mirror of https://github.com/hpcaitech/ColossalAI
[tutorial] polish all README (#1946)
parent
de56b563b9
commit
9183e0dec5
|
@ -18,22 +18,6 @@ quickly deploy large AI model training and inference, reducing large AI model tr
|
|||
[**Forum**](https://github.com/hpcaitech/ColossalAI/discussions) |
|
||||
[**Slack**](https://join.slack.com/t/colossalaiworkspace/shared_invite/zt-z7b26eeb-CBp7jouvu~r0~lcFzX832w)
|
||||
|
||||
|
||||
## Prerequisite
|
||||
|
||||
To run this example, you only need to have PyTorch and Colossal-AI installed. A sample script to download the dependencies is given below.
|
||||
|
||||
```
|
||||
# install torch 1.12 with CUDA 11.3
|
||||
# visit https://pytorch.org/get-started/locally/ to download other versions
|
||||
pip install torch==1.12.1+cu113 torchvision==0.13.1+cu113 torchaudio==0.12.1 --extra-index-url https://download.pytorch.org/whl/cu113
|
||||
|
||||
# install latest ColossalAI
|
||||
# visit https://colossalai.org/download to download corresponding version of Colossal-AI
|
||||
pip install colossalai==0.1.11+torch1.12cu11.3 -f https://release.colossalai.org
|
||||
```
|
||||
|
||||
|
||||
## Table of Content
|
||||
|
||||
- Multi-dimensional Parallelism
|
||||
|
@ -59,14 +43,6 @@ pip install colossalai==0.1.11+torch1.12cu11.3 -f https://release.colossalai.org
|
|||
- Stable Diffusion with Lightning
|
||||
- Try Lightning Colossal-AI strategy to optimize memory and accelerate speed
|
||||
|
||||
## Prepare Common Dataset
|
||||
|
||||
**This tutorial folder aims to let the user to quickly try out the training scripts**. One major task for deep learning is data preparataion. To save time on data preparation, we use `CIFAR10` for most tutorials and synthetic datasets if the dataset required is too large. To make the `CIFAR10` dataset shared across the different examples, it should be downloaded in tutorial root directory with the following command.
|
||||
|
||||
```python
|
||||
python download_cifar10.py
|
||||
```
|
||||
|
||||
|
||||
## Discussion
|
||||
|
||||
|
@ -74,3 +50,144 @@ Discussion about the [Colossal-AI](https://github.com/hpcaitech/ColossalAI) proj
|
|||
If you think there is a need to discuss anything, you may jump to our [Slack](https://join.slack.com/t/colossalaiworkspace/shared_invite/zt-z7b26eeb-CBp7jouvu~r0~lcFzX832w).
|
||||
|
||||
If you encounter any problem while running these tutorials, you may want to raise an [issue](https://github.com/hpcaitech/ColossalAI/issues/new/choose) in this repository.
|
||||
|
||||
## 🛠️ Setup environment
|
||||
You should use `conda` to create a virtual environment, we recommend **python 3.8**, e.g. `conda create -n colossal python=3.8`. This installation commands are for CUDA 11.3, if you have a different version of CUDA, please download PyTorch and Colossal-AI accordingly.
|
||||
|
||||
```
|
||||
# install torch
|
||||
# visit https://pytorch.org/get-started/locally/ to download other versions
|
||||
pip install torch==1.12.1+cu113 torchvision==0.13.1+cu113 torchaudio==0.12.1 --extra-index-url https://download.pytorch.org/whl/cu113
|
||||
|
||||
# install latest ColossalAI
|
||||
# visit https://colossalai.org/download to download corresponding version of Colossal-AI
|
||||
pip install colossalai==0.1.11rc3+torch1.12cu11.3 -f https://release.colossalai.org
|
||||
```
|
||||
|
||||
You can run `colossalai check -i` to verify if you have correctly set up your environment 🕹️.
|
||||

|
||||
|
||||
If you encounter messages like `please install with cuda_ext`, do let me know as it could be a problem of the distribution wheel. 😥
|
||||
|
||||
Then clone the Colossal-AI repository from GitHub.
|
||||
```bash
|
||||
git clone https://github.com/hpcaitech/ColossalAI.git
|
||||
cd ColossalAI/examples/tutorial
|
||||
```
|
||||
|
||||
## 🔥 Multi-dimensional Hybrid Parallel with Vision Transformer
|
||||
1. Go to **hybrid_parallel** folder in the **tutorial** directory.
|
||||
2. Install our model zoo.
|
||||
```bash
|
||||
pip install titans
|
||||
```
|
||||
3. Run with synthetic data which is of similar shape to CIFAR10 with the `-s` flag.
|
||||
```bash
|
||||
colossalai run --nproc_per_node 4 train.py --config config.py -s
|
||||
```
|
||||
|
||||
4. Modify the config file to play with different types of tensor parallelism, for example, change tensor parallel size to be 4 and mode to be 2d and run on 8 GPUs.
|
||||
|
||||
## ☀️ Sequence Parallel with BERT
|
||||
1. Go to the **sequence_parallel** folder in the **tutorial** directory.
|
||||
2. Run with the following command
|
||||
```bash
|
||||
export PYTHONPATH=$PWD
|
||||
colossalai run --nproc_per_node 4 train.py -s
|
||||
```
|
||||
3. The default config is sequence parallel size = 2, pipeline size = 1, let’s change pipeline size to be 2 and try it again.
|
||||
|
||||
## 📕 Large batch optimization with LARS and LAMB
|
||||
1. Go to the **large_batch_optimizer** folder in the **tutorial** directory.
|
||||
2. Run with synthetic data
|
||||
```bash
|
||||
colossalai run --nproc_per_node 4 train.py --config config.py -s
|
||||
```
|
||||
|
||||
## 😀 Auto-Parallel Tutorial
|
||||
1. Go to the **auto_parallel** folder in the **tutorial** directory.
|
||||
2. Install `pulp` and `coin-or-cbc` for the solver.
|
||||
```bash
|
||||
pip install pulp
|
||||
conda install -c conda-forge coin-or-cbc
|
||||
```
|
||||
2. Run the auto parallel resnet example with 4 GPUs with synthetic dataset.
|
||||
```bash
|
||||
colossalai run --nproc_per_node 4 auto_parallel_with_resnet.py -s
|
||||
```
|
||||
|
||||
You should expect to the log like this. This log shows the edge cost on the computation graph as well as the sharding strategy for an operation. For example, `layer1_0_conv1 S01R = S01R X RR` means that the first dimension (batch) of the input and output is sharded while the weight is not sharded (S means sharded, R means replicated), simply equivalent to data parallel training.
|
||||
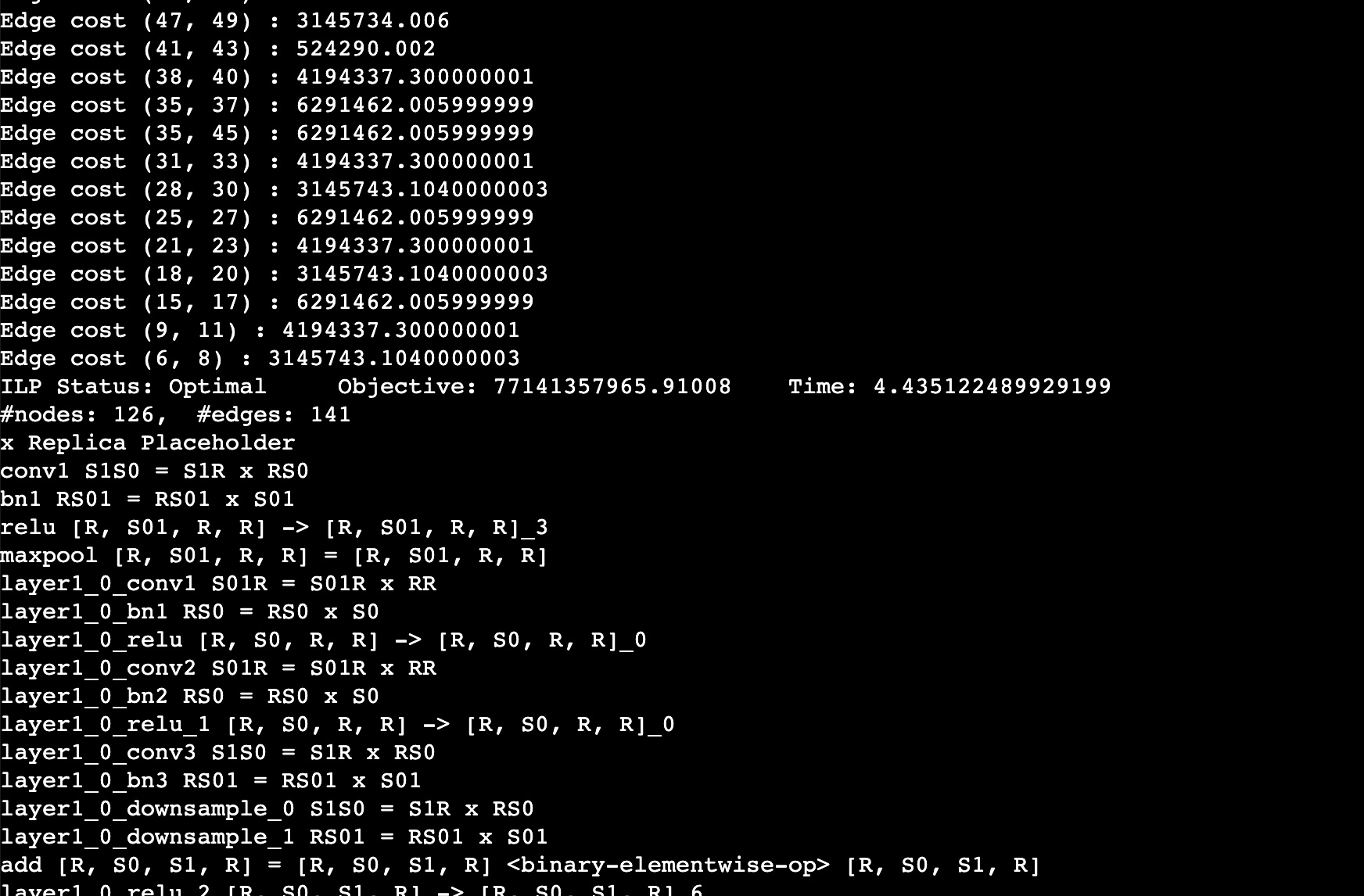
|
||||
|
||||
## 🎆 Auto-Checkpoint Tutorial
|
||||
1. Stay in the `auto_parallel` folder.
|
||||
2. Install the dependencies.
|
||||
```bash
|
||||
pip install matplotlib transformers
|
||||
```
|
||||
3. Run a simple resnet50 benchmark to automatically checkpoint the model.
|
||||
```bash
|
||||
python auto_ckpt_solver_test.py --model resnet50
|
||||
```
|
||||
|
||||
You should expect the log to be like this
|
||||
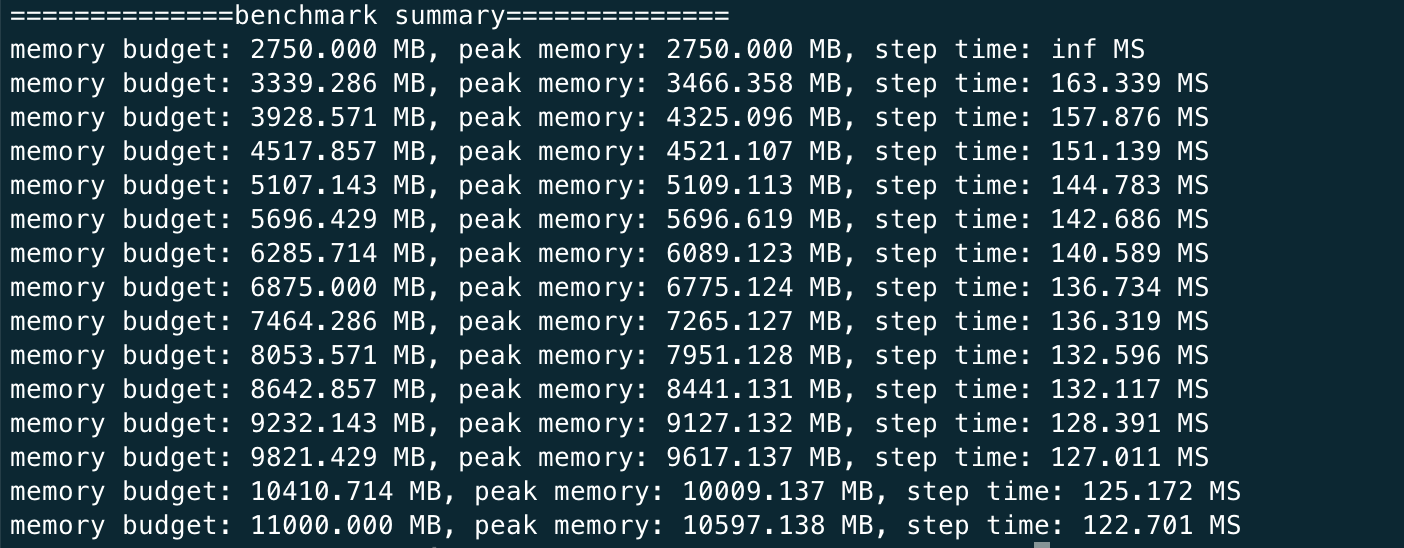
|
||||
|
||||
This shows that given different memory budgets, the model is automatically injected with activation checkpoint and its time taken per iteration. You can run this benchmark for GPT as well but it can much longer since the model is larger.
|
||||
```bash
|
||||
python auto_ckpt_solver_test.py --model gpt2
|
||||
```
|
||||
|
||||
4. Run a simple benchmark to find the optimal batch size for checkpointed model.
|
||||
```bash
|
||||
python auto_ckpt_batchsize_test.py
|
||||
```
|
||||
|
||||
You can expect the log to be like
|
||||

|
||||
|
||||
## 🚀 Run OPT finetuning and inference
|
||||
1. Install the dependency
|
||||
```bash
|
||||
pip install datasets accelerate
|
||||
```
|
||||
2. Run finetuning with synthetic datasets with one GPU
|
||||
```bash
|
||||
bash ./run_clm_synthetic.sh
|
||||
```
|
||||
3. Run finetuning with 4 GPUs
|
||||
```bash
|
||||
bash ./run_clm_synthetic.sh 16 0 125m 4
|
||||
```
|
||||
4. Run inference with OPT 125M
|
||||
```bash
|
||||
docker hpcaitech/tutorial:opt-inference
|
||||
docker run -it --rm --gpus all --ipc host -p 7070:7070 hpcaitech/tutorial:opt-inference
|
||||
```
|
||||
5. Start the http server inside the docker container with tensor parallel size 2
|
||||
```bash
|
||||
python opt_fastapi.py opt-125m --tp 2 --checkpoint /data/opt-125m
|
||||
```
|
||||
|
||||
## 🖼️ Accelerate Stable Diffusion with Colossal-AI
|
||||
1. Create a new environment for diffusion
|
||||
```bash
|
||||
conda env create -f environment.yaml
|
||||
conda activate ldm
|
||||
```
|
||||
2. Install Colossal-AI from our official page
|
||||
```bash
|
||||
pip install colossalai==0.1.10+torch1.11cu11.3 -f https://release.colossalai.org
|
||||
```
|
||||
3. Install PyTorch Lightning compatible commit
|
||||
```bash
|
||||
git clone https://github.com/Lightning-AI/lightning && cd lightning && git reset --hard b04a7aa
|
||||
pip install -r requirements.txt && pip install .
|
||||
cd ..
|
||||
```
|
||||
|
||||
4. Comment out the `from_pretrained` field in the `train_colossalai_cifar10.yaml`.
|
||||
5. Run training with CIFAR10.
|
||||
```bash
|
||||
python main.py -logdir /tmp -t true -postfix test -b configs/train_colossalai_cifar10.yaml
|
||||
```
|
||||
|
|
|
@ -1,5 +1,49 @@
|
|||
# Auto-Parallelism with ResNet
|
||||
|
||||
## 🚀Quick Start
|
||||
### Auto-Parallel Tutorial
|
||||
1. Install `pulp` and `coin-or-cbc` for the solver.
|
||||
```bash
|
||||
pip install pulp
|
||||
conda install -c conda-forge coin-or-cbc
|
||||
```
|
||||
2. Run the auto parallel resnet example with 4 GPUs with synthetic dataset.
|
||||
```bash
|
||||
colossalai run --nproc_per_node 4 auto_parallel_with_resnet.py -s
|
||||
```
|
||||
|
||||
You should expect to the log like this. This log shows the edge cost on the computation graph as well as the sharding strategy for an operation. For example, `layer1_0_conv1 S01R = S01R X RR` means that the first dimension (batch) of the input and output is sharded while the weight is not sharded (S means sharded, R means replicated), simply equivalent to data parallel training.
|
||||
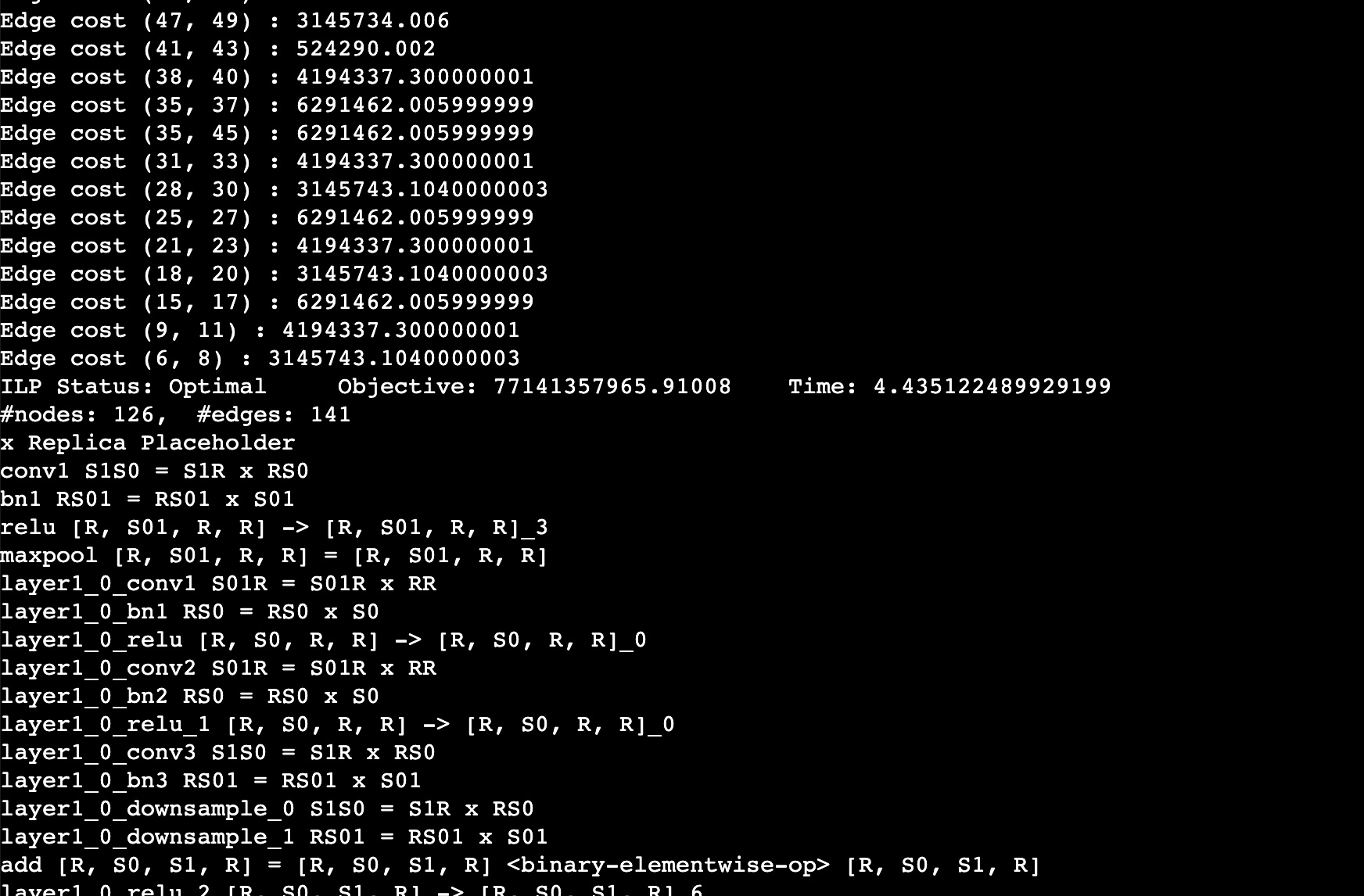
|
||||
|
||||
|
||||
### Auto-Checkpoint Tutorial
|
||||
1. Stay in the `auto_parallel` folder.
|
||||
2. Install the dependencies.
|
||||
```bash
|
||||
pip install matplotlib transformers
|
||||
```
|
||||
3. Run a simple resnet50 benchmark to automatically checkpoint the model.
|
||||
```bash
|
||||
python auto_ckpt_solver_test.py --model resnet50
|
||||
```
|
||||
|
||||
You should expect the log to be like this
|
||||
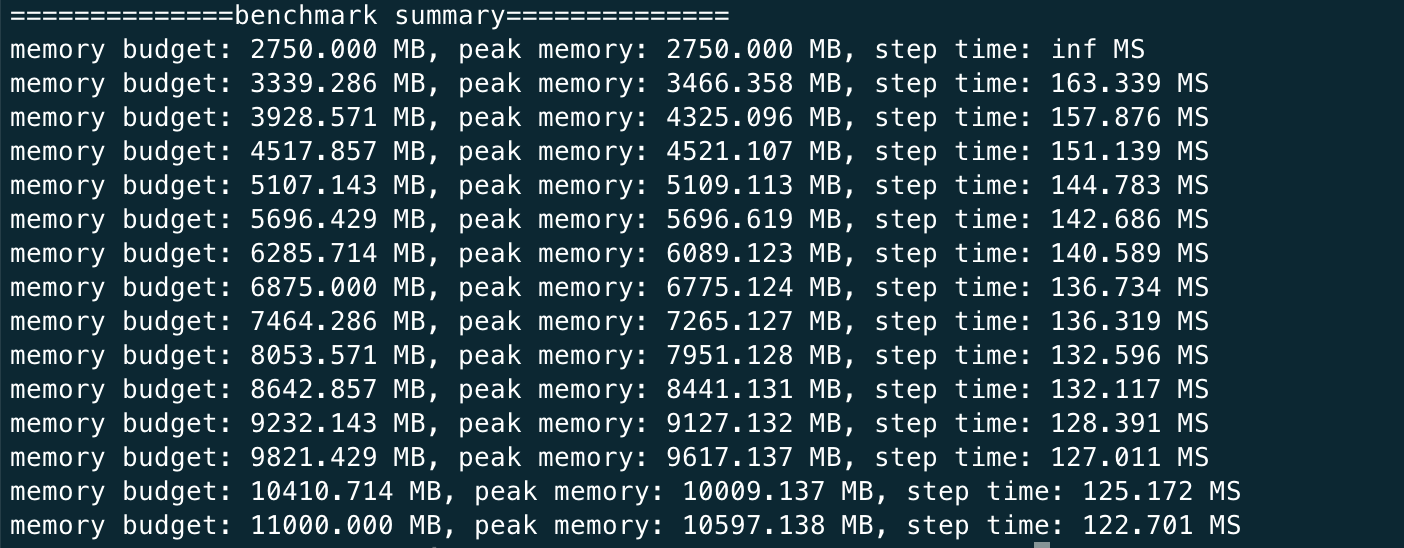
|
||||
|
||||
This shows that given different memory budgets, the model is automatically injected with activation checkpoint and its time taken per iteration. You can run this benchmark for GPT as well but it can much longer since the model is larger.
|
||||
```bash
|
||||
python auto_ckpt_solver_test.py --model gpt2
|
||||
```
|
||||
|
||||
4. Run a simple benchmark to find the optimal batch size for checkpointed model.
|
||||
```bash
|
||||
python auto_ckpt_batchsize_test.py
|
||||
```
|
||||
|
||||
You can expect the log to be like
|
||||

|
||||
|
||||
|
||||
## Prepare Dataset
|
||||
|
||||
We use CIFAR10 dataset in this example. You should invoke the `donwload_cifar10.py` in the tutorial root directory or directly run the `auto_parallel_with_resnet.py`.
|
||||
|
|
|
@ -1,6 +1,19 @@
|
|||
# Multi-dimensional Parallelism with Colossal-AI
|
||||
|
||||
|
||||
## 🚀Quick Start
|
||||
1. Install our model zoo.
|
||||
```bash
|
||||
pip install titans
|
||||
```
|
||||
2. Run with synthetic data which is of similar shape to CIFAR10 with the `-s` flag.
|
||||
```bash
|
||||
colossalai run --nproc_per_node 4 train.py --config config.py -s
|
||||
```
|
||||
|
||||
3. Modify the config file to play with different types of tensor parallelism, for example, change tensor parallel size to be 4 and mode to be 2d and run on 8 GPUs.
|
||||
|
||||
|
||||
## Install Titans Model Zoo
|
||||
|
||||
```bash
|
||||
|
|
|
@ -1,5 +1,12 @@
|
|||
# Comparison of Large Batch Training Optimization
|
||||
|
||||
## 🚀Quick Start
|
||||
Run with synthetic data
|
||||
```bash
|
||||
colossalai run --nproc_per_node 4 train.py --config config.py -s
|
||||
```
|
||||
|
||||
|
||||
## Prepare Dataset
|
||||
|
||||
We use CIFAR10 dataset in this example. You should invoke the `donwload_cifar10.py` in the tutorial root directory or directly run the `auto_parallel_with_resnet.py`.
|
||||
|
|
|
@ -4,6 +4,17 @@ This is an example showing how to run OPT generation. The OPT model is implement
|
|||
|
||||
It supports tensor parallelism, batching and caching.
|
||||
|
||||
## 🚀Quick Start
|
||||
1. Run inference with OPT 125M
|
||||
```bash
|
||||
docker hpcaitech/tutorial:opt-inference
|
||||
docker run -it --rm --gpus all --ipc host -p 7070:7070 hpcaitech/tutorial:opt-inference
|
||||
```
|
||||
2. Start the http server inside the docker container with tensor parallel size 2
|
||||
```bash
|
||||
python opt_fastapi.py opt-125m --tp 2 --checkpoint /data/opt-125m
|
||||
```
|
||||
|
||||
# How to run
|
||||
|
||||
Run OPT-125M:
|
||||
|
|
|
@ -15,6 +15,7 @@ limitations under the License.
|
|||
-->
|
||||
# Train OPT model with Colossal-AI
|
||||
|
||||
|
||||
## OPT
|
||||
Meta recently released [Open Pretrained Transformer (OPT)](https://github.com/facebookresearch/metaseq), a 175-Billion parameter AI language model, which stimulates AI programmers to perform various downstream tasks and application deployments.
|
||||
|
||||
|
@ -26,7 +27,21 @@ the tokenization). This training script is adapted from the [HuggingFace Languag
|
|||
## Our Modifications
|
||||
We adapt the OPT training code to ColossalAI by leveraging Gemini and ZeRO DDP.
|
||||
|
||||
## Quick Start
|
||||
## 🚀Quick Start for Tutorial
|
||||
1. Install the dependency
|
||||
```bash
|
||||
pip install datasets accelerate
|
||||
```
|
||||
2. Run finetuning with synthetic datasets with one GPU
|
||||
```bash
|
||||
bash ./run_clm_synthetic.sh
|
||||
```
|
||||
3. Run finetuning with 4 GPUs
|
||||
```bash
|
||||
bash ./run_clm_synthetic.sh 16 0 125m 4
|
||||
```
|
||||
|
||||
## Quick Start for Practical Use
|
||||
You can launch training by using the following bash script
|
||||
|
||||
```bash
|
||||
|
|
|
@ -5,6 +5,15 @@ activation along the sequence dimension. This method can achieve better memory e
|
|||
|
||||
Paper: [Sequence Parallelism: Long Sequence Training from System Perspective](https://arxiv.org/abs/2105.13120)
|
||||
|
||||
## 🚀Quick Start
|
||||
1. Run with the following command
|
||||
```bash
|
||||
export PYTHONPATH=$PWD
|
||||
colossalai run --nproc_per_node 4 train.py -s
|
||||
```
|
||||
2. The default config is sequence parallel size = 2, pipeline size = 1, let’s change pipeline size to be 2 and try it again.
|
||||
|
||||
|
||||
## How to Prepare WikiPedia Dataset
|
||||
|
||||
First, let's prepare the WikiPedia dataset from scratch. To generate a preprocessed dataset, we need four items:
|
||||
|
|
|
@ -5,6 +5,29 @@ fine-tuning for AIGC (AI-Generated Content) applications such as the model [stab
|
|||
We take advantage of [Colosssal-AI](https://github.com/hpcaitech/ColossalAI) to exploit multiple optimization strategies
|
||||
, e.g. data parallelism, tensor parallelism, mixed precision & ZeRO, to scale the training to multiple GPUs.
|
||||
|
||||
## 🚀Quick Start
|
||||
1. Create a new environment for diffusion
|
||||
```bash
|
||||
conda env create -f environment.yaml
|
||||
conda activate ldm
|
||||
```
|
||||
2. Install Colossal-AI from our official page
|
||||
```bash
|
||||
pip install colossalai==0.1.10+torch1.11cu11.3 -f https://release.colossalai.org
|
||||
```
|
||||
3. Install PyTorch Lightning compatible commit
|
||||
```bash
|
||||
git clone https://github.com/Lightning-AI/lightning && cd lightning && git reset --hard b04a7aa
|
||||
pip install -r requirements.txt && pip install .
|
||||
cd ..
|
||||
```
|
||||
|
||||
4. Comment out the `from_pretrained` field in the `train_colossalai_cifar10.yaml`.
|
||||
5. Run training with CIFAR10.
|
||||
```bash
|
||||
python main.py -logdir /tmp -t true -postfix test -b configs/train_colossalai_cifar10.yaml
|
||||
```
|
||||
|
||||
## Stable Diffusion
|
||||
[Stable Diffusion](https://huggingface.co/CompVis/stable-diffusion) is a latent text-to-image diffusion
|
||||
model.
|
||||
|
|
Loading…
Reference in New Issue