init__(self):
|
||||
super().__init__()
|
||||
|
||||
def forward(self, x, cos, sin, position_ids):
|
||||
# The first two dimensions of cos and sin are always 1, so we can `squeeze` them.
|
||||
cos = cos.squeeze(1).squeeze(0) # [seq_len, dim]
|
||||
sin = sin.squeeze(1).squeeze(0) # [seq_len, dim]
|
||||
cos = cos[position_ids].unsqueeze(1) # [bs, 1, seq_len, dim]
|
||||
sin = sin[position_ids].unsqueeze(1) # [bs, 1, seq_len, dim]
|
||||
x_embed = (x * cos) + (rotate_half(x) * sin)
|
||||
|
||||
return x_embed
|
||||
|
||||
|
||||
# Adapted from https://github.com/huggingface/transformers/blob/main/src/transformers/models/llama/modeling_llama.py
|
||||
def llama_decoder_layer_forward(
|
||||
self,
|
||||
hidden_states: torch.Tensor,
|
||||
attention_mask: Optional[torch.Tensor] = None,
|
||||
position_ids: Optional[torch.LongTensor] = None,
|
||||
past_key_value: Optional[Tuple[torch.Tensor]] = None,
|
||||
output_attentions: bool = False,
|
||||
use_cache: bool = False,
|
||||
padding_mask: Optional[torch.LongTensor] = None,
|
||||
) -> Tuple[torch.Tensor, Optional[torch.Tensor], Optional[Tuple[torch.Tensor]]]:
|
||||
bsz, q_len, _ = hidden_states.size()
|
||||
|
||||
if self.config.pretraining_tp > 1:
|
||||
key_value_slicing = (self.num_key_value_heads * self.head_dim) // self.config.pretraining_tp
|
||||
query_slices = self.q_proj.weight.split((self.num_heads * self.head_dim) // self.config.pretraining_tp, dim=0)
|
||||
key_slices = self.k_proj.weight.split(key_value_slicing, dim=0)
|
||||
value_slices = self.v_proj.weight.split(key_value_slicing, dim=0)
|
||||
|
||||
query_states = [F.linear(hidden_states, query_slices[i]) for i in range(self.config.pretraining_tp)]
|
||||
query_states = torch.cat(query_states, dim=-1)
|
||||
|
||||
key_states = [F.linear(hidden_states, key_slices[i]) for i in range(self.config.pretraining_tp)]
|
||||
key_states = torch.cat(key_states, dim=-1)
|
||||
|
||||
value_states = [F.linear(hidden_states, value_slices[i]) for i in range(self.config.pretraining_tp)]
|
||||
value_states = torch.cat(value_states, dim=-1)
|
||||
|
||||
else:
|
||||
query_states = self.q_proj(hidden_states)
|
||||
key_states = self.k_proj(hidden_states)
|
||||
value_states = self.v_proj(hidden_states)
|
||||
|
||||
query_states = query_states.view(bsz, q_len, self.num_heads, self.head_dim).transpose(1, 2)
|
||||
key_states = key_states.view(bsz, q_len, self.num_key_value_heads, self.head_dim).transpose(1, 2)
|
||||
value_states = value_states.view(bsz, q_len, self.num_key_value_heads, self.head_dim).transpose(1, 2)
|
||||
|
||||
kv_seq_len = key_states.shape[-2]
|
||||
if past_key_value is not None:
|
||||
kv_seq_len += past_key_value[0].shape[-2]
|
||||
cos, sin = self.rotary_emb(value_states, seq_len=kv_seq_len)
|
||||
query_states = self.q_apply_rotary(query_states, cos, sin, position_ids)
|
||||
key_states = self.k_apply_rotary(key_states, cos, sin, position_ids)
|
||||
|
||||
if past_key_value is not None:
|
||||
# reuse k, v, self_attention
|
||||
key_states = torch.cat([past_key_value[0], key_states], dim=2)
|
||||
value_states = torch.cat([past_key_value[1], value_states], dim=2)
|
||||
|
||||
past_key_value = (key_states, value_states) if use_cache else None
|
||||
|
||||
key_states = repeat_kv(key_states, self.num_key_value_groups)
|
||||
value_states = repeat_kv(value_states, self.num_key_value_groups)
|
||||
|
||||
attn_weights = torch.matmul(query_states, key_states.transpose(2, 3)) / math.sqrt(self.head_dim)
|
||||
|
||||
if attn_weights.size() != (bsz, self.num_heads, q_len, kv_seq_len):
|
||||
raise ValueError(
|
||||
f"Attention weights should be of size {(bsz, self.num_heads, q_len, kv_seq_len)}, but is"
|
||||
f" {attn_weights.size()}"
|
||||
)
|
||||
|
||||
if attention_mask is not None:
|
||||
if attention_mask.size() != (bsz, 1, q_len, kv_seq_len):
|
||||
raise ValueError(
|
||||
f"Attention mask should be of size {(bsz, 1, q_len, kv_seq_len)}, but is {attention_mask.size()}"
|
||||
)
|
||||
attn_weights = attn_weights + attention_mask
|
||||
|
||||
# upcast attention to fp32
|
||||
attn_weights = nn.functional.softmax(attn_weights, dim=-1, dtype=torch.float32).to(query_states.dtype)
|
||||
attn_output = torch.matmul(attn_weights, value_states)
|
||||
|
||||
if attn_output.size() != (bsz, self.num_heads, q_len, self.head_dim):
|
||||
raise ValueError(
|
||||
f"`attn_output` should be of size {(bsz, self.num_heads, q_len, self.head_dim)}, but is"
|
||||
f" {attn_output.size()}"
|
||||
)
|
||||
|
||||
attn_output = attn_output.transpose(1, 2).contiguous()
|
||||
|
||||
attn_output = attn_output.reshape(bsz, q_len, self.hidden_size)
|
||||
|
||||
if self.config.pretraining_tp > 1:
|
||||
attn_output = attn_output.split(self.hidden_size // self.config.pretraining_tp, dim=2)
|
||||
o_proj_slices = self.o_proj.weight.split(self.hidden_size // self.config.pretraining_tp, dim=1)
|
||||
attn_output = sum([F.linear(attn_output[i], o_proj_slices[i]) for i in range(self.config.pretraining_tp)])
|
||||
else:
|
||||
attn_output = self.o_proj(attn_output)
|
||||
|
||||
if not output_attentions:
|
||||
attn_weights = None
|
||||
|
||||
return attn_output, attn_weights, past_key_value
|
||||
|
||||
|
||||
def init_to_get_rotary(config, base=10000, use_elem=False):
|
||||
"""
|
||||
This function initializes the rotary positional embedding, it is compatible for all models and is called in ShardFormer
|
||||
Args:
|
||||
base : calculation arg
|
||||
use_elem : activated when using chatglm-based models
|
||||
"""
|
||||
config.head_dim_ = config.hidden_size // config.num_attention_heads
|
||||
if not hasattr(config, "rope_scaling"):
|
||||
rope_scaling_factor = 1.0
|
||||
else:
|
||||
rope_scaling_factor = config.rope_scaling.factor if config.rope_scaling is not None else 1.0
|
||||
|
||||
if hasattr(config, "max_sequence_length"):
|
||||
max_seq_len = config.max_sequence_length
|
||||
elif hasattr(config, "max_position_embeddings"):
|
||||
max_seq_len = config.max_position_embeddings * rope_scaling_factor
|
||||
else:
|
||||
max_seq_len = 2048 * rope_scaling_factor
|
||||
base = float(base)
|
||||
|
||||
# NTK ref: https://www.reddit.com/r/LocalLLaMA/comments/14lz7j5/ntkaware_scaled_rope_allows_llama_models_to_have/
|
||||
try:
|
||||
ntk_alpha = float(os.environ.get("INFER_NTK_ALPHA", 1))
|
||||
assert ntk_alpha >= 1
|
||||
if ntk_alpha > 1:
|
||||
print(f"Note: NTK enabled, alpha set to {ntk_alpha}")
|
||||
max_seq_len *= ntk_alpha
|
||||
base = base * (ntk_alpha ** (config.head_dim_ / (config.head_dim_ - 2))) # Base change formula
|
||||
except:
|
||||
pass
|
||||
|
||||
n_elem = config.head_dim_
|
||||
if use_elem:
|
||||
n_elem //= 2
|
||||
|
||||
inv_freq = 1.0 / (base ** (torch.arange(0, n_elem, 2, device="cpu", dtype=torch.float32) / n_elem))
|
||||
t = torch.arange(max_seq_len + 1024 * 64, device="cpu", dtype=torch.float32) / rope_scaling_factor
|
||||
freqs = torch.outer(t, inv_freq)
|
||||
|
||||
_cos_cached = torch.cos(freqs).to(torch.float)
|
||||
_sin_cached = torch.sin(freqs).to(torch.float)
|
||||
return _cos_cached, _sin_cached
|
||||
|
||||
|
||||
# Adapted from https://github.com/huggingface/transformers/blob/main/src/transformers/models/llama/modeling_llama.py
|
||||
@add_start_docstrings_to_model_forward(LLAMA_INPUTS_DOCSTRING)
|
||||
def llama_model_forward(
|
||||
self,
|
||||
input_ids: torch.LongTensor = None,
|
||||
attention_mask: Optional[torch.Tensor] = None,
|
||||
position_ids: Optional[torch.LongTensor] = None,
|
||||
past_key_values: Optional[List[torch.FloatTensor]] = None,
|
||||
inputs_embeds: Optional[torch.FloatTensor] = None,
|
||||
use_cache: Optional[bool] = None,
|
||||
output_attentions: Optional[bool] = None,
|
||||
output_hidden_states: Optional[bool] = None,
|
||||
return_dict: Optional[bool] = None,
|
||||
) -> Union[Tuple, BaseModelOutputWithPast]:
|
||||
output_attentions = output_attentions if output_attentions is not None else self.config.output_attentions
|
||||
output_hidden_states = (
|
||||
output_hidden_states if output_hidden_states is not None else self.config.output_hidden_states
|
||||
)
|
||||
use_cache = use_cache if use_cache is not None else self.config.use_cache
|
||||
|
||||
return_dict = return_dict if return_dict is not None else self.config.use_return_dict
|
||||
|
||||
# retrieve input_ids and inputs_embeds
|
||||
if input_ids is not None and inputs_embeds is not None:
|
||||
raise ValueError("You cannot specify both input_ids and inputs_embeds at the same time")
|
||||
elif input_ids is not None:
|
||||
batch_size, seq_length = input_ids.shape
|
||||
elif inputs_embeds is not None:
|
||||
batch_size, seq_length, _ = inputs_embeds.shape
|
||||
else:
|
||||
raise ValueError("You have to specify either input_ids or inputs_embeds")
|
||||
|
||||
infer_state = self.infer_state
|
||||
if infer_state.is_context_stage:
|
||||
past_key_values_length = 0
|
||||
else:
|
||||
past_key_values_length = infer_state.max_len_in_batch - 1
|
||||
|
||||
seq_length_with_past = seq_length + past_key_values_length
|
||||
|
||||
# NOTE: differentiate with prefill stage
|
||||
# block_loc require different value-assigning method for two different stage
|
||||
# NOTE: differentiate with prefill stage
|
||||
# block_loc require different value-assigning method for two different stage
|
||||
if infer_state.is_context_stage:
|
||||
infer_state.context_mem_index = infer_state.cache_manager.alloc(infer_state.total_token_num)
|
||||
infer_state.init_block_loc(
|
||||
infer_state.block_loc, infer_state.seq_len, seq_length, infer_state.context_mem_index
|
||||
)
|
||||
else:
|
||||
alloc_mem = infer_state.cache_manager.alloc_contiguous(batch_size)
|
||||
if alloc_mem is not None:
|
||||
infer_state.decode_is_contiguous = True
|
||||
infer_state.decode_mem_index = alloc_mem[0]
|
||||
infer_state.decode_mem_start = alloc_mem[1]
|
||||
infer_state.decode_mem_end = alloc_mem[2]
|
||||
infer_state.block_loc[:, seq_length_with_past - 1] = infer_state.decode_mem_index
|
||||
else:
|
||||
print(f" *** Encountered allocation non-contiguous")
|
||||
print(f" infer_state.cache_manager.max_len_in_batch: {infer_state.max_len_in_batch}")
|
||||
infer_state.decode_is_contiguous = False
|
||||
alloc_mem = infer_state.cache_manager.alloc(batch_size)
|
||||
infer_state.decode_mem_index = alloc_mem
|
||||
infer_state.block_loc[:, seq_length_with_past - 1] = infer_state.decode_mem_index
|
||||
|
||||
if position_ids is None:
|
||||
device = input_ids.device if input_ids is not None else inputs_embeds.device
|
||||
position_ids = torch.arange(
|
||||
past_key_values_length, seq_length + past_key_values_length, dtype=torch.long, device=device
|
||||
)
|
||||
position_ids = position_ids.unsqueeze(0).view(-1, seq_length)
|
||||
else:
|
||||
position_ids = position_ids.view(-1, seq_length).long()
|
||||
|
||||
if inputs_embeds is None:
|
||||
inputs_embeds = self.embed_tokens(input_ids)
|
||||
# embed positions
|
||||
if attention_mask is None:
|
||||
attention_mask = torch.ones((batch_size, seq_length_with_past), dtype=torch.bool, device=inputs_embeds.device)
|
||||
padding_mask = None
|
||||
else:
|
||||
if 0 in attention_mask:
|
||||
padding_mask = attention_mask
|
||||
else:
|
||||
padding_mask = None
|
||||
|
||||
attention_mask = self._prepare_decoder_attention_mask(
|
||||
attention_mask, (batch_size, seq_length), inputs_embeds, past_key_values_length
|
||||
)
|
||||
|
||||
hidden_states = inputs_embeds
|
||||
|
||||
if self.gradient_checkpointing and self.training:
|
||||
raise NotImplementedError("not implement gradient_checkpointing and training options ")
|
||||
|
||||
if past_key_values_length == 0:
|
||||
position_cos = torch.index_select(self._cos_cached, 0, position_ids.view(-1)).view(
|
||||
position_ids.view(-1).shape[0], -1
|
||||
)
|
||||
position_sin = torch.index_select(self._sin_cached, 0, position_ids.view(-1)).view(
|
||||
position_ids.view(-1).shape[0], -1
|
||||
)
|
||||
else:
|
||||
position_cos = torch.index_select(self._cos_cached, 0, position_ids.view(-1)).view(batch_size, -1)
|
||||
position_sin = torch.index_select(self._sin_cached, 0, position_ids.view(-1)).view(batch_size, -1)
|
||||
|
||||
# decoder layers
|
||||
all_hidden_states = () if output_hidden_states else None
|
||||
all_self_attns = () if output_attentions else None
|
||||
next_decoder_cache = () if use_cache else None
|
||||
infer_state.decode_layer_id = 0
|
||||
for idx, decoder_layer in enumerate(self.layers):
|
||||
if output_hidden_states:
|
||||
all_hidden_states += (hidden_states,)
|
||||
|
||||
past_key_value = past_key_values[idx] if past_key_values is not None else None
|
||||
|
||||
layer_outputs = decoder_layer(
|
||||
hidden_states,
|
||||
rotary_emb=(position_cos, position_sin),
|
||||
attention_mask=attention_mask,
|
||||
position_ids=position_ids,
|
||||
past_key_value=past_key_value,
|
||||
output_attentions=output_attentions,
|
||||
use_cache=use_cache,
|
||||
padding_mask=padding_mask,
|
||||
infer_state=infer_state,
|
||||
)
|
||||
|
||||
hidden_states = layer_outputs[0]
|
||||
infer_state.decode_layer_id += 1
|
||||
|
||||
if use_cache:
|
||||
next_decoder_cache += (layer_outputs[2 if output_attentions else 1],)
|
||||
|
||||
if output_attentions:
|
||||
all_self_attns += (layer_outputs[1],)
|
||||
|
||||
hidden_states = self.norm(hidden_states)
|
||||
|
||||
# add hidden states from the last decoder layer
|
||||
if output_hidden_states:
|
||||
all_hidden_states += (hidden_states,)
|
||||
|
||||
infer_state.is_context_stage = False
|
||||
infer_state.start_loc = infer_state.start_loc + torch.arange(0, batch_size, dtype=torch.int32, device="cuda")
|
||||
infer_state.seq_len += 1
|
||||
infer_state.max_len_in_batch += 1
|
||||
|
||||
next_cache = next_decoder_cache if use_cache else None
|
||||
if not return_dict:
|
||||
return tuple(v for v in [hidden_states, next_cache, all_hidden_states, all_self_attns] if v is not None)
|
||||
return BaseModelOutputWithPast(
|
||||
last_hidden_state=hidden_states,
|
||||
past_key_values=next_cache,
|
||||
hidden_states=all_hidden_states,
|
||||
attentions=all_self_attns,
|
||||
)
|
||||
|
||||
|
||||
class SmoothLlamaForCausalLM(BaseSmoothForCausalLM):
|
||||
layer_type = "LlamaDecoderLayer"
|
||||
|
||||
def __init__(self, model: PreTrainedModel, quantized: bool = False):
|
||||
super().__init__(model, quantized)
|
||||
|
||||
# Adatped from https://github.com/mit-han-lab/smoothquant/blob/main/smoothquant/calibration.py
|
||||
def get_act_dict(
|
||||
self,
|
||||
tokenizer,
|
||||
dataset,
|
||||
num_samples=512,
|
||||
seq_len=512,
|
||||
):
|
||||
llama_model = self.model
|
||||
|
||||
llama_model.eval()
|
||||
device = next(llama_model.parameters()).device
|
||||
# print("model:", llama_model)
|
||||
act_dict = defaultdict(dict)
|
||||
|
||||
def stat_io_hook(m, x, y, name):
|
||||
if isinstance(x, tuple):
|
||||
x = x[0]
|
||||
if name not in act_dict or "input" not in act_dict[name]:
|
||||
act_dict[name]["input"] = x.detach().abs().max().item()
|
||||
else:
|
||||
act_dict[name]["input"] = max(act_dict[name]["input"], x.detach().abs().max().item())
|
||||
if isinstance(y, tuple):
|
||||
y = y[0]
|
||||
if name not in act_dict or "output" not in act_dict[name]:
|
||||
act_dict[name]["output"] = y.detach().abs().max().item()
|
||||
else:
|
||||
act_dict[name]["output"] = max(act_dict[name]["output"], y.detach().abs().max().item())
|
||||
|
||||
for name, m in llama_model.named_modules():
|
||||
if isinstance(m, LlamaAttention):
|
||||
setattr(m, "q_apply_rotary", LlamaApplyRotary())
|
||||
setattr(m, "k_apply_rotary", LlamaApplyRotary())
|
||||
m.forward = types.MethodType(llama_decoder_layer_forward, m)
|
||||
|
||||
hooks = []
|
||||
for name, m in llama_model.named_modules():
|
||||
if isinstance(m, LlamaApplyRotary):
|
||||
hooks.append(m.register_forward_hook(partial(stat_io_hook, name=name)))
|
||||
if isinstance(m, torch.nn.Linear):
|
||||
hooks.append(m.register_forward_hook(partial(stat_io_hook, name=name)))
|
||||
|
||||
self.collect_act_dict(llama_model, tokenizer, dataset, act_dict, device, num_samples, seq_len)
|
||||
|
||||
for hook in hooks:
|
||||
hook.remove()
|
||||
return act_dict
|
||||
|
||||
def smooth_fn(self, scales, alpha=0.5):
|
||||
model = self.model
|
||||
for name, module in model.named_modules():
|
||||
if isinstance(module, LlamaDecoderLayer):
|
||||
attn_ln = module.input_layernorm
|
||||
qkv = [module.self_attn.q_proj, module.self_attn.k_proj, module.self_attn.v_proj]
|
||||
qkv_input_scales = scales[name + ".self_attn.q_proj"]
|
||||
self.smooth_ln_fcs(attn_ln, qkv, qkv_input_scales, alpha)
|
||||
|
||||
def create_quantized_model(model):
|
||||
llama_config = model.config
|
||||
for i, layer in enumerate(model.model.layers):
|
||||
model.model.layers[i] = LlamaSmoothquantDecoderLayer(llama_config)
|
||||
|
||||
model.model.forward = types.MethodType(llama_model_forward, model.model)
|
||||
cos, sin = init_to_get_rotary(llama_config)
|
||||
model.model.register_buffer("_cos_cached", cos)
|
||||
model.model.register_buffer("_sin_cached", sin)
|
||||
|
||||
def quantized(
|
||||
self,
|
||||
tokenizer,
|
||||
dataset,
|
||||
num_samples=512,
|
||||
seq_len=512,
|
||||
alpha=0.5,
|
||||
):
|
||||
llama_model = self.model
|
||||
llama_config = llama_model.config
|
||||
|
||||
act_scales = self.get_act_scales(llama_model, tokenizer, dataset, num_samples, seq_len)
|
||||
|
||||
self.smooth_fn(act_scales, alpha)
|
||||
|
||||
act_dict = self.get_act_dict(tokenizer, dataset, num_samples, seq_len)
|
||||
decoder_layer_scales = []
|
||||
|
||||
for idx in range(llama_config.num_hidden_layers):
|
||||
scale_dict = {}
|
||||
scale_dict["attn_input_scale"] = act_dict[f"model.layers.{idx}.self_attn.q_proj"]["input"] / 127
|
||||
scale_dict["q_output_scale"] = act_dict[f"model.layers.{idx}.self_attn.q_proj"]["output"] / 127
|
||||
scale_dict["k_output_scale"] = act_dict[f"model.layers.{idx}.self_attn.k_proj"]["output"] / 127
|
||||
scale_dict["v_output_scale"] = act_dict[f"model.layers.{idx}.self_attn.v_proj"]["output"] / 127
|
||||
|
||||
scale_dict["q_rotary_output_scale"] = (
|
||||
act_dict[f"model.layers.{idx}.self_attn.q_apply_rotary"]["output"] / 127
|
||||
)
|
||||
scale_dict["k_rotary_output_scale"] = (
|
||||
act_dict[f"model.layers.{idx}.self_attn.k_apply_rotary"]["output"] / 127
|
||||
)
|
||||
|
||||
scale_dict["out_input_scale"] = act_dict[f"model.layers.{idx}.self_attn.o_proj"]["input"] / 127
|
||||
|
||||
scale_dict["gate_input_scale"] = act_dict[f"model.layers.{idx}.mlp.gate_proj"]["input"] / 127
|
||||
scale_dict["up_input_scale"] = act_dict[f"model.layers.{idx}.mlp.up_proj"]["input"] / 127
|
||||
scale_dict["down_input_scale"] = act_dict[f"model.layers.{idx}.mlp.down_proj"]["input"] / 127
|
||||
|
||||
decoder_layer_scales.append(scale_dict)
|
||||
|
||||
for i, layer in enumerate(llama_model.model.layers):
|
||||
orig_layer = layer
|
||||
llama_model.model.layers[i] = LlamaSmoothquantDecoderLayer.pack(orig_layer, **decoder_layer_scales[i])
|
||||
|
||||
llama_model.model.forward = types.MethodType(llama_model_forward, llama_model.model)
|
||||
|
||||
cos, sin = init_to_get_rotary(llama_config)
|
||||
llama_model.model.register_buffer("_cos_cached", cos.to(self.model.device))
|
||||
llama_model.model.register_buffer("_sin_cached", sin.to(self.model.device))
|
|
@ -0,0 +1,118 @@
|
|||
# might want to consider combine with InferenceConfig in colossalai/ppinference/inference_config.py later
|
||||
from dataclasses import dataclass
|
||||
|
||||
import torch
|
||||
from transformers.tokenization_utils_base import BatchEncoding
|
||||
|
||||
from .kvcache_manager import MemoryManager
|
||||
|
||||
|
||||
# adapted from: lightllm/server/router/model_infer/infer_batch.py
|
||||
@dataclass
|
||||
class BatchInferState:
|
||||
r"""
|
||||
Information to be passed and used for a batch of inputs during
|
||||
a single model forward
|
||||
"""
|
||||
batch_size: int
|
||||
max_len_in_batch: int
|
||||
|
||||
cache_manager: MemoryManager = None
|
||||
|
||||
block_loc: torch.Tensor = None
|
||||
start_loc: torch.Tensor = None
|
||||
seq_len: torch.Tensor = None
|
||||
past_key_values_len: int = None
|
||||
|
||||
is_context_stage: bool = False
|
||||
context_mem_index: torch.Tensor = None
|
||||
decode_is_contiguous: bool = None
|
||||
decode_mem_start: int = None
|
||||
decode_mem_end: int = None
|
||||
decode_mem_index: torch.Tensor = None
|
||||
decode_layer_id: int = None
|
||||
|
||||
device: torch.device = torch.device("cuda")
|
||||
|
||||
@property
|
||||
def total_token_num(self):
|
||||
# return self.batch_size * self.max_len_in_batch
|
||||
assert self.seq_len is not None and self.seq_len.size(0) > 0
|
||||
return int(torch.sum(self.seq_len))
|
||||
|
||||
def set_cache_manager(self, manager: MemoryManager):
|
||||
self.cache_manager = manager
|
||||
|
||||
# adapted from: https://github.com/ModelTC/lightllm/blob/28c1267cfca536b7b4f28e921e03de735b003039/lightllm/common/infer_utils.py#L1
|
||||
@staticmethod
|
||||
def init_block_loc(
|
||||
b_loc: torch.Tensor, seq_len: torch.Tensor, max_len_in_batch: int, alloc_mem_index: torch.Tensor
|
||||
):
|
||||
"""in-place update block loc mapping based on the sequence length of the inputs in current bath"""
|
||||
start_index = 0
|
||||
seq_len_numpy = seq_len.cpu().numpy()
|
||||
for i, cur_seq_len in enumerate(seq_len_numpy):
|
||||
b_loc[i, max_len_in_batch - cur_seq_len : max_len_in_batch] = alloc_mem_index[
|
||||
start_index : start_index + cur_seq_len
|
||||
]
|
||||
start_index += cur_seq_len
|
||||
return
|
||||
|
||||
@classmethod
|
||||
def init_from_batch(
|
||||
cls,
|
||||
batch: torch.Tensor,
|
||||
max_input_len: int,
|
||||
max_output_len: int,
|
||||
cache_manager: MemoryManager,
|
||||
):
|
||||
if not isinstance(batch, (BatchEncoding, dict, list, torch.Tensor)):
|
||||
raise TypeError(f"batch type {type(batch)} is not supported in prepare_batch_state")
|
||||
|
||||
input_ids_list = None
|
||||
attention_mask = None
|
||||
|
||||
if isinstance(batch, (BatchEncoding, dict)):
|
||||
input_ids_list = batch["input_ids"]
|
||||
attention_mask = batch["attention_mask"]
|
||||
else:
|
||||
input_ids_list = batch
|
||||
if isinstance(input_ids_list[0], int): # for a single input
|
||||
input_ids_list = [input_ids_list]
|
||||
attention_mask = [attention_mask] if attention_mask is not None else attention_mask
|
||||
|
||||
batch_size = len(input_ids_list)
|
||||
|
||||
seq_start_indexes = torch.zeros(batch_size, dtype=torch.int32, device="cuda")
|
||||
seq_lengths = torch.zeros(batch_size, dtype=torch.int32, device="cuda")
|
||||
start_index = 0
|
||||
|
||||
max_len_in_batch = -1
|
||||
if isinstance(batch, (BatchEncoding, dict)):
|
||||
for i, attn_mask in enumerate(attention_mask):
|
||||
curr_seq_len = len(attn_mask)
|
||||
seq_lengths[i] = curr_seq_len
|
||||
seq_start_indexes[i] = start_index
|
||||
start_index += curr_seq_len
|
||||
max_len_in_batch = curr_seq_len if curr_seq_len > max_len_in_batch else max_len_in_batch
|
||||
else:
|
||||
length = max(len(input_id) for input_id in input_ids_list)
|
||||
for i, input_ids in enumerate(input_ids_list):
|
||||
curr_seq_len = length
|
||||
seq_lengths[i] = curr_seq_len
|
||||
seq_start_indexes[i] = start_index
|
||||
start_index += curr_seq_len
|
||||
max_len_in_batch = curr_seq_len if curr_seq_len > max_len_in_batch else max_len_in_batch
|
||||
block_loc = torch.zeros((batch_size, max_input_len + max_output_len), dtype=torch.long, device="cuda")
|
||||
|
||||
return cls(
|
||||
batch_size=batch_size,
|
||||
max_len_in_batch=max_len_in_batch,
|
||||
seq_len=seq_lengths.to("cuda"),
|
||||
start_loc=seq_start_indexes.to("cuda"),
|
||||
block_loc=block_loc,
|
||||
decode_layer_id=0,
|
||||
past_key_values_len=0,
|
||||
is_context_stage=True,
|
||||
cache_manager=cache_manager,
|
||||
)
|
|
@ -0,0 +1,106 @@
|
|||
"""
|
||||
Refered/Modified from lightllm/common/mem_manager.py
|
||||
of the ModelTC/lightllm GitHub repository
|
||||
https://github.com/ModelTC/lightllm/blob/050af3ce65edca617e2f30ec2479397d5bb248c9/lightllm/common/mem_manager.py
|
||||
we slightly changed it to make it suitable for our colossal-ai shardformer TP-engine design.
|
||||
"""
|
||||
import torch
|
||||
from transformers.utils import logging
|
||||
|
||||
|
||||
class MemoryManager:
|
||||
r"""
|
||||
Manage token block indexes and allocate physical memory for key and value cache
|
||||
|
||||
Args:
|
||||
size: maximum token number used as the size of key and value buffer
|
||||
dtype: data type of cached key and value
|
||||
head_num: number of heads the memory manager is responsible for
|
||||
head_dim: embedded size per head
|
||||
layer_num: the number of layers in the model
|
||||
device: device used to store the key and value cache
|
||||
"""
|
||||
|
||||
def __init__(
|
||||
self,
|
||||
size: int,
|
||||
dtype: torch.dtype,
|
||||
head_num: int,
|
||||
head_dim: int,
|
||||
layer_num: int,
|
||||
device: torch.device = torch.device("cuda"),
|
||||
):
|
||||
self.logger = logging.get_logger(__name__)
|
||||
self.available_size = size
|
||||
self.max_len_in_batch = 0
|
||||
self._init_mem_states(size, device)
|
||||
self._init_kv_buffers(size, device, dtype, head_num, head_dim, layer_num)
|
||||
|
||||
def _init_mem_states(self, size, device):
|
||||
"""Initialize tensors used to manage memory states"""
|
||||
self.mem_state = torch.ones((size,), dtype=torch.bool, device=device)
|
||||
self.mem_cum_sum = torch.empty((size,), dtype=torch.int32, device=device)
|
||||
self.indexes = torch.arange(0, size, dtype=torch.long, device=device)
|
||||
|
||||
def _init_kv_buffers(self, size, device, dtype, head_num, head_dim, layer_num):
|
||||
"""Initialize key buffer and value buffer on specified device"""
|
||||
self.key_buffer = [
|
||||
torch.empty((size, head_num, head_dim), dtype=dtype, device=device) for _ in range(layer_num)
|
||||
]
|
||||
self.value_buffer = [
|
||||
torch.empty((size, head_num, head_dim), dtype=dtype, device=device) for _ in range(layer_num)
|
||||
]
|
||||
|
||||
@torch.no_grad()
|
||||
def alloc(self, required_size):
|
||||
"""allocate space of required_size by providing indexes representing available physical spaces"""
|
||||
if required_size > self.available_size:
|
||||
self.logger.warning(f"No enough cache: required_size {required_size} " f"left_size {self.available_size}")
|
||||
return None
|
||||
torch.cumsum(self.mem_state, dim=0, dtype=torch.int32, out=self.mem_cum_sum)
|
||||
select_index = torch.logical_and(self.mem_cum_sum <= required_size, self.mem_state == 1)
|
||||
select_index = self.indexes[select_index]
|
||||
self.mem_state[select_index] = 0
|
||||
self.available_size -= len(select_index)
|
||||
return select_index
|
||||
|
||||
@torch.no_grad()
|
||||
def alloc_contiguous(self, required_size):
|
||||
"""allocate contiguous space of required_size"""
|
||||
if required_size > self.available_size:
|
||||
self.logger.warning(f"No enough cache: required_size {required_size} " f"left_size {self.available_size}")
|
||||
return None
|
||||
torch.cumsum(self.mem_state, dim=0, dtype=torch.int32, out=self.mem_cum_sum)
|
||||
sum_size = len(self.mem_cum_sum)
|
||||
loc_sums = (
|
||||
self.mem_cum_sum[required_size - 1 :]
|
||||
- self.mem_cum_sum[0 : sum_size - required_size + 1]
|
||||
+ self.mem_state[0 : sum_size - required_size + 1]
|
||||
)
|
||||
can_used_loc = self.indexes[0 : sum_size - required_size + 1][loc_sums == required_size]
|
||||
if can_used_loc.shape[0] == 0:
|
||||
self.logger.info(
|
||||
f"No enough contiguous cache: required_size {required_size} " f"left_size {self.available_size}"
|
||||
)
|
||||
return None
|
||||
start_loc = can_used_loc[0]
|
||||
select_index = self.indexes[start_loc : start_loc + required_size]
|
||||
self.mem_state[select_index] = 0
|
||||
self.available_size -= len(select_index)
|
||||
start = start_loc.item()
|
||||
end = start + required_size
|
||||
return select_index, start, end
|
||||
|
||||
@torch.no_grad()
|
||||
def free(self, free_index):
|
||||
"""free memory by updating memory states based on given indexes"""
|
||||
self.available_size += free_index.shape[0]
|
||||
self.mem_state[free_index] = 1
|
||||
|
||||
@torch.no_grad()
|
||||
def free_all(self):
|
||||
"""free all memory by updating memory states"""
|
||||
self.available_size = len(self.mem_state)
|
||||
self.mem_state[:] = 1
|
||||
self.max_len_in_batch = 0
|
||||
self.logger.info("freed all space of memory manager")
|
|
@ -0,0 +1,67 @@
|
|||
"""
|
||||
Utils for model inference
|
||||
"""
|
||||
import os
|
||||
|
||||
import torch
|
||||
|
||||
from colossalai.kernel.triton.copy_kv_cache_dest import copy_kv_cache_to_dest
|
||||
|
||||
|
||||
def copy_kv_to_mem_cache(layer_id, key_buffer, value_buffer, context_mem_index, mem_manager):
|
||||
"""
|
||||
This function copies the key and value cache to the memory cache
|
||||
Args:
|
||||
layer_id : id of current layer
|
||||
key_buffer : key cache
|
||||
value_buffer : value cache
|
||||
context_mem_index : index of memory cache in kv cache manager
|
||||
mem_manager : cache manager
|
||||
"""
|
||||
copy_kv_cache_to_dest(key_buffer, context_mem_index, mem_manager.key_buffer[layer_id])
|
||||
copy_kv_cache_to_dest(value_buffer, context_mem_index, mem_manager.value_buffer[layer_id])
|
||||
|
||||
|
||||
def init_to_get_rotary(self, base=10000, use_elem=False):
|
||||
"""
|
||||
This function initializes the rotary positional embedding, it is compatible for all models and is called in ShardFormer
|
||||
Args:
|
||||
self : Model that holds the rotary positional embedding
|
||||
base : calculation arg
|
||||
use_elem : activated when using chatglm-based models
|
||||
"""
|
||||
self.config.head_dim_ = self.config.hidden_size // self.config.num_attention_heads
|
||||
if not hasattr(self.config, "rope_scaling"):
|
||||
rope_scaling_factor = 1.0
|
||||
else:
|
||||
rope_scaling_factor = self.config.rope_scaling.factor if self.config.rope_scaling is not None else 1.0
|
||||
|
||||
if hasattr(self.config, "max_sequence_length"):
|
||||
max_seq_len = self.config.max_sequence_length
|
||||
elif hasattr(self.config, "max_position_embeddings"):
|
||||
max_seq_len = self.config.max_position_embeddings * rope_scaling_factor
|
||||
else:
|
||||
max_seq_len = 2048 * rope_scaling_factor
|
||||
base = float(base)
|
||||
|
||||
# NTK ref: https://www.reddit.com/r/LocalLLaMA/comments/14lz7j5/ntkaware_scaled_rope_allows_llama_models_to_have/
|
||||
ntk_alpha = os.environ.get("INFER_NTK_ALPHA", None)
|
||||
|
||||
if ntk_alpha is not None:
|
||||
ntk_alpha = float(ntk_alpha)
|
||||
assert ntk_alpha >= 1, "NTK alpha must be greater than or equal to 1"
|
||||
if ntk_alpha > 1:
|
||||
print(f"Note: NTK enabled, alpha set to {ntk_alpha}")
|
||||
max_seq_len *= ntk_alpha
|
||||
base = base * (ntk_alpha ** (self.head_dim_ / (self.head_dim_ - 2))) # Base change formula
|
||||
|
||||
n_elem = self.config.head_dim_
|
||||
if use_elem:
|
||||
n_elem //= 2
|
||||
|
||||
inv_freq = 1.0 / (base ** (torch.arange(0, n_elem, 2, device="cpu", dtype=torch.float32) / n_elem))
|
||||
t = torch.arange(max_seq_len + 1024 * 64, device="cpu", dtype=torch.float32) / rope_scaling_factor
|
||||
freqs = torch.outer(t, inv_freq)
|
||||
|
||||
self._cos_cached = torch.cos(freqs).to(torch.float16).cuda()
|
||||
self._sin_cached = torch.sin(freqs).to(torch.float16).cuda()
|
|
@ -20,7 +20,10 @@ from colossalai.inference.tensor_parallel.batch_infer_state import BatchInferSta
|
|||
from colossalai.kernel.triton import bloom_context_attn_fwd, copy_kv_cache_to_dest, token_attention_fwd
|
||||
|
||||
try:
|
||||
from lightllm.models.bloom.triton_kernel.context_flashattention_nopad import context_attention_fwd as lightllm_bloom_context_attention_fwd
|
||||
from lightllm.models.bloom.triton_kernel.context_flashattention_nopad import (
|
||||
context_attention_fwd as lightllm_bloom_context_attention_fwd,
|
||||
)
|
||||
|
||||
HAS_LIGHTLLM_KERNEL = True
|
||||
except:
|
||||
HAS_LIGHTLLM_KERNEL = False
|
|
@ -4,7 +4,6 @@ import torch
|
|||
from torch.nn import LayerNorm
|
||||
|
||||
import colossalai.shardformer.layer as col_nn
|
||||
from colossalai.shardformer.modeling.bloom import build_bloom_alibi_tensor_fn
|
||||
from colossalai.shardformer.policies.base_policy import ModulePolicyDescription, SubModuleReplacementDescription
|
||||
from colossalai.shardformer.policies.bloom import BloomForCausalLMPolicy
|
||||
|
||||
|
@ -40,33 +39,36 @@ class BloomModelInferPolicy(BloomForCausalLMPolicy):
|
|||
policy = super().module_policy()
|
||||
if self.shard_config.inference_gptq:
|
||||
from colossalai.inference.quant.gptq.cai_gptq import ColCaiQuantLinear, RowCaiQuantLinear
|
||||
policy[BloomBlock] = ModulePolicyDescription(attribute_replacement={
|
||||
"self_attention.hidden_size": self.model.config.hidden_size // self.shard_config.tensor_parallel_size,
|
||||
"self_attention.split_size": self.model.config.hidden_size // self.shard_config.tensor_parallel_size,
|
||||
"self_attention.num_heads": self.model.config.n_head // self.shard_config.tensor_parallel_size,
|
||||
},
|
||||
sub_module_replacement=[
|
||||
SubModuleReplacementDescription(
|
||||
suffix="self_attention.query_key_value",
|
||||
target_module=ColCaiQuantLinear,
|
||||
kwargs={'split_num': 3}),
|
||||
SubModuleReplacementDescription(
|
||||
suffix="self_attention.dense",
|
||||
target_module=RowCaiQuantLinear,
|
||||
kwargs={'split_num': 1}),
|
||||
SubModuleReplacementDescription(
|
||||
suffix="self_attention.attention_dropout",
|
||||
target_module=col_nn.DropoutForParallelInput,
|
||||
),
|
||||
SubModuleReplacementDescription(
|
||||
suffix="mlp.dense_h_to_4h",
|
||||
target_module=ColCaiQuantLinear,
|
||||
kwargs={'split_num': 1}),
|
||||
SubModuleReplacementDescription(
|
||||
suffix="mlp.dense_4h_to_h",
|
||||
target_module=RowCaiQuantLinear,
|
||||
kwargs={'split_num': 1}),
|
||||
])
|
||||
|
||||
policy[BloomBlock] = ModulePolicyDescription(
|
||||
attribute_replacement={
|
||||
"self_attention.hidden_size": self.model.config.hidden_size
|
||||
// self.shard_config.tensor_parallel_size,
|
||||
"self_attention.split_size": self.model.config.hidden_size
|
||||
// self.shard_config.tensor_parallel_size,
|
||||
"self_attention.num_heads": self.model.config.n_head // self.shard_config.tensor_parallel_size,
|
||||
},
|
||||
sub_module_replacement=[
|
||||
SubModuleReplacementDescription(
|
||||
suffix="self_attention.query_key_value",
|
||||
target_module=ColCaiQuantLinear,
|
||||
kwargs={"split_num": 3},
|
||||
),
|
||||
SubModuleReplacementDescription(
|
||||
suffix="self_attention.dense", target_module=RowCaiQuantLinear, kwargs={"split_num": 1}
|
||||
),
|
||||
SubModuleReplacementDescription(
|
||||
suffix="self_attention.attention_dropout",
|
||||
target_module=col_nn.DropoutForParallelInput,
|
||||
),
|
||||
SubModuleReplacementDescription(
|
||||
suffix="mlp.dense_h_to_4h", target_module=ColCaiQuantLinear, kwargs={"split_num": 1}
|
||||
),
|
||||
SubModuleReplacementDescription(
|
||||
suffix="mlp.dense_4h_to_h", target_module=RowCaiQuantLinear, kwargs={"split_num": 1}
|
||||
),
|
||||
],
|
||||
)
|
||||
# NOTE set inference mode to shard config
|
||||
self.shard_config._infer()
|
||||
|
|
@ -13,6 +13,7 @@ from ..modeling.llama import LlamaInferenceForwards
|
|||
|
||||
try:
|
||||
from lightllm.models.llama.triton_kernel.rmsnorm import rmsnorm_forward as lightllm_rmsnorm_forward
|
||||
|
||||
HAS_TRITON_RMSNORM = True
|
||||
except:
|
||||
print("you should install triton from https://github.com/openai/triton")
|
||||
|
@ -21,6 +22,7 @@ except:
|
|||
|
||||
def get_triton_rmsnorm_forward():
|
||||
if HAS_TRITON_RMSNORM:
|
||||
|
||||
def _triton_rmsnorm_forward(self: LlamaRMSNorm, hidden_states: torch.Tensor):
|
||||
return lightllm_rmsnorm_forward(hidden_states, self.weight.data, self.variance_epsilon)
|
||||
|
|
@ -7,7 +7,7 @@ import torch.cuda
|
|||
from torch.nn import Module
|
||||
from torch.utils._pytree import tree_map
|
||||
|
||||
from colossalai.inference.pipeline.microbatch_manager import MicroBatchManager, Status
|
||||
from colossalai.inference.hybridengine.microbatch_manager import MicroBatchManager, Status
|
||||
from colossalai.pipeline.p2p import PipelineP2PCommunication
|
||||
from colossalai.pipeline.stage_manager import PipelineStageManager
|
||||
from colossalai.utils.cuda import get_current_device
|
||||
|
|
|
@ -0,0 +1,134 @@
|
|||
import argparse
|
||||
import time
|
||||
|
||||
import torch
|
||||
import torch.distributed as dist
|
||||
import transformers
|
||||
|
||||
import colossalai
|
||||
from colossalai.inference import PPInferEngine
|
||||
from colossalai.inference.pipeline.policies import LlamaModelInferPolicy
|
||||
|
||||
GIGABYTE = 1024**3
|
||||
MEGABYTE = 1024 * 1024
|
||||
|
||||
colossalai.launch_from_torch(config={})
|
||||
|
||||
|
||||
def data_gen(batch_size: int = 4, seq_len: int = 512):
|
||||
input_ids = torch.randint(10, 30000, (1, seq_len), dtype=torch.int32)
|
||||
attention_mask = torch.ones((1, seq_len), dtype=torch.int32)
|
||||
data = dict(input_ids=input_ids, attention_mask=attention_mask)
|
||||
for k, v in data.items():
|
||||
if torch.is_tensor(v) or "Tensor" in v.__class__.__name__:
|
||||
new_shape = [1] * v.dim()
|
||||
new_shape[0] = batch_size
|
||||
data[k] = v.to("cuda").repeat(*new_shape)
|
||||
return data
|
||||
|
||||
|
||||
def print_details_info(timestamps, model_config, args, whole_end2end):
|
||||
if dist.get_rank() == 0:
|
||||
prefill = []
|
||||
encoder = []
|
||||
end2end = []
|
||||
for timestamp in timestamps:
|
||||
prefill.append(timestamp[1] - timestamp[0])
|
||||
encoder.append(
|
||||
sum(timestamp[i + 1] - timestamp[i] for i in range(1, len(timestamp) - 1)) / (len(timestamp) - 2)
|
||||
)
|
||||
end2end.append(timestamp[-1] - timestamp[0])
|
||||
print(whole_end2end)
|
||||
with open(
|
||||
f"{args.log_path}/llama-{args.model}{args.dtype}_pp{args.pp_size}_{args.seq_len}_{args.new_length}_bsz{args.batch_size}_mbsz{args.mb_size}.log",
|
||||
"w+",
|
||||
) as f:
|
||||
mb_avg_end2end = sum(end2end) / len(end2end)
|
||||
mb_avg_latency = mb_avg_end2end / (args.new_length * args.mb_size)
|
||||
whole_avg_latency = whole_end2end / (args.new_length * args.batch_size)
|
||||
num_layers = getattr(model_config, "num_layers", model_config.num_hidden_layers)
|
||||
num_parameters = num_layers * model_config.hidden_size * model_config.hidden_size * 12 / args.pp_size
|
||||
if args.dtype in ["fp16", "bf16"]:
|
||||
num_bytes = 2
|
||||
else:
|
||||
num_bytes = 4
|
||||
|
||||
f.write(
|
||||
f"llama-{args.model}{args.dtype}_pp{args.pp_size}, input_len:{args.seq_len}, output_len:{args.new_length}, bsz:{args.batch_size}, mbsz:{args.mb_size}\n"
|
||||
)
|
||||
f.write("Average prefill time: {0:8.2f} ms\n".format(sum(prefill) / len(prefill) * 1000))
|
||||
f.write("Average encode time: {0:8.2f} ms\n".format(sum(encoder) / len(encoder) * 1000))
|
||||
f.write("Average micro batch end2end time: {0:8.2f} ms\n".format(mb_avg_end2end * 1000))
|
||||
f.write("Average micro batch Per Token Latency: {0:8.2f} ms\n".format(mb_avg_latency * 1000))
|
||||
f.write("Whole batch end2end time: {0:8.2f} ms\n".format(whole_end2end * 1000))
|
||||
f.write("Whole batch Per Token Latency: {0:8.2f} ms\n".format(whole_avg_latency * 1000))
|
||||
f.write("Throughput: {} tokens/s\n".format((1000 / (whole_avg_latency * 1000))))
|
||||
f.write("flops: {0:8.2f} TFlops/s\n".format(1 / whole_avg_latency * num_parameters * num_bytes / 1e12))
|
||||
f.write("----------------------------------------------------------\n")
|
||||
|
||||
if torch.cuda.is_available():
|
||||
current_device = torch.cuda.current_device()
|
||||
|
||||
# free memory and the total available memory in bytes
|
||||
global_free_memory, total_GPU_memory_occupied = torch.cuda.mem_get_info()
|
||||
memory_allocated = torch.cuda.memory_allocated()
|
||||
max_memory_allocated = torch.cuda.max_memory_allocated()
|
||||
memory_reserved = torch.cuda.memory_reserved()
|
||||
max_memory_reserved = torch.cuda.max_memory_reserved()
|
||||
with open(
|
||||
f"{args.log_path}/llama-{args.model}{args.dtype}_pp{args.pp_size}_{args.seq_len}_{args.new_length}_bsz{args.batch_size}_mbsz{args.mb_size}.log",
|
||||
"a",
|
||||
) as f:
|
||||
f.write(
|
||||
f"\nCurrently using GPU: {current_device}\n"
|
||||
f"free memory : {global_free_memory / GIGABYTE:.4f} GB,\n"
|
||||
f"total memory: {total_GPU_memory_occupied / GIGABYTE:.4f} GB,\n"
|
||||
f"memory allocated: {memory_allocated / GIGABYTE:.4f} GB,\n"
|
||||
f"Max CUDA memory allocated: {max_memory_allocated / GIGABYTE:.4f} GB,\n"
|
||||
f"memory reserved/cached: {memory_reserved / GIGABYTE:.4f} GB,\n"
|
||||
f"Max CUDA memory reserved/cached: {max_memory_reserved / GIGABYTE:.4f} GB,\n"
|
||||
)
|
||||
|
||||
|
||||
if __name__ == "__main__":
|
||||
parser = argparse.ArgumentParser()
|
||||
parser.add_argument("--model", default="toy", help="the size of model")
|
||||
parser.add_argument("-b", "--batch_size", type=int, default=8, help="batch size")
|
||||
parser.add_argument("-s", "--seq_len", type=int, default=8, help="sequence length")
|
||||
parser.add_argument("--new_length", type=int, default=4, help="new tokens length")
|
||||
parser.add_argument("--mb_size", type=int, default=1, help="micro_batch_size")
|
||||
parser.add_argument("--pp_size", type=int, default=2, help="pipeline size")
|
||||
parser.add_argument("--log_path", type=str, default="./log", help="where to store the benchmark log")
|
||||
parser.add_argument("--dtype", type=str, default="fp16", help="data type")
|
||||
args = parser.parse_args()
|
||||
|
||||
if args.model == "toy":
|
||||
model = transformers.LlamaForCausalLM(transformers.LlamaConfig(num_hidden_layers=8))
|
||||
elif args.model == "7b":
|
||||
model = transformers.LlamaForCausalLM(transformers.AutoConfig.from_pretrained("decapoda-research/llama-7b-hf"))
|
||||
elif args.model == "13b":
|
||||
model = transformers.LlamaForCausalLM(transformers.AutoConfig.from_pretrained("decapoda-research/llama-13b-hf"))
|
||||
else:
|
||||
raise NotImplementedError
|
||||
|
||||
engine = PPInferEngine(
|
||||
pp_size=args.pp_size,
|
||||
dtype=args.dtype,
|
||||
micro_batch_size=args.mb_size,
|
||||
new_length=args.new_length,
|
||||
model=model,
|
||||
model_policy=LlamaModelInferPolicy(),
|
||||
verbose=True,
|
||||
max_batch_size=args.mb_size,
|
||||
max_input_len=args.seq_len,
|
||||
max_output_len=args.seq_len + args.new_length + 256,
|
||||
)
|
||||
data = data_gen(args.batch_size, args.seq_len)
|
||||
|
||||
torch.cuda.synchronize()
|
||||
whole_end2end = time.time()
|
||||
output, timestamps = engine.inference([data])
|
||||
torch.cuda.synchronize()
|
||||
whole_end2end = time.time() - whole_end2end
|
||||
|
||||
print_details_info(timestamps, model.config, args, whole_end2end)
|
|
@ -0,0 +1,50 @@
|
|||
script_dir=$(cd "$(dirname "$0")" && pwd)
|
||||
cd "${script_dir}"
|
||||
|
||||
# 7b, fp16, 2 gpu, 1024, 128
|
||||
for BATCH_SIZE in 2 4 8 16; do
|
||||
CUDA_VISIBLE_DEVICES=0,1 colossalai run --nproc_per_node 2 --master_port 29800 ./benchmark.py \
|
||||
--model="7b" \
|
||||
--dtype="fp16" \
|
||||
--batch_size=${BATCH_SIZE} \
|
||||
--seq_len=1024 \
|
||||
--new_length=128 \
|
||||
--mb_size=$((${BATCH_SIZE}/2)) \
|
||||
--pp_size=2
|
||||
done
|
||||
|
||||
# 7b, fp16, 2 gpu, 512, 512
|
||||
for BATCH_SIZE in 2 4 8 16 32; do
|
||||
CUDA_VISIBLE_DEVICES=0,1 colossalai run --nproc_per_node 2 --master_port 29800 ./benchmark.py \
|
||||
--model="7b" \
|
||||
--dtype="fp16" \
|
||||
--batch_size=${BATCH_SIZE} \
|
||||
--seq_len=512 \
|
||||
--new_length=512 \
|
||||
--mb_size=$((${BATCH_SIZE}/2)) \
|
||||
--pp_size=2
|
||||
done
|
||||
|
||||
# 7b, fp16, 2 gpu, 1024, 128
|
||||
for BATCH_SIZE in 2 4 8; do
|
||||
CUDA_VISIBLE_DEVICES=0,1 colossalai run --nproc_per_node 2 --master_port 29800 ./benchmark.py \
|
||||
--model="13b" \
|
||||
--dtype="fp16" \
|
||||
--batch_size=${BATCH_SIZE} \
|
||||
--seq_len=1024 \
|
||||
--new_length=128 \
|
||||
--mb_size=$((${BATCH_SIZE}/2)) \
|
||||
--pp_size=2
|
||||
done
|
||||
|
||||
# 13b, fp16, 2 gpu, 512, 512
|
||||
for BATCH_SIZE in 2 4 8 16; do
|
||||
CUDA_VISIBLE_DEVICES=0,1 colossalai run --nproc_per_node 2 --master_port 29800 ./benchmark.py \
|
||||
--model="13b" \
|
||||
--dtype="fp16" \
|
||||
--batch_size=${BATCH_SIZE} \
|
||||
--seq_len=512 \
|
||||
--new_length=512 \
|
||||
--mb_size=$((${BATCH_SIZE}/2)) \
|
||||
--pp_size=2
|
||||
done
|
|
@ -1,70 +0,0 @@
|
|||
import pytest
|
||||
import torch
|
||||
from packaging import version
|
||||
from transformers import BloomForCausalLM
|
||||
from transformers.models.bloom.configuration_bloom import BloomConfig
|
||||
|
||||
import colossalai
|
||||
from colossalai.inference.tensor_parallel import TPInferEngine
|
||||
from colossalai.logging import disable_existing_loggers
|
||||
from colossalai.shardformer import ShardConfig
|
||||
from colossalai.testing import clear_cache_before_run, parameterize, rerun_if_address_is_in_use, spawn
|
||||
|
||||
try:
|
||||
import lightllm
|
||||
HAS_LIGHTLLM_KERNEL = True
|
||||
except:
|
||||
HAS_LIGHTLLM_KERNEL = False
|
||||
|
||||
TP_SIZE = 2
|
||||
MAX_BATCH_SIZE = 4
|
||||
MAX_INPUT_LEN = 16
|
||||
MAX_OUTPUT_LEN = 32
|
||||
|
||||
CUDA_SUPPORT = version.parse(torch.version.cuda) > version.parse("11.5")
|
||||
|
||||
|
||||
@parameterize(
|
||||
"test_config",
|
||||
[
|
||||
{
|
||||
"tp_size": TP_SIZE,
|
||||
}
|
||||
],
|
||||
)
|
||||
def run(test_config):
|
||||
bloom_config = BloomConfig(num_hidden_layers=2, bos_token_id=0, eos_token_id=1, vocab_size=1200, hidden_size=1024)
|
||||
model = BloomForCausalLM(bloom_config)
|
||||
model = model.half()
|
||||
|
||||
shard_config = ShardConfig(
|
||||
enable_tensor_parallelism=True if test_config["tp_size"] > 1 else False, inference_only=True
|
||||
)
|
||||
infer_engine = TPInferEngine(model, shard_config, MAX_BATCH_SIZE, MAX_INPUT_LEN, MAX_OUTPUT_LEN)
|
||||
generate_kwargs = dict(max_new_tokens=MAX_OUTPUT_LEN, do_sample=False)
|
||||
|
||||
input_tokens = {
|
||||
"input_ids": torch.randint(1, 1000, (MAX_BATCH_SIZE, MAX_INPUT_LEN), device="cuda"),
|
||||
"attention_mask": torch.ones((MAX_BATCH_SIZE, MAX_INPUT_LEN), device="cuda"),
|
||||
}
|
||||
outputs = infer_engine.generate(input_tokens, **generate_kwargs)
|
||||
|
||||
assert outputs is not None
|
||||
|
||||
|
||||
def check_bloom(rank, world_size, port):
|
||||
disable_existing_loggers()
|
||||
colossalai.launch(config={}, rank=rank, world_size=world_size, host="localhost", port=port, backend="nccl")
|
||||
run()
|
||||
|
||||
|
||||
@pytest.mark.skipif(not CUDA_SUPPORT or not HAS_LIGHTLLM_KERNEL, reason="kv-cache manager engine requires cuda version to be higher than 11.5")
|
||||
@pytest.mark.dist
|
||||
@rerun_if_address_is_in_use()
|
||||
@clear_cache_before_run()
|
||||
def test_bloom_infer():
|
||||
spawn(check_bloom, TP_SIZE)
|
||||
|
||||
|
||||
if __name__ == "__main__":
|
||||
test_bloom_infer()
|
|
@ -1,83 +0,0 @@
|
|||
import os
|
||||
|
||||
import pytest
|
||||
import torch
|
||||
from packaging import version
|
||||
|
||||
import colossalai
|
||||
from colossalai.inference.tensor_parallel.engine import TPInferEngine
|
||||
from colossalai.logging import disable_existing_loggers
|
||||
from colossalai.shardformer import ShardConfig
|
||||
from colossalai.shardformer.modeling.chatglm2_6b.configuration_chatglm import ChatGLMConfig
|
||||
from colossalai.shardformer.modeling.chatglm2_6b.modeling_chatglm import ChatGLMForConditionalGeneration
|
||||
from colossalai.testing import clear_cache_before_run, parameterize, rerun_if_address_is_in_use, spawn
|
||||
|
||||
try:
|
||||
import lightllm # noqa
|
||||
|
||||
HAS_LIGHTLLM_KERNEL = True
|
||||
except:
|
||||
HAS_LIGHTLLM_KERNEL = False
|
||||
|
||||
os.environ["TRANSFORMERS_NO_ADVISORY_WARNINGS"] = "true"
|
||||
TPSIZE = 2
|
||||
BATCH_SIZE = 8
|
||||
MAX_INPUT_LEN = 12
|
||||
MAX_OUTPUT_LEN = 100
|
||||
CUDA_SUPPORT = version.parse(torch.version.cuda) > version.parse("11.5")
|
||||
|
||||
|
||||
@parameterize(
|
||||
"test_config",
|
||||
[
|
||||
{
|
||||
"tp_size": TPSIZE,
|
||||
}
|
||||
],
|
||||
)
|
||||
def run_chatglm2_test(test_config):
|
||||
chatglm_config = ChatGLMConfig(
|
||||
num_layers=2,
|
||||
vocab_size=1200,
|
||||
use_cache=True,
|
||||
multi_query_attention=True,
|
||||
multi_query_group_num=2,
|
||||
num_attention_heads=8,
|
||||
hidden_size=1024,
|
||||
)
|
||||
model = ChatGLMForConditionalGeneration(chatglm_config)
|
||||
model = model.half()
|
||||
|
||||
shard_config = ShardConfig(
|
||||
enable_tensor_parallelism=True if test_config["tp_size"] > 1 else False, inference_only=True
|
||||
)
|
||||
infer_engine = TPInferEngine(model, shard_config, BATCH_SIZE, MAX_INPUT_LEN, MAX_OUTPUT_LEN)
|
||||
generate_kwargs = dict(max_new_tokens=MAX_OUTPUT_LEN, do_sample=False)
|
||||
|
||||
input_tokens = {
|
||||
"input_ids": torch.randint(1, 1000, (BATCH_SIZE, MAX_INPUT_LEN), device="cuda"),
|
||||
"attention_mask": torch.ones((BATCH_SIZE, MAX_INPUT_LEN), device="cuda"),
|
||||
}
|
||||
outputs = infer_engine.generate(input_tokens, **generate_kwargs)
|
||||
assert outputs is not None
|
||||
|
||||
|
||||
def check_chatglm2(rank, world_size, port):
|
||||
disable_existing_loggers()
|
||||
colossalai.launch(config={}, rank=rank, world_size=world_size, host="localhost", port=port, backend="nccl")
|
||||
run_chatglm2_test()
|
||||
|
||||
|
||||
@pytest.mark.skipif(
|
||||
not CUDA_SUPPORT or not HAS_LIGHTLLM_KERNEL,
|
||||
reason="kv-cache manager engine requires cuda version to be higher than 11.5",
|
||||
)
|
||||
@pytest.mark.dist
|
||||
@rerun_if_address_is_in_use()
|
||||
@clear_cache_before_run()
|
||||
def test_chatglm2():
|
||||
spawn(check_chatglm2, TPSIZE)
|
||||
|
||||
|
||||
if __name__ == "__main__":
|
||||
test_chatglm2()
|
|
@ -1,14 +0,0 @@
|
|||
engine_config:
|
||||
model: MODEL_PATH
|
||||
tensor_parallel_size: 1
|
||||
max_batch_size: 2
|
||||
max_input_len: 1024
|
||||
max_output_len: 512
|
||||
# config for app router deployment
|
||||
# Resources assigned to each model replica. This should correspond to Ray AIR ScalingConfig.
|
||||
router_config:
|
||||
max_total_token_num: 4096
|
||||
batch_max_tokens: 4096
|
||||
disable_log_stats: False
|
||||
log_stats_interval: 10
|
||||
model: MODEL_PATH
|
|
@ -1,61 +0,0 @@
|
|||
import asyncio
|
||||
import os
|
||||
import uuid
|
||||
|
||||
import pytest
|
||||
|
||||
import colossalai
|
||||
from colossalai.inference.async_engine import Async_Engine
|
||||
from colossalai.inference.dynamic_batching.ray_init_config import RayInitConfig
|
||||
from colossalai.inference.dynamic_batching.sampling_params import SamplingParams
|
||||
from colossalai.testing import clear_cache_before_run, rerun_if_address_is_in_use, spawn
|
||||
|
||||
PATH = "config.yaml"
|
||||
|
||||
|
||||
def run_async_engine(path: str):
|
||||
if not os.path.exists(path):
|
||||
return
|
||||
|
||||
config = RayInitConfig.from_yaml_path(path)
|
||||
engine_config = config.engine_config_data
|
||||
model = engine_config.model
|
||||
if model is None or not os.path.exists(model):
|
||||
return
|
||||
|
||||
prompt = "Introduce some landmarks in London.\n The Tower of London is a historic castle on the north bank of the River Thames in central London. It was founded towards the end of 10"
|
||||
sampling_params = SamplingParams()
|
||||
asyncio.run(asy_for_loop_test(config, prompt, sampling_params))
|
||||
|
||||
|
||||
async def get_result(engine, prompt, sampling_params):
|
||||
request_id = str(uuid.uuid4().hex)
|
||||
results = engine.generate(request_id, prompt, sampling_params)
|
||||
async for result in results:
|
||||
# print(result)
|
||||
assert result is not None
|
||||
|
||||
|
||||
async def asy_for_loop_test(config, prompt, sampling_params):
|
||||
router_config = config.router_config_data
|
||||
engine_config = config.engine_config_data
|
||||
engine = Async_Engine(router_config=router_config, engine_config=engine_config)
|
||||
for i in range(10):
|
||||
print("in for loop", i)
|
||||
await get_result(engine, prompt, sampling_params)
|
||||
|
||||
|
||||
def check_async_engine(rank, world_size, port):
|
||||
colossalai.launch(config={}, rank=rank, world_size=world_size, host="localhost", port=port, backend="nccl")
|
||||
run_async_engine(PATH)
|
||||
|
||||
|
||||
@pytest.mark.dist
|
||||
@rerun_if_address_is_in_use()
|
||||
@clear_cache_before_run()
|
||||
def test_async_engine():
|
||||
spawn(check_async_engine, 1)
|
||||
|
||||
|
||||
if __name__ == "__main__":
|
||||
test_async_engine()
|
|
@ -1,95 +0,0 @@
|
|||
import pytest
|
||||
from transformers import LlamaForCausalLM
|
||||
from transformers.models.llama.configuration_llama import LlamaConfig
|
||||
|
||||
import colossalai
|
||||
from colossalai.inference.dynamic_batching.io_struct import Req
|
||||
from colossalai.inference.dynamic_batching.sampling_params import SamplingParams
|
||||
from colossalai.inference.manager import DynamicBatchManager
|
||||
from colossalai.inference.tensor_parallel import TPInferEngine
|
||||
from colossalai.shardformer import ShardConfig
|
||||
from colossalai.testing import clear_cache_before_run, rerun_if_address_is_in_use, spawn
|
||||
|
||||
TP_SIZE = 1
|
||||
BATCH_SIZE = 2
|
||||
MAX_INPUT_LEN = 48
|
||||
MAX_OUTPUT_LEN = 256
|
||||
|
||||
|
||||
def run():
|
||||
sampling_params = SamplingParams()
|
||||
|
||||
req1 = Req(0, [1], sampling_params)
|
||||
req2 = Req(1, [2], sampling_params)
|
||||
req3 = Req(2, [3], sampling_params)
|
||||
# req 1-3 are initiliazed as token forward requests
|
||||
req4 = Req(3, [10, 10, 10, 9, 1], sampling_params)
|
||||
waiting_list = []
|
||||
waiting_list.append(req1)
|
||||
waiting_list.append(req2)
|
||||
waiting_list.append(req3)
|
||||
|
||||
# init model and tp engine
|
||||
llama_config = LlamaConfig(num_hidden_layers=2, bos_token_id=0, eos_token_id=1, vocab_size=1200, hidden_size=1024)
|
||||
model = LlamaForCausalLM(llama_config)
|
||||
model = model.half()
|
||||
|
||||
shard_config = ShardConfig(enable_tensor_parallelism=False, inference_only=True)
|
||||
infer_engine = TPInferEngine(model, shard_config, BATCH_SIZE, MAX_INPUT_LEN, MAX_OUTPUT_LEN)
|
||||
|
||||
dynamic_batch_manager = DynamicBatchManager(
|
||||
tp_engine=infer_engine,
|
||||
max_total_token_num=640,
|
||||
batch_max_tokens=608,
|
||||
eos_id=0,
|
||||
log_stats=False,
|
||||
log_stats_interval=10,
|
||||
waiting_req_list=waiting_list,
|
||||
model="llama",
|
||||
)
|
||||
before_add = len(dynamic_batch_manager.req_queue)
|
||||
|
||||
# test add req function
|
||||
dynamic_batch_manager.add_req(req4.request_id, req4.prompt_ids, req4.sample_params)
|
||||
assert len(dynamic_batch_manager.req_queue.waiting_req_list) == before_add + 1
|
||||
|
||||
# test abort function
|
||||
dynamic_batch_manager.abort(req4.request_id)
|
||||
assert dynamic_batch_manager.req_queue.waiting_req_list[-1].aborted == True
|
||||
|
||||
# test filter batch function, loop_for_fwd, _step, _init_batch and _prefill/_decode batch are tested
|
||||
batch = dynamic_batch_manager.req_queue.generate_new_batch()
|
||||
assert len(batch) == 2
|
||||
|
||||
dynamic_batch_manager._init_batch(batch)
|
||||
assert dynamic_batch_manager.engine.cache[batch.batch_id] is not None
|
||||
|
||||
batch.reqs[0].has_generate_finished = True
|
||||
# filter one finished
|
||||
batch.filter_finished()
|
||||
dynamic_batch_manager._filter_batch(batch)
|
||||
assert len(dynamic_batch_manager.engine.cache) == 1
|
||||
|
||||
# test merge batch
|
||||
new_batch = dynamic_batch_manager.req_queue.generate_new_batch(batch)
|
||||
assert len(new_batch) == 1
|
||||
dynamic_batch_manager._init_batch(new_batch)
|
||||
dynamic_batch_manager._merge_batch(batch, new_batch)
|
||||
|
||||
assert len(dynamic_batch_manager.engine.cache[batch.batch_id]) == 2
|
||||
|
||||
|
||||
def check_dynamic_batching_manager(rank, world_size, port):
|
||||
colossalai.launch(config={}, rank=rank, world_size=world_size, host="localhost", port=port, backend="nccl")
|
||||
run()
|
||||
|
||||
|
||||
@pytest.mark.dist
|
||||
@rerun_if_address_is_in_use()
|
||||
@clear_cache_before_run()
|
||||
def test_dynamic_batching_manager():
|
||||
spawn(check_dynamic_batching_manager, 1)
|
||||
|
||||
|
||||
if __name__ == "__main__":
|
||||
test_dynamic_batching_manager()
|
|
@ -1,84 +0,0 @@
|
|||
from dataclasses import dataclass
|
||||
|
||||
import pytest
|
||||
import torch
|
||||
from packaging import version
|
||||
from transformers import LlamaForCausalLM
|
||||
from transformers.models.llama.configuration_llama import LlamaConfig
|
||||
|
||||
import colossalai
|
||||
from colossalai.inference.dynamic_batching.io_struct import Req
|
||||
from colossalai.inference.dynamic_batching.sampling_params import SamplingParams
|
||||
from colossalai.inference.manager import start_dynamic_batching
|
||||
from colossalai.inference.tensor_parallel import TPInferEngine
|
||||
from colossalai.shardformer import ShardConfig
|
||||
from colossalai.testing import clear_cache_before_run, rerun_if_address_is_in_use, spawn
|
||||
|
||||
TP_SIZE = 1
|
||||
MAX_BATCH_SIZE = 2
|
||||
MAX_INPUT_LEN = 5
|
||||
MAX_OUTPUT_LEN = 16
|
||||
CUDA_SUPPORT = version.parse(torch.version.cuda) > version.parse("11.5")
|
||||
|
||||
|
||||
@dataclass
|
||||
class args:
|
||||
max_total_token_num: int
|
||||
batch_max_tokens: int
|
||||
model: str
|
||||
eos_id: int
|
||||
disable_log_stats: bool
|
||||
log_stats_interval: int
|
||||
|
||||
|
||||
def run():
|
||||
arg = args(
|
||||
max_total_token_num=42,
|
||||
model="llama",
|
||||
batch_max_tokens=42,
|
||||
eos_id=0,
|
||||
disable_log_stats=False,
|
||||
log_stats_interval=10,
|
||||
)
|
||||
sampling_params = SamplingParams()
|
||||
|
||||
req1 = Req(0, [0, 0, 10, 6, 8], sampling_params)
|
||||
req2 = Req(1, [10, 10, 10, 10, 10], sampling_params)
|
||||
req3 = Req(2, [0, 0, 10, 10, 10], sampling_params)
|
||||
req4 = Req(3, [0, 0, 10, 10, 10], sampling_params)
|
||||
|
||||
waiting_list = []
|
||||
waiting_list.append(req1)
|
||||
waiting_list.append(req2)
|
||||
waiting_list.append(req3)
|
||||
waiting_list.append(req4)
|
||||
|
||||
llama_config = LlamaConfig(num_hidden_layers=2, bos_token_id=0, eos_token_id=1, vocab_size=30000, hidden_size=1024)
|
||||
model = LlamaForCausalLM(llama_config)
|
||||
model = model.half()
|
||||
|
||||
shard_config = ShardConfig(enable_tensor_parallelism=True if TP_SIZE > 1 else False, inference_only=True)
|
||||
|
||||
infer_engine = TPInferEngine(model, shard_config, MAX_BATCH_SIZE, MAX_INPUT_LEN, MAX_OUTPUT_LEN)
|
||||
batch_manager = start_dynamic_batching(arg, tp_engine=infer_engine, waiting_req_list=waiting_list)
|
||||
|
||||
ans_gen = batch_manager.generate(request_id=5, prompts="hello", sampling_params=sampling_params)
|
||||
for result in ans_gen:
|
||||
assert result is not None
|
||||
|
||||
|
||||
def check_dynamic_forward(rank, world_size, port):
|
||||
colossalai.launch(config={}, rank=rank, world_size=world_size, host="localhost", port=port, backend="nccl")
|
||||
run()
|
||||
|
||||
|
||||
@pytest.mark.skipif(not CUDA_SUPPORT, reason="kv-cache manager engine requires cuda version to be higher than 11.5")
|
||||
@pytest.mark.dist
|
||||
@rerun_if_address_is_in_use()
|
||||
@clear_cache_before_run()
|
||||
def test_dynamic_batching():
|
||||
spawn(check_dynamic_forward, TP_SIZE)
|
||||
|
||||
|
||||
if __name__ == "__main__":
|
||||
test_dynamic_batching()
|
|
@ -1,66 +0,0 @@
|
|||
import asyncio
|
||||
import os
|
||||
import uuid
|
||||
|
||||
import pytest
|
||||
|
||||
import colossalai
|
||||
from colossalai.inference.dynamic_batching.ray_dist_init import Driver
|
||||
from colossalai.inference.dynamic_batching.ray_init_config import RayInitConfig
|
||||
from colossalai.inference.dynamic_batching.sampling_params import SamplingParams
|
||||
from colossalai.testing import clear_cache_before_run, rerun_if_address_is_in_use, spawn
|
||||
|
||||
PATH = "config.yaml"
|
||||
|
||||
|
||||
def run_ray_dist(path: str):
|
||||
if not os.path.exists(path):
|
||||
return
|
||||
config = RayInitConfig.from_yaml_path(path)
|
||||
router_config = config.router_config_data
|
||||
engine_config = config.engine_config_data
|
||||
model = engine_config.model
|
||||
if model is None or not os.path.exists(model):
|
||||
return
|
||||
driver = Driver(router_config=router_config, engine_config=engine_config)
|
||||
prompt = "Introduce some landmarks in Beijing"
|
||||
|
||||
request_id = str(uuid.uuid4().hex)
|
||||
sampling_params = SamplingParams()
|
||||
print("sampling_params: ", sampling_params)
|
||||
|
||||
async def get_result(request_id, prompt, sampling_params):
|
||||
return await driver.async_generate(request_id, prompt, sampling_params)
|
||||
|
||||
for test_async in [True, False]:
|
||||
if test_async:
|
||||
print("test_async: ", test_async)
|
||||
result = asyncio.run(get_result(request_id, prompt, sampling_params))
|
||||
assert result is not None
|
||||
print("result: ", result)
|
||||
else:
|
||||
print("test_async: ", test_async)
|
||||
result = driver.generate(request_id, prompt, sampling_params)
|
||||
assert result is not None
|
||||
print("result: ", result)
|
||||
|
||||
is_running = None
|
||||
is_running = driver.is_running()
|
||||
assert is_running is not None
|
||||
print("is_running: ", is_running)
|
||||
|
||||
|
||||
def check_ray_dist(rank, world_size, port):
|
||||
colossalai.launch(config={}, rank=rank, world_size=world_size, host="localhost", port=port, backend="nccl")
|
||||
run_ray_dist(PATH)
|
||||
|
||||
|
||||
@pytest.mark.dist
|
||||
@rerun_if_address_is_in_use()
|
||||
@clear_cache_before_run()
|
||||
def test_ray_dist():
|
||||
spawn(check_ray_dist, 1)
|
||||
|
||||
|
||||
if __name__ == "__main__":
|
||||
test_ray_dist()
|
|
@ -1,3 +1,5 @@
|
|||
import importlib.util
|
||||
|
||||
import pytest
|
||||
import torch
|
||||
import torch.distributed as dist
|
||||
|
@ -9,9 +11,9 @@ from colossalai.inference import BloomModelInferPolicy, CaiInferEngine
|
|||
from colossalai.testing import clear_cache_before_run, parameterize, rerun_if_address_is_in_use, spawn
|
||||
|
||||
CUDA_SUPPORT = version.parse(torch.version.cuda) > version.parse("11.5")
|
||||
try:
|
||||
HAS_LIGHTLLM_KERNEL = True
|
||||
except:
|
||||
HAS_LIGHTLLM_KERNEL = True
|
||||
|
||||
if importlib.util.find_spec("lightllm") is None:
|
||||
HAS_LIGHTLLM_KERNEL = False
|
||||
|
||||
|
||||
|
|
|
@ -1,3 +1,5 @@
|
|||
import importlib.util
|
||||
|
||||
import pytest
|
||||
import torch
|
||||
import torch.distributed as dist
|
||||
|
@ -9,9 +11,12 @@ from colossalai.inference import CaiInferEngine, LlamaModelInferPolicy
|
|||
from colossalai.testing import clear_cache_before_run, parameterize, rerun_if_address_is_in_use, spawn
|
||||
|
||||
CUDA_SUPPORT = version.parse(torch.version.cuda) > version.parse("11.5")
|
||||
try:
|
||||
HAS_LIGHTLLM_KERNEL = True
|
||||
except:
|
||||
|
||||
import importlib.util
|
||||
|
||||
HAS_LIGHTLLM_KERNEL = True
|
||||
|
||||
if importlib.util.find_spec("lightllm") is None:
|
||||
HAS_LIGHTLLM_KERNEL = False
|
||||
|
||||
|
||||
|
|
|
@ -1,102 +0,0 @@
|
|||
from itertools import accumulate
|
||||
|
||||
import pytest
|
||||
import torch
|
||||
from packaging import version
|
||||
from transformers import BloomConfig, BloomForCausalLM
|
||||
from transformers.tokenization_utils_base import BatchEncoding
|
||||
|
||||
import colossalai
|
||||
from colossalai.inference.tensor_parallel import TPInferEngine
|
||||
from colossalai.logging import disable_existing_loggers
|
||||
from colossalai.shardformer import ShardConfig
|
||||
from colossalai.testing import clear_cache_before_run, parameterize, rerun_if_address_is_in_use, spawn
|
||||
|
||||
TP_SIZE = 2
|
||||
MAX_BATCH_SIZE = 4
|
||||
MAX_INPUT_LEN = 16
|
||||
MAX_OUTPUT_LEN = 8
|
||||
|
||||
CUDA_SUPPORT = version.parse(torch.version.cuda) > version.parse("11.5")
|
||||
|
||||
|
||||
@parameterize(
|
||||
"test_config",
|
||||
[
|
||||
{
|
||||
"tp_size": TP_SIZE,
|
||||
}
|
||||
],
|
||||
)
|
||||
def run(test_config):
|
||||
model_config = BloomConfig(num_hidden_layers=4, hidden_size=128, intermediate_size=256, num_attention_heads=4)
|
||||
model = BloomForCausalLM(model_config)
|
||||
model = model.half()
|
||||
model.to(torch.cuda.current_device())
|
||||
|
||||
# 1. check TPInferEngine init and model optimization
|
||||
shard_config = ShardConfig(
|
||||
enable_tensor_parallelism=True if test_config["tp_size"] > 1 else False, inference_only=True
|
||||
)
|
||||
infer_engine = TPInferEngine(model, shard_config, MAX_BATCH_SIZE, MAX_INPUT_LEN, MAX_OUTPUT_LEN)
|
||||
|
||||
assert infer_engine.cache_manager is not None
|
||||
assert infer_engine.tp_size == TP_SIZE
|
||||
assert infer_engine.head_num == model_config.num_attention_heads // TP_SIZE
|
||||
|
||||
# 2. check data preparation
|
||||
input_ids_list = [
|
||||
[80540, 15473, 3331, 11970, 90472, 361, 61335],
|
||||
[80540, 15473, 3331, 11970],
|
||||
[80540, 15473, 3331, 11970],
|
||||
[80540, 15473],
|
||||
]
|
||||
batch_size = len(input_ids_list)
|
||||
max_seq_len = max(len(li) for li in input_ids_list)
|
||||
attention_mask = [[0] * max_seq_len for _ in range(batch_size)]
|
||||
for i, li in enumerate(input_ids_list):
|
||||
attention_mask[i][max_seq_len - len(li) :] = [1 for _ in range(len(li))]
|
||||
data = dict(input_ids=input_ids_list, attention_mask=attention_mask)
|
||||
inputs_batch_encoding = BatchEncoding(data=data)
|
||||
seq_lengths = [len(li) for li in input_ids_list]
|
||||
start_loc = list(accumulate([0] + seq_lengths[:-1]))
|
||||
seq_lengths = torch.tensor(seq_lengths, dtype=torch.int32)
|
||||
start_loc = torch.tensor(start_loc, dtype=torch.int32)
|
||||
# input token id list as inputs
|
||||
batch_state_out1 = infer_engine.prepare_batch_state(inputs_batch_encoding)
|
||||
# BatchEncoding as inputs
|
||||
batch_state_out2 = infer_engine.prepare_batch_state(input_ids_list)
|
||||
|
||||
assert batch_state_out1.batch_size == batch_state_out2.batch_size == batch_size
|
||||
assert torch.equal(batch_state_out1.seq_len, batch_state_out2.seq_len)
|
||||
|
||||
# The following tests are discarded for now, and will be reused after all features are added
|
||||
# assert torch.equal(batch_state_out1.seq_len.to(seq_lengths.device), seq_lengths)
|
||||
# assert torch.equal(batch_state_out2.seq_len.to(seq_lengths.device), seq_lengths)
|
||||
# assert torch.equal(batch_state_out1.start_loc.to(start_loc.device), start_loc)
|
||||
# assert torch.equal(batch_state_out2.start_loc.to(start_loc.device), start_loc)
|
||||
|
||||
# 3. check optimized model generate
|
||||
input_ids = torch.randint(low=10, high=1000, size=(MAX_BATCH_SIZE, MAX_INPUT_LEN))
|
||||
generate_kwargs = dict(do_sample=False)
|
||||
infer_engine.generate(input_ids, **generate_kwargs)
|
||||
|
||||
torch.cuda.empty_cache()
|
||||
|
||||
|
||||
def check_engine(rank, world_size, port):
|
||||
disable_existing_loggers()
|
||||
colossalai.launch(config={}, rank=rank, world_size=world_size, host="localhost", port=port, backend="nccl")
|
||||
run()
|
||||
|
||||
|
||||
@pytest.mark.skipif(not CUDA_SUPPORT, reason="kv-cache manager engine requires cuda version to be higher than 11.5")
|
||||
@pytest.mark.dist
|
||||
@rerun_if_address_is_in_use()
|
||||
@clear_cache_before_run()
|
||||
def test_engine():
|
||||
spawn(check_engine, TP_SIZE)
|
||||
|
||||
|
||||
if __name__ == "__main__":
|
||||
test_engine()
|
|
@ -4,7 +4,7 @@ import pytest
|
|||
import torch
|
||||
from packaging import version
|
||||
|
||||
from colossalai.inference.tensor_parallel import MemoryManager
|
||||
from colossalai.inference.kvcache_manager import MemoryManager
|
||||
from colossalai.logging import disable_existing_loggers
|
||||
from colossalai.testing import rerun_if_address_is_in_use, spawn
|
||||
|
||||
|
|
|
@ -1,75 +0,0 @@
|
|||
import os
|
||||
|
||||
import pytest
|
||||
import torch
|
||||
from packaging import version
|
||||
from transformers import LlamaForCausalLM
|
||||
from transformers.models.llama.configuration_llama import LlamaConfig
|
||||
|
||||
import colossalai
|
||||
from colossalai.inference.tensor_parallel.engine import TPInferEngine
|
||||
from colossalai.logging import disable_existing_loggers
|
||||
from colossalai.shardformer import ShardConfig
|
||||
from colossalai.testing import clear_cache_before_run, parameterize, rerun_if_address_is_in_use, spawn
|
||||
|
||||
try:
|
||||
import lightllm
|
||||
HAS_LIGHTLLM_KERNEL = True
|
||||
except:
|
||||
HAS_LIGHTLLM_KERNEL = False
|
||||
|
||||
os.environ["TRANSFORMERS_NO_ADVISORY_WARNINGS"] = "true"
|
||||
TPSIZE = 2
|
||||
BATCH_SIZE = 8
|
||||
MAX_INPUT_LEN = 12
|
||||
MAX_OUTPUT_LEN = 100
|
||||
|
||||
CUDA_SUPPORT = version.parse(torch.version.cuda) > version.parse("11.5")
|
||||
|
||||
|
||||
@parameterize(
|
||||
"test_config",
|
||||
[
|
||||
{
|
||||
"tp_size": TPSIZE,
|
||||
}
|
||||
],
|
||||
)
|
||||
def run_llama_test(test_config):
|
||||
llama_config = LlamaConfig(
|
||||
num_hidden_layers=2, num_key_value_heads=8, bos_token_id=0, eos_token_id=1, vocab_size=1200, hidden_size=1024
|
||||
)
|
||||
model = LlamaForCausalLM(llama_config)
|
||||
model = model.half()
|
||||
|
||||
shard_config = ShardConfig(
|
||||
enable_tensor_parallelism=True if test_config["tp_size"] > 1 else False, inference_only=True
|
||||
)
|
||||
infer_engine = TPInferEngine(model, shard_config, BATCH_SIZE, MAX_INPUT_LEN, MAX_OUTPUT_LEN)
|
||||
generate_kwargs = dict(max_new_tokens=MAX_OUTPUT_LEN, do_sample=False)
|
||||
|
||||
input_tokens = {
|
||||
"input_ids": torch.randint(1, 1000, (BATCH_SIZE, MAX_INPUT_LEN), device="cuda"),
|
||||
"attention_mask": torch.ones((BATCH_SIZE, MAX_INPUT_LEN), device="cuda"),
|
||||
}
|
||||
outputs = infer_engine.generate(input_tokens, **generate_kwargs)
|
||||
|
||||
assert outputs is not None
|
||||
|
||||
|
||||
def check_llama(rank, world_size, port):
|
||||
disable_existing_loggers()
|
||||
colossalai.launch(config={}, rank=rank, world_size=world_size, host="localhost", port=port, backend="nccl")
|
||||
run_llama_test()
|
||||
|
||||
|
||||
@pytest.mark.skipif(not CUDA_SUPPORT or not HAS_LIGHTLLM_KERNEL, reason="kv-cache manager engine requires cuda version to be higher than 11.5")
|
||||
@pytest.mark.dist
|
||||
@rerun_if_address_is_in_use()
|
||||
@clear_cache_before_run()
|
||||
def test_llama():
|
||||
spawn(check_llama, TPSIZE)
|
||||
|
||||
|
||||
if __name__ == "__main__":
|
||||
test_llama()
|
|
@ -1,73 +0,0 @@
|
|||
import os
|
||||
|
||||
import pytest
|
||||
import torch
|
||||
from packaging import version
|
||||
from transformers import LlamaForCausalLM
|
||||
from transformers.models.llama.configuration_llama import LlamaConfig
|
||||
|
||||
import colossalai
|
||||
from colossalai.inference.tensor_parallel.engine import TPInferEngine
|
||||
from colossalai.logging import disable_existing_loggers
|
||||
from colossalai.shardformer import ShardConfig
|
||||
from colossalai.testing import clear_cache_before_run, parameterize, rerun_if_address_is_in_use, spawn
|
||||
|
||||
try:
|
||||
import lightllm
|
||||
HAS_LIGHTLLM_KERNEL = True
|
||||
except:
|
||||
HAS_LIGHTLLM_KERNEL = False
|
||||
|
||||
os.environ["TRANSFORMERS_NO_ADVISORY_WARNINGS"] = "true"
|
||||
TPSIZE = 2
|
||||
BATCH_SIZE = 8
|
||||
MAX_INPUT_LEN = 12
|
||||
MAX_OUTPUT_LEN = 100
|
||||
|
||||
CUDA_SUPPORT = version.parse(torch.version.cuda) > version.parse("11.5")
|
||||
|
||||
|
||||
@parameterize(
|
||||
"test_config",
|
||||
[
|
||||
{
|
||||
"tp_size": TPSIZE,
|
||||
}
|
||||
],
|
||||
)
|
||||
def run_llama_test(test_config):
|
||||
llama_config = LlamaConfig(num_hidden_layers=2, bos_token_id=0, eos_token_id=1, vocab_size=1200, hidden_size=1024)
|
||||
model = LlamaForCausalLM(llama_config)
|
||||
model = model.half()
|
||||
|
||||
shard_config = ShardConfig(
|
||||
enable_tensor_parallelism=True if test_config["tp_size"] > 1 else False, inference_only=True
|
||||
)
|
||||
infer_engine = TPInferEngine(model, shard_config, BATCH_SIZE, MAX_INPUT_LEN, MAX_OUTPUT_LEN)
|
||||
generate_kwargs = dict(max_new_tokens=MAX_OUTPUT_LEN, do_sample=False)
|
||||
|
||||
input_tokens = {
|
||||
"input_ids": torch.randint(1, 1000, (BATCH_SIZE, MAX_INPUT_LEN), device="cuda"),
|
||||
"attention_mask": torch.ones((BATCH_SIZE, MAX_INPUT_LEN), device="cuda"),
|
||||
}
|
||||
outputs = infer_engine.generate(input_tokens, **generate_kwargs)
|
||||
|
||||
assert outputs is not None
|
||||
|
||||
|
||||
def check_llama(rank, world_size, port):
|
||||
disable_existing_loggers()
|
||||
colossalai.launch(config={}, rank=rank, world_size=world_size, host="localhost", port=port, backend="nccl")
|
||||
run_llama_test()
|
||||
|
||||
|
||||
@pytest.mark.skipif(not CUDA_SUPPORT or not HAS_LIGHTLLM_KERNEL, reason="kv-cache manager engine requires cuda version to be higher than 11.5")
|
||||
@pytest.mark.dist
|
||||
@rerun_if_address_is_in_use()
|
||||
@clear_cache_before_run()
|
||||
def test_llama():
|
||||
spawn(check_llama, TPSIZE)
|
||||
|
||||
|
||||
if __name__ == "__main__":
|
||||
test_llama()
|
|
@ -4,11 +4,20 @@ from packaging import version
|
|||
|
||||
try:
|
||||
from colossalai.kernel.triton.token_attention_kernel import token_attention_fwd
|
||||
|
||||
HAS_TRITON = True
|
||||
except ImportError:
|
||||
HAS_TRITON = False
|
||||
print("please install triton from https://github.com/openai/triton")
|
||||
|
||||
|
||||
import importlib.util
|
||||
|
||||
HAS_LIGHTLLM_KERNEL = True
|
||||
|
||||
if importlib.util.find_spec("lightllm") is None:
|
||||
HAS_LIGHTLLM_KERNEL = False
|
||||
|
||||
TRITON_CUDA_SUPPORT = version.parse(torch.version.cuda) >= version.parse("11.6")
|
||||
|
||||
|
||||
|
@ -25,7 +34,8 @@ def torch_att(xq, xk, xv, bs, seqlen, num_head, head_dim):
|
|||
|
||||
|
||||
@pytest.mark.skipif(
|
||||
not TRITON_CUDA_SUPPORT or not HAS_TRITON, reason="triton requires cuda version to be higher than 11.4"
|
||||
not TRITON_CUDA_SUPPORT or not HAS_TRITON or not HAS_LIGHTLLM_KERNEL,
|
||||
reason="triton requires cuda version to be higher than 11.4 or not install lightllm",
|
||||
)
|
||||
def test():
|
||||
Z, head_num, seq_len, head_dim = 22, 112 // 8, 2048, 128
|
||||
|
|
Loading…
Reference in New Issue